Create incredible AI portraits and headshots of yourself, your loved ones, dead relatives (or really anyone) in stunning 8K quality. (Get started for free)
7 Techniques to Enhance GPT Memory for Consistent AI Portrait Generation
7 Techniques to Enhance GPT Memory for Consistent AI Portrait Generation - Fine-tuning GPT models with portrait-specific datasets
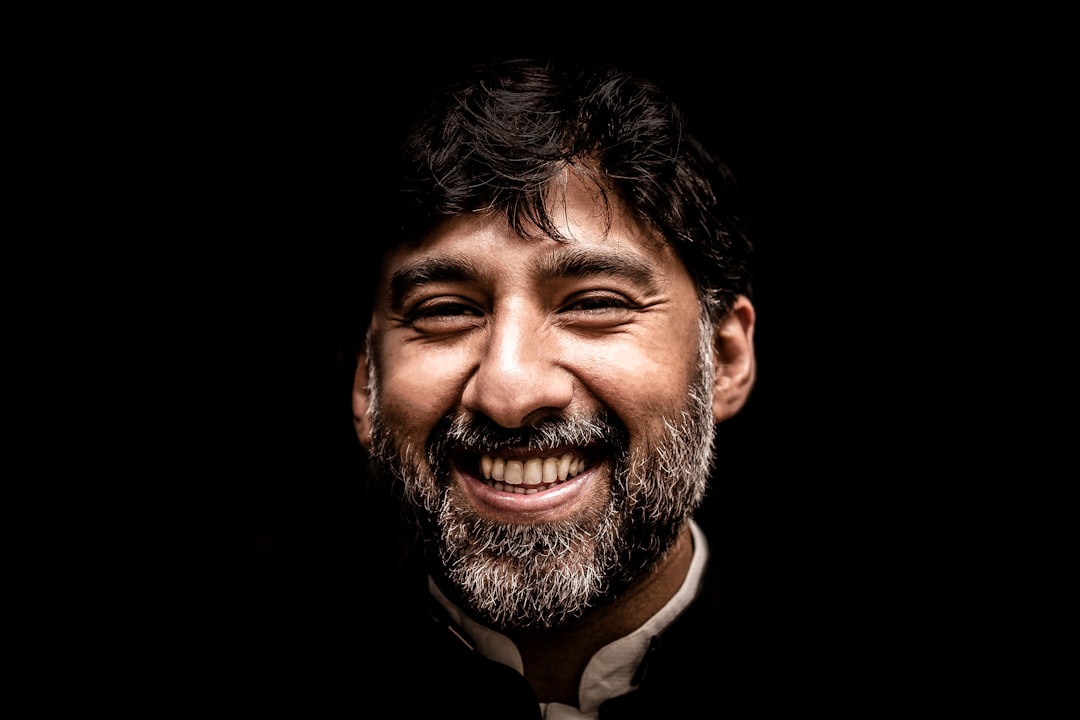
Tailoring GPT models for portrait generation involves fine-tuning them with datasets specifically designed for portraits. This approach aims to refine the AI's understanding of portrait styles, subjects, and desired characteristics. The benefits are clear: developers can achieve a higher degree of accuracy and consistency in the generated outputs. Some anecdotal evidence even suggests substantial gains in output correctness, highlighting the power of this technique. However, fine-tuning comes at a cost – training and utilizing the model involves token usage charges. But, the investment can lead to improved results, creating a trade-off between expenditure and refined outputs.
The adaptability of the fine-tuning process is a significant advantage. Developers can iteratively refine the model by incorporating user feedback and adjusting the dataset over time. This allows for a dynamic adaptation to the complexities of portrait photography, ultimately helping to ensure the AI's output better reflects the intended artistic vision. This flexibility enables a continuous evolution of the AI's capabilities, aligning more closely with the nuances of portraiture.
Adapting GPT models to generate compelling AI portraits involves fine-tuning them with specialized datasets. This approach capitalizes on the rich detail found in portrait photography, encompassing skin tones, lighting variations, and diverse backdrops to refine the model's understanding of human faces.
While the adage "more data is better" is often true, in portrait generation, it's not always the case. Datasets specifically focused on high-quality portraits often surpass larger, generic ones in effectiveness. This is due to their concentrated focus on the subtle nuances crucial to aesthetically pleasing portraiture. Even seemingly minor changes in the training data, such as the images' aspect ratios or portrait angles, can notably alter how a model interprets and renders human features. This highlights the importance of carefully curating these datasets.
The computational demands of fine-tuning with high-resolution imagery can be a significant hurdle. Processing and training require more resources compared to standard text-based model training. This factor needs careful consideration in a project's budget. In contrast to general datasets, portrait-specific training significantly reduces the probability of unwanted artifacts or distortions. This contributes to generating more precise portraits that match user expectations closely.
Training on niche portrait collections can contribute to mitigating biases in the generated outputs. It helps produce portraits that are more representative of diverse populations, reducing the risk of perpetuating stereotypical facial features.
While aiming for realism is desirable, finding the right balance between likeness and artistic interpretation remains a challenge. Fine-tuned models can occasionally overemphasize realism, resulting in portraits that feel overly artificial or lifelike, depending on user instructions. The choice of training data influences not just the aesthetic outcome but also the model's interpretation of facial features. Different cultures embed distinct nuances in photography, which can impact how a model interprets and defines these features.
Portrait datasets often incorporate metadata like age, gender, and emotional expression. This information can be leveraged during fine-tuning to generate portraits that align with specific user requests. However, the dynamic nature of portrait photography requires continuous dataset updates. Relying on static data risks generating images that quickly become outdated or fail to reflect evolving photographic styles and social norms. Staying current with trends and societal changes through dataset maintenance is therefore crucial for the continued relevance of these models.
7 Techniques to Enhance GPT Memory for Consistent AI Portrait Generation - Implementing memory-augmented neural networks
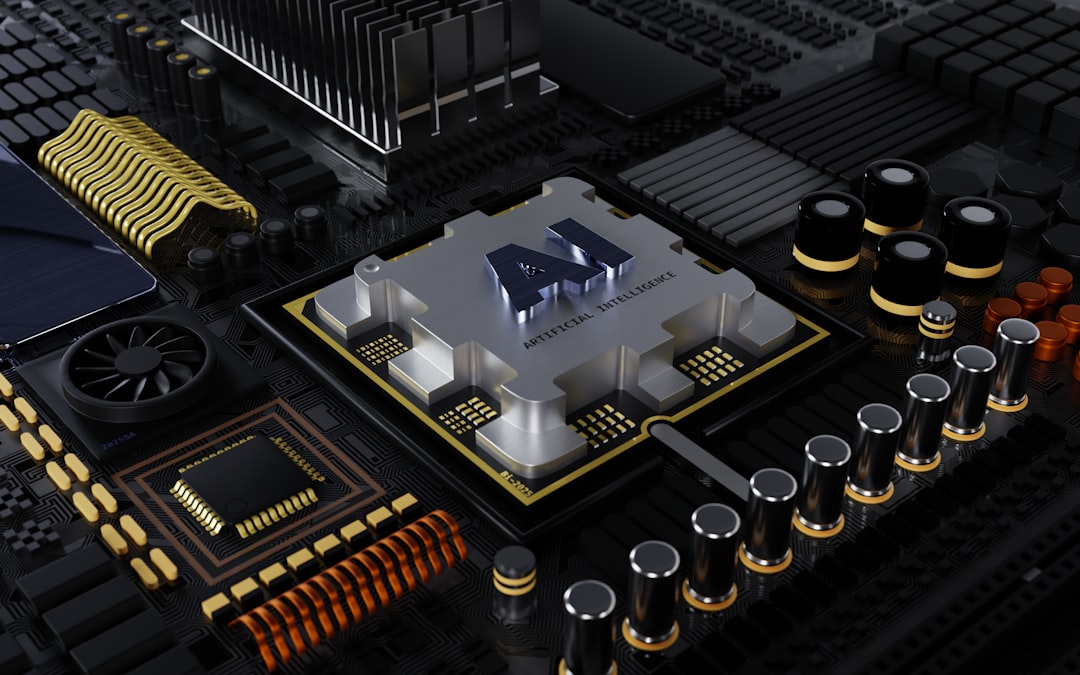
Integrating memory-augmented neural networks (MANNs) into AI systems presents a path towards enhancing memory for tasks like AI portrait generation. These networks incorporate external memory structures, allowing them to retain and access detailed information about individuals, a key requirement for crafting consistently high-quality AI headshots. This approach aims to improve a model's generalization capabilities, even with limited training data, and allow faster adaptation to user feedback and changing trends in portrait photography.
The implementation of MANNs, however, presents complexities. Designing efficient memory access mechanisms and managing the computational overhead of handling high-resolution images are major obstacles. Despite these challenges, the promise of MANNs lies in their potential to significantly improve the quality and consistency of AI-generated portraits. This includes generating outputs that align more closely with individual preferences and providing users with a more refined and personalized experience. The benefits seem promising, but the practical hurdles are non-trivial.
Memory-augmented neural networks (MANNs) are a fascinating approach that integrates external memory systems into the core of neural networks. Think of it like giving the AI a scratchpad or a personal journal to store and retrieve information, much like how our brains use memory to guide our decisions. This can potentially lead to more consistent AI portrait generation, especially when trying to capture diverse stylistic nuances.
The integration of MANNs can potentially accelerate the portrait generation process. By accessing stored representations, these networks could potentially deliver outputs much faster compared to traditional methods. This speed advantage could be particularly valuable in fields like professional photography, where rapid turnaround is essential.
Interestingly, MANNs, when properly trained, may be able to glean a deeper semantic understanding of the context behind a portrait. They may not just be replicating features but also grasping the core idea or feeling behind the portrait, resulting in outputs that are more contextually relevant.
A key advantage of MANNs over traditional neural networks is their adaptive capacity. Rather than needing significant retraining with every new stylistic change or photographic trend, they can supposedly adjust to new parameters more smoothly. This responsiveness could allow AI-powered portrait photography to rapidly adapt to shifting market demands.
One promising aspect of MANNs is the potential for increased resilience to information loss. They can potentially recall crucial details about stylistic preferences or individual client needs across multiple sessions. This consistent recall capability could be a game-changer for photographers striving for a cohesive visual identity across various projects for the same person.
The introduction of memory mechanisms can amplify interactive portrait creation. Imagine a scenario where users can give feedback in real-time, guiding the AI model toward the desired outcome through continuous adjustments. This type of feedback loop could greatly enhance the satisfaction level with the final portraits.
The path to integrating MANNs is not without its hurdles. The very complexity of these memory structures introduces a greater possibility of overfitting, meaning the model could become too specialized on the training data and fail to generalize to new scenarios. Finding the right balance between rich memory access and broad applicability is key to preventing overly rigid or repetitive portrait output.
Research suggests that MANNs can aid in the retention of stylistic details, potentially allowing AI systems to embrace trending portrait styles while catering to individual user preferences. This combination could ultimately boost client satisfaction and propel the use of AI-generated headshots in professional settings.
However, it's crucial to recognize that implementing MANNs can come at a cost. The extra layers and storage mechanisms they require can increase project budgets significantly. When evaluating MANNs, it's essential to consider whether the potential improvement in output quality justifies the higher cost.
Finally, it's noteworthy that MANNs could foster innovation in portrait creation. By blending various styles and aesthetics, they might push the boundaries of what's considered possible in AI-generated portraiture. This potential for creativity could result in the emergence of novel portrait genres, expanding the landscape of AI-generated photography.
7 Techniques to Enhance GPT Memory for Consistent AI Portrait Generation - Utilizing attention mechanisms for long-term consistency
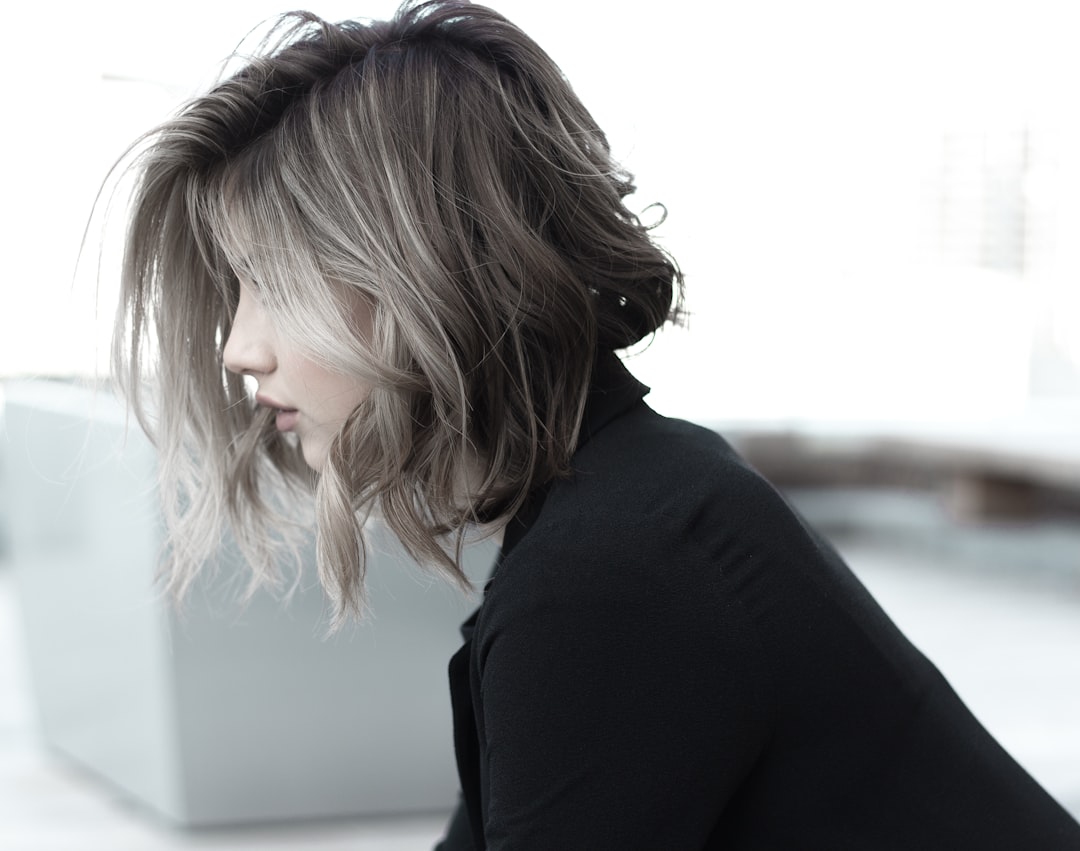
Integrating attention mechanisms into AI portrait generation presents a compelling way to achieve long-term consistency in the outputs. Attention mechanisms allow AI models to prioritize certain aspects of the input data, essentially focusing on important elements within a portrait. This enhanced focus gives the AI a better grasp of the visual relationships and context within the image. Through this deeper understanding, AI models can maintain consistency in their generated portraits over time. They can seamlessly adapt to shifts in photographic styles or trends while still adhering to the user's specific preferences for a portrait. This consistency fosters a smoother user experience and allows the AI-generated portraits to better reflect a photographer's distinct aesthetic vision. By leveraging attention mechanisms, we can potentially move towards a more refined and trustworthy approach to rendering AI-generated portraits, broadening the creative potential within portrait photography. However, it's important to remember that while the potential benefits are clear, practical implementation can be tricky and requires careful consideration of how the AI system processes and understands the complex aspects of human faces.
7 Techniques to Enhance GPT Memory for Consistent AI Portrait Generation - Utilizing Attention Mechanisms for Long-Term Consistency
Attention mechanisms are a crucial part of how GPT models work, allowing them to pick out relationships between different pieces of the input text and generate responses that fit the situation. This ability to zero in on specific parts is key for keeping the style and overall feel consistent in AI-generated portraits across multiple outputs.
In essence, attention mechanisms help GPT models keep track of the evolving trends in portrait styles. They learn from the changes and adjust, ensuring that AI-generated portraits remain relevant while still staying true to the user's previous preferences. It's like the model is paying attention to the changing landscape of portrait photography and adjusting accordingly.
One interesting side-effect of using attention mechanisms is they often lead to models that use less memory compared to older methods. This efficiency is good because it means the model can handle the complex world of portrait photography—with all its different resolutions and nuances—without needing too many computing resources. Potentially, this could result in faster processing and a reduction in the overall costs of running the model.
Beyond just keeping a consistent style, attention mechanisms help GPT models understand how everything in a portrait fits together. For example, they can pick up on the connection between facial expressions and the background elements, creating outputs that are not only visually harmonious but also tell a coherent story. It's a bit like how humans see things–the relationship between a person's facial expression and the background can tell us a lot about the mood or purpose of the portrait. Can an AI do this? That's the question we're trying to address.
Further, the way attention works naturally leads to a refinement process where the model constantly tweaks its outputs. This "iterative" approach can lead to portraits that get better and better with each iteration, improving satisfaction and reducing the likelihood of the weird glitches sometimes found in simpler AI models.
Finding the right balance between different styles and details is always tricky. But attention mechanisms offer a potential solution. The AI can weigh the importance of things like lighting, color, and texture, creating outputs that have a variety of looks but are still stylistically consistent.
Interestingly, attention might even help AI create portraits that trigger specific emotions in the user. This is achieved by focusing on elements traditionally linked to feeling, like warm colors or certain types of facial expressions. It is still early days in figuring out how to create art with AI but capturing the emotional essence of a portrait is an area of great interest.
Attention mechanisms can also help GPT models identify critical elements in the input data, such as a person's age, gender, or ethnicity. This enables more tailored portrait generation, ensuring that not only are the results accurate but also sensitive to the nuances of a user's requests. This opens up a world of possibilities and also introduces a lot of important questions around fairness, bias, and privacy.
Another strength of attention mechanisms is the way they can simplify the complexity of the portrait generation process. By adjusting their focus during training, the model can avoid over-specializing in just a few styles, and potentially create a more diverse range of portraits that are still coherent and consistent.
Finally, attention mechanisms make it easier for models to respond to user feedback, which in turn makes for a more satisfying, personalized experience. Users can essentially tweak a portrait in real-time as it's being generated, making sure it aligns perfectly with their wishes. This, of course, would be ideal but we have a long way to go before this becomes commonplace.
In short, attention mechanisms offer a promising path forward in developing AI models for generating portraits. They can help improve consistency, understand context, and adapt to user needs. However, the research is still young, and many challenges remain before we can unleash the full potential of this technology.
7 Techniques to Enhance GPT Memory for Consistent AI Portrait Generation - Applying transfer learning from high-quality portrait photography
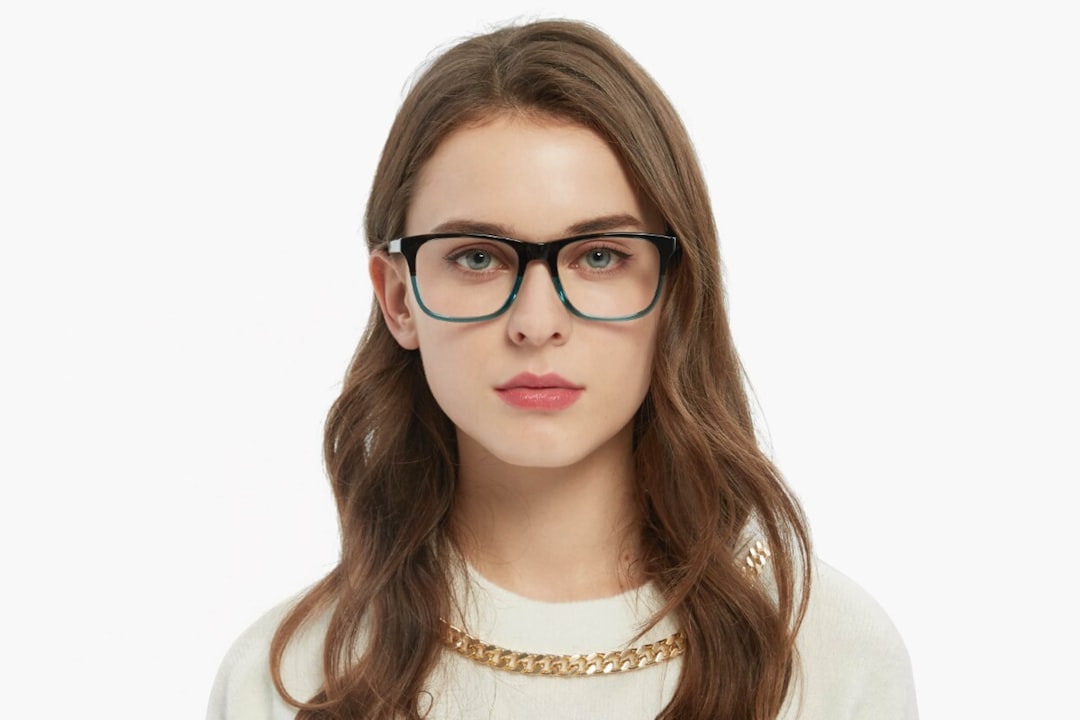
Applying transfer learning from high-quality portrait photography offers a promising avenue for enhancing AI-generated portraits. By training AI models on a diverse collection of expertly crafted portraits, we can teach them to understand the nuances of facial features, lighting, and stylistic elements. This allows the AI to generate portraits that retain a person's identity while still providing options for adjusting the aesthetic aspects, like the overall mood or artistic style.
This technique can be particularly valuable when AI models lack sufficient training data, effectively bridging the gap between limited resources and desired output quality. Transfer learning helps to improve the realism and artistry of the generated portraits, potentially leading to a more natural and appealing outcome.
While this approach is powerful, it's important to acknowledge that biases can exist within the initial portrait datasets. If these biases are not addressed, they can be transferred and amplified in the AI's generated outputs. Moreover, the styles and trends in portrait photography constantly evolve, demanding a constant influx of new, relevant data to keep the AI's results up-to-date. If datasets are not updated, the AI might generate portraits that feel outdated or miss the mark on current visual aesthetics. Continuously refreshing training data is key to ensuring relevance and maintaining the effectiveness of transfer learning in this domain.
Transfer learning can be a powerful tool for refining AI-generated portraits by leveraging knowledge from high-quality portrait photography. This approach essentially teaches the AI to map generated portraits to real ones, preserving facial identity while allowing for changes in style or appearance. It's like teaching an AI to paint like a master portrait artist by studying their work.
One interesting research direction explores the use of semantic, temporal, and lighting consistency in real-time video portrait relighting. Imagine adjusting the lighting on a video portrait in real-time, and the AI maintains the quality and authenticity of the face. While conceptually fascinating, the technical aspects of achieving such seamless integration are complex.
Another area of research involves generating line-based portrait sketches, like those you might find in a traditional art class, using generative adversarial networks (GANs). The process of training GANs to perform such a transformation could involve using a transfer learning framework, allowing them to draw upon the knowledge of how to generate realistic images.
The process of creating training data for AI portrait sketches can be enhanced by using a technique called style transfer. Here, researchers take a single artist's sketch and use style transfer models to generate a large dataset of variations. This dataset then helps train AI models to produce more creative and diverse sketch styles.
GANs have demonstrated their effectiveness in various image manipulation tasks, including portrait generation and editing, through the application of transfer learning. This speaks to the potential of GANs to not just create realistic images but to modify them in nuanced and artistic ways. However, achieving high-quality and consistent results in these areas can still be a challenge.
Tools are emerging that aim to assist amateur photographers in creating better portraits. One such model, called PhotoHelper, combines deep learning techniques with empirical aesthetic rules to provide feedback to users on portrait quality. It's like having an experienced photographer guide you on framing, lighting, and composition, but powered by AI. Such tools offer a tantalizing vision of AI-driven improvement in photography.
The rapid advancement of AI, including techniques like neural style transfer and multimodal models, is revolutionizing mobile photography. This allows for the creation of AI-generated content, which could range from stylistic filters to the complete creation of photographs. The democratization of photo editing is a fascinating development.
Studies have shown that using examples of high-resolution portrait styles during transfer learning can boost the quality of AI-generated portraits, even with limited data. This suggests that a few well-chosen examples are sometimes better than a vast pool of general imagery.
A specific architecture, called DualStyleGAN, allows for finer control over both intrinsic and extrinsic portrait styles. This model can essentially separate the elements of a portrait (like lighting and facial structure) and allows independent control over them. This opens doors to advanced artistic control over generated portraits.
Combining a multi-tasking architecture with lighting disentanglement leads to improvements in portrait generation and relighting models. This means that AI can perform multiple tasks simultaneously, like adjusting the lighting and generating a portrait, leading to more efficient and advanced models.
The path to truly authentic AI-generated portraits is an active area of research. The techniques listed here hint at the exciting developments being explored to bring better, more realistic, and artistically capable AI portrait generation to fruition. While challenges persist, the field is continuously advancing.
7 Techniques to Enhance GPT Memory for Consistent AI Portrait Generation - Leveraging meta-learning for adaptive portrait generation
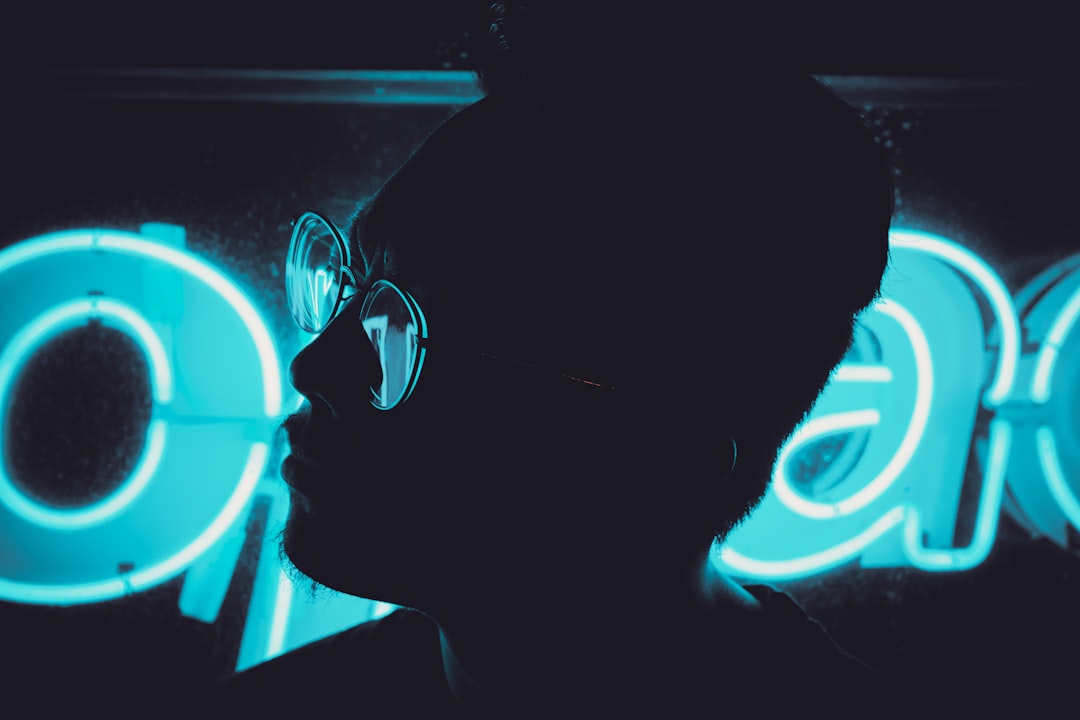
Meta-learning offers a promising avenue for creating more adaptable and personalized AI-generated portraits. By training models on a vast dataset, spanning over 14,000 iterations, meta-learning enables models to swiftly adapt to different portrait styles and individual preferences. This approach not only improves the accuracy of capturing facial details and emotions but also helps maintain consistency in stylistic choices across multiple portrait generations. Techniques like generative priors are often incorporated to boost the details and enhance the visual quality of the generated portraits.
However, the sophistication of this approach introduces challenges in handling computational demands and preventing overfitting, which is where a model becomes too specialized on the training data and struggles with new scenarios. Careful algorithm design is crucial to ensure the model generalizes well and produces diverse, relevant portraits. In essence, meta-learning could revolutionize how we create AI-generated portraits, but its effectiveness depends on continued research and improvements in model design and training. The long-term viability of this technique depends on ongoing refinements to ensure outputs remain consistent and reflect the evolving trends and expectations within portrait photography.
In the pursuit of crafting AI-generated portraits that feel authentic and artistically compelling, meta-learning presents itself as a promising avenue. One particular aspect of this involves the model's ability to learn from a wide array of portrait examples, understanding not just the technical elements but also the nuanced styles and trends in portrait photography. We find that in AI portrait generation, quality trumps sheer quantity when it comes to training datasets. Highly curated, specific datasets focused on portraiture yield better results than larger, more general datasets. It's a bit like saying the right kind of practice, even if a bit less, can be more effective than just endless repetitions.
Furthermore, the incorporation of memory-augmented neural networks holds significant potential for customizing outputs based on previous interactions. Think of this as giving the AI a sort of memory to learn from previous sessions with the user. This capability can help maintain consistency across various sessions and allow users to progressively refine their preferred artistic style, be it a particular lighting preference or a focus on certain facial features. This dynamic tailoring ensures that the outputs reflect the user's unique preferences better.
The ability for these AI models to dynamically adapt to trends within the world of portrait photography is a powerful advantage. Essentially, the models incorporate feedback mechanisms, letting them tweak and adjust without extensive retraining every time a new photographic style becomes popular. This makes for a more responsive and relevant outcome, keeping the AI's outputs fresh and in tune with the latest artistic preferences, all while still adhering to a user's established preferences.
Attention mechanisms come into play to help the AI prioritize and understand the relationships within the input image, such as how facial expressions interact with the surrounding elements in a portrait. It's like giving the AI a way to see the bigger picture, beyond just individual features. This heightened understanding can lead to outputs that are not only visually appealing but also convey a deeper narrative or message, making the AI portrait more engaging and meaningful.
Interestingly, research suggests that AI models can learn to evoke particular emotions through lighting and color manipulations. This hints at a potential for AI to not only mimic reality but also to evoke a specific emotional response. The AI might be able to understand the connection between warm lighting and a feeling of comfort, or how a dramatic shadow can emphasize a certain emotion. It's early days still, but it's fascinating to ponder how AI might be able to go beyond simple replication and start to evoke the kind of emotional depth we expect from art.
Leveraging transfer learning from collections of high-quality portrait photography provides a powerful method for improving AI portrait generation, especially when the AI has limited training data to work with. This transfer of existing knowledge allows for an improvement in the generated outputs, making them more realistic and aesthetically pleasing. It's analogous to a student learning by studying the work of a master artist. However, it's crucial to remain mindful of any inherent biases in the original datasets to avoid amplifying those tendencies within the generated outputs.
The inclusion of memory mechanisms in these AI models brings another benefit: resilience to information loss. AI systems can maintain stylistic preferences and remember past user interactions. This persistence allows for smoother and more collaborative generation processes, aiding in the development of a consistent artistic persona across multiple projects.
While training with high-resolution images is beneficial for achieving visually richer results, it comes with a cost. The computational resources required for training with such imagery can be substantial, leading to concerns about budget constraints within AI projects. Striking a balance between output quality and resource allocation is a constant consideration.
Through careful selection and curation of training datasets, AI can minimize biases in the generated outputs. By ensuring the training data reflects a wider variety of individuals, AI can generate portraits that are more representative and avoid inadvertently perpetuating any stereotypes.
Finally, the advent of advanced AI techniques, like Generative Adversarial Networks (GANs), has opened doors to a wealth of creative possibilities within AI portrait generation. By learning from a diverse range of artistic styles, the AI has the potential to not only mimic but also innovate, giving birth to entirely new portrait styles and furthering the frontiers of AI's artistic potential in photography.
While there are ongoing challenges and areas for refinement, leveraging these meta-learning techniques suggests a promising future for AI in portrait generation, offering the potential to create more authentic, artistic, and personalized portraits.
7 Techniques to Enhance GPT Memory for Consistent AI Portrait Generation - Integrating multi-modal inputs to enhance portrait context
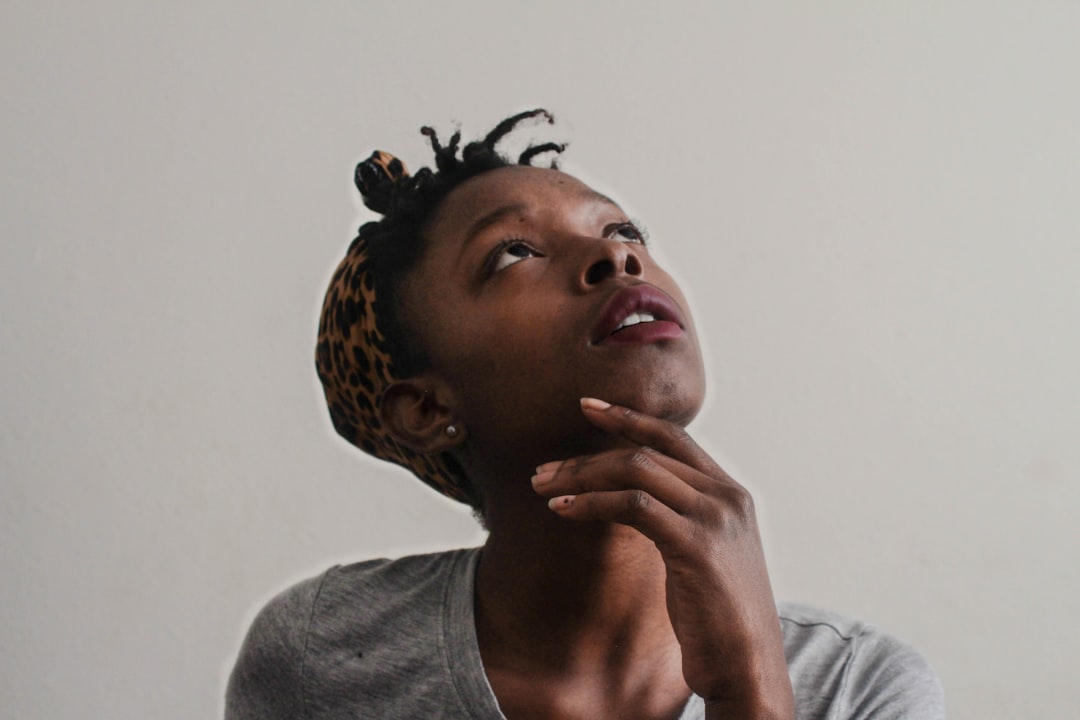
The concept of "Integrating multi-modal inputs to enhance portrait context" introduces a new dimension to AI portrait generation. It aims to improve AI's understanding of portraits by feeding it multiple types of information, such as images, spatial details, and specific facial features. Think of it as providing the AI with a more complete picture, much like how humans use various senses to perceive the world.
This approach employs techniques like multimodal pose detection, where the AI not only analyzes the image but also factors in spatial clues like the person's position and body language. Other methods, like Contrastive Chain-of-Thought (CoCoT) prompting, help the AI dig deeper into the visual details within a portrait. The ultimate goal is to create a richer understanding of the context surrounding the portrait.
Currently, many AI models rely mainly on single-resolution images, which can be limiting in understanding details and capturing the complete essence of a portrait. This multi-modal approach moves beyond this limitation, mimicking the human brain's ability to combine various types of data. The hope is that by understanding the context more thoroughly, the AI can produce portraits that are not only visually accurate but also capture the deeper nuances and feelings associated with portrait photography. While still a developing area, this multi-modal approach holds potential for transforming the field of AI portrait generation.
Incorporating multiple types of input, like images combined with text descriptions, empowers AI systems to develop a richer understanding of the person within a portrait. This enriched context helps the AI to produce facial expressions and emotions with more accuracy, potentially leading to portraits that not only look realistic but also convey a deeper story.
This idea is supported by cognitive load theory, which proposes that simultaneously receiving different forms of information helps with learning and memory. In the AI context, it allows models to build a more comprehensive and contextually aware representation of people in portraits.
Diverse generative techniques, like adversarial networks in conjunction with other generative models, can leverage multi-modal input to produce complex details in portraits. This enhances the texture of AI-generated headshots, bringing them closer to the appearance of traditional portraits. It replicates the subtle interplay of light and shadows that makes human faces appear so realistic.
Multi-modal datasets have the capacity to minimize bias in AI-generated portraits. By including a variety of inputs that represent different cultures, ages, and genders, AI can be trained to create more inclusive and representative portraits. This expands the aesthetic range and accuracy of outputs.
Utilizing multi-modal learning can optimize computational resources by minimizing the need for massive datasets. AI models can achieve better performance with fewer data points by cleverly integrating textual and visual inputs, potentially lowering the cost of producing high-quality portraits.
Furthermore, multi-modal integration allows AI models to adapt in real-time to user feedback. As users make comments or changes during the portrait generation process, the AI can incorporate the information instantly. This dynamic adjustment offers a more personalized and satisfying user experience.
Multi-modal approaches can enrich the emotional impact of AI-generated portraits. By analyzing the relationship between textual cues (like mood descriptors) and visual components, the AI can create portraits that stimulate specific emotions or themes, utilizing psychological principles that affect how people respond to images.
AI systems show enhanced adaptive learning abilities when using multi-modal inputs. They can rapidly recalibrate their understanding of portrait aesthetics based on shifting trends and cultural influences within photography. This ensures that outputs remain relevant and reduces the risk of portraits appearing outdated.
Multi-modal systems are better suited for high-resolution image training, managing both computational requirements and memory efficiently. This lets AI portraits achieve finer details and textures that enhance their visual quality, while mitigating the issue of overfitting commonly found in simpler models that use a single input type.
Ultimately, using multiple types of inputs positions AI portrait generation not just as a technological pursuit, but also as a potential business advantage. Photographers and content creators can leverage this technology to swiftly react to client preferences and industry changes, impacting the cost and demand for AI-generated images in professional settings.
While promising, there is still much research needed in this field to push the boundaries of AI's capabilities. The evolution of this technology will continue to reshape the field of portrait photography and the broader landscape of image generation.
7 Techniques to Enhance GPT Memory for Consistent AI Portrait Generation - Employing iterative refinement techniques for improved accuracy
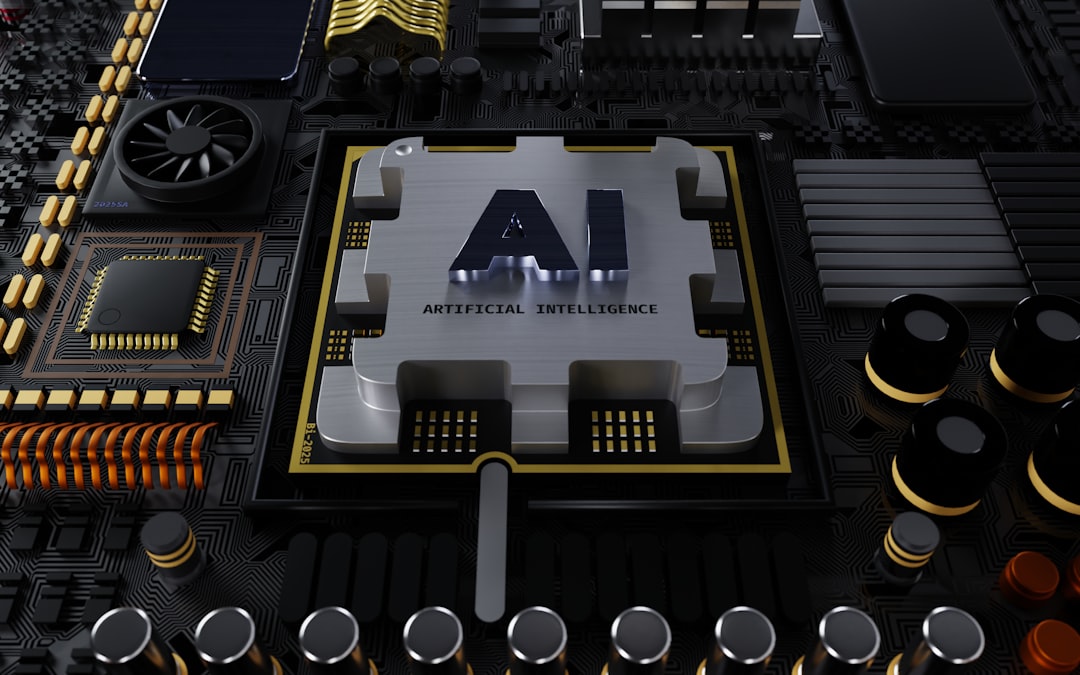
Using iterative refinement techniques to improve accuracy in AI-generated portraits can significantly boost the quality and consistency of the output. This method involves continually adjusting the AI model based on feedback and new data, leading to more refined results with each iteration. This systematic approach helps address inaccuracies that can arise, especially when capturing the fine details that are crucial in portrait photography. While this can introduce computational challenges, particularly in maintaining the necessary precision for detail-rich portraits while also keeping operations efficient, the benefits of increased accuracy suggest that the effort is worthwhile. In the end, iterative refinement can better bridge the gap between AI outputs and the photographer's artistic vision, contributing to more realistic and aesthetically pleasing portraits created by artificial intelligence.
1. **Gradual Improvements through Iterations:** Iterative refinement, a technique borrowed from fields like mathematics and data science, offers a way for AI models to learn and improve progressively. Each portrait generated provides feedback to the model, subtly adjusting its understanding of what constitutes a good headshot. This continuous refinement allows the AI to get better at creating accurate and visually appealing portraits without needing a massive overhaul of its training data.
2. **Managing Costs Effectively:** Training AI models from scratch can be expensive, especially when dealing with high-resolution portrait imagery. Iterative refinement offers a more efficient path, as we're not throwing away previous work and starting over. It allows us to focus on fine-tuning specific areas of portrait creation, like enhancing skin tones or adjusting expressions, without a complete retraining. It's a matter of strategically allocating resources.
3. **Breaking Down Complex Problems:** Training AI to generate portraits involves a wide range of factors–lighting, expression, composition, etc. Iterative refinement allows us to break these complexities down into smaller, more manageable steps. Instead of trying to teach the AI everything at once, we can focus on refining one aspect at a time. This targeted approach leads to better outcomes as the AI can delve deeper into the details that truly define a successful portrait.
4. **Adapting in Real Time:** One of the exciting aspects of iterative refinement is its ability to incorporate user feedback. Imagine a scenario where you give the AI model feedback on a generated portrait, and it uses that information to adjust its output in subsequent attempts. This dynamic learning process ensures the AI remains responsive not only to specific preferences but also to the ever-changing trends in portrait photography. The results should be more consistent and updated portraits.
5. **Addressing Biases:** The issue of bias in AI models is something researchers are acutely aware of. Iterative refinement offers a path toward reducing this bias. As the AI iteratively generates portraits, it can identify patterns that might favor certain features or styles over others. It can then adapt to minimize or remove these biases, leading to more inclusive and equitable outcomes in AI-generated portraits. This, however, could be complex to implement in the real world and requires more research.
6. **Focus on Detailed Features:** Portraiture is about capturing the fine details of a person's face–the soft curve of a smile, the sparkle in their eyes, or the delicate play of light and shadow. Iterative refinement allows the AI model to focus on these small, yet critical, elements. Each iteration hones the model's ability to depict finer details, creating a gradual build-up of nuanced features that mimic the complexities of a high-quality photograph.
7. **Meeting Individual Needs:** Users will have different ideas about what a good portrait looks like. Iterative refinement helps to create more personalized results. If a user dislikes a specific element of a generated portrait, that feedback can be incorporated into the next iteration, leading to a more accurate representation of the user's vision. It's a more collaborative approach to image creation.
8. **Incremental Gains Can Be Substantial:** It's sometimes tempting to think that larger datasets and massive computational resources will automatically yield better results in AI training. But, research suggests that focused refinement using incremental changes can lead to significant improvements in performance. A few small, well-chosen adjustments to training data can sometimes outperform broad, general approaches.
9. **Capturing Emotional Nuances:** Portrait photography is not just about capturing a person's appearance but also about their emotions. Iterative refinement can be used to fine-tune the emotional tone of a portrait. Maybe it's a matter of subtly adjusting lighting to create a warmer, more inviting feel or changing features to convey a feeling of joy or sadness. It's an open question to what extent AI can capture or inspire emotions with a portrait.
10. **Smarter Resource Usage:** The computational power needed for training AI can be expensive, especially with high-resolution images. Iterative refinement allows us to strategically manage these resources. Instead of putting all our effort into one giant training run, we can refine the model incrementally. This approach optimizes computational use, making the AI-portrait generation process more cost-effective in the long run. This area will need to be watched closely as AI model training becomes more demanding.
Create incredible AI portraits and headshots of yourself, your loved ones, dead relatives (or really anyone) in stunning 8K quality. (Get started for free)
More Posts from kahma.io: