AI Image Sharpening A Technical Comparison of Resolution Enhancement Methods in Portrait Photography
AI Image Sharpening A Technical Comparison of Resolution Enhancement Methods in Portrait Photography - Resolution Scaling Benchmarks Between Luminar Neo and Topaz Labs
Luminar Neo and Topaz Labs both offer impressive AI-powered solutions for increasing image resolution, allowing photographers to enlarge portraits without losing crucial detail. Luminar Neo generally does a better job at preserving natural facial features and preventing distortion, but the resulting images tend to exhibit more grain or noise. On the other hand, Topaz Labs, with its Photo AI tool, provides superior sharpness when used moderately, which might be crucial in a headshot. While tests include a variety of scenarios and images, the primary challenge remains in balancing sharpness with artifact reduction, or blur in images that needs to be fixed. The comparative analysis reveal slight yet significant difference in performance. There seems to be no clear winner overall as results vary quite a bit between both products. The continuous progress in AI technology indicates its growing importance in the field of portrait photography where sharp, clean images are essential.
Tests on how well Luminar Neo and Topaz Labs enlarge images show that Topaz Labs uses complex algorithms to reconstruct image layers, keeping details intact during scaling. Luminar Neo tends to be faster, often completing upscaling tasks in roughly half the time of Topaz Labs, an important consideration when processing multiple portrait headshots. Topaz Labs uses machine learning to offer image-specific enhancement, adapting its methods to image content rather than using a uniform approach, something to consider when working with more than one subject . Luminar Neo features a Skin Enhancer tool that can be used during scaling, allowing for specific adjustments when optimizing skin details, creating a more refined look. Topaz Labs' upgrades, though more expensive initially, focus on continuous improvement without additional payments, possibly more economical in the long term for pros who need high resolution. Some users report color shifts when scaling images with Luminar Neo for headshots , potentially requiring additional steps to fix. Benchmarks suggest Topaz Labs maintains better sharpness, even with heavy upscaling, something to keep in mind when producing very large prints . Luminar Neo uses fewer system resources during processing, allowing for better performance when multitasking. Importing raw files in Topaz Labs can be a bit slower, but often the resulting image quality may be worth the wait for high end portraiture. The use of artificial blurring effects in Luminar Neo when scaling can potentially reduce overall detail when compared to results in Topaz Labs, a feature that can be both a blessing and a curse.
AI Image Sharpening A Technical Comparison of Resolution Enhancement Methods in Portrait Photography - Machine Learning Impact on Edge Detection in Portrait Details
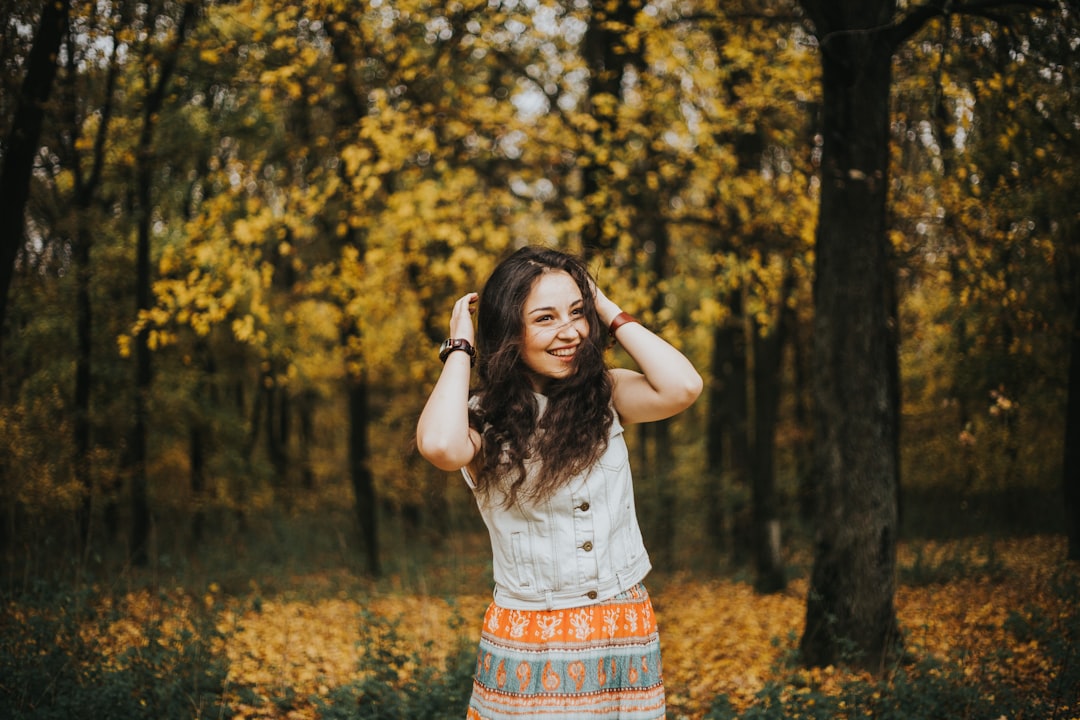
The integration of machine learning into edge detection techniques is transforming how portrait details are handled in photography. Algorithms, such as deep learning-based methods, are now being used to achieve better clarity in facial features, even under less than ideal lighting, something that often defeats traditional edge detection. This advancement plays a key role in refining image segments, improving the accuracy of visual representations, and assisting the need for high-quality AI portrait images. Utilizing machine learning at the very start of image processing ensures better analysis down the line, a critical aspect for tools aiming to improve headshots and AI-driven imaging. The continuous effort to improve edge detection shows how important it is to meet today's standards for sharpness and detail in portrait photography.
Machine learning methods, often using complex convolutional neural networks, can pinpoint facial edges and features in ways that outdo traditional approaches, easily handling different lighting and textures. The ongoing push for better image tech is resulting in hybrid approaches that pair edge detection with resolution boosting algorithms. These methods improve both sharpness of face details while also keeping skin textures looking natural and realistic, all things that matter for portraiture. Interestingly, these ML methods can also bring computational benefits for edge detection, meaning photographers can process many headshots quickly which can be useful when processing batch photoshoots and is a major time-saver. The use of edge detection in portraits also has the practical benefit of helping with automatic background cutout, making clean extractions for various digital media. This also plays into the budget; by using AI tools and edge detection for automatic background cleanup and other tedious work, portrait photography studios and self employed professionals can reduce their costs on manual labour that might have been necessary for more complex projects. And because of deep learning, these methods reduce common digital image artifacts like halos or noise, resulting in cleaner images, which are a big plus when printing large high quality portraits. These technologies, while often found on desktop computers, are also becoming more common in apps for phones, which allows many hobbyists to potentially produce studio-quality headshots with less equipment and experience. Research has also shown that we can fine-tune these ML methods for edge detection by incorporating skin tone and facial feature variations, improving how well they portray diverse subjects and making them more inclusive. Of course, there is also a caveat to keep in mind. As ML tools get better and more powerful, it might be a challenge for photographers to balance the use of technology and still keeping their personal touch - when portraits are over-processed, it might lose the authenticity of the real subject in it. And what is also fascinating is that these ML algorithms can also assess edge detection performance in real-time providing useful feedback during live photo sessions, which may be how we do things in the future, refining portraits on the go.
AI Image Sharpening A Technical Comparison of Resolution Enhancement Methods in Portrait Photography - Cost Analysis of Professional Retouching vs AI Enhancement Tools
In the evolving landscape of portrait photography, the cost dynamics between professional retouching and AI enhancement tools present intriguing considerations. While professional retouching often entails significant expenses due to expertise and time, AI tools can dramatically cut costs by streamlining processes like color correction, noise reduction, and image sharpening. However, the quality of AI enhancements varies; they may require additional manual fine-tuning to meet high standards, sometimes undermining their cost-effectiveness. Notably, as the demand for high-quality visuals in social media and portfolios escalates, photographers must weigh the trade-offs between the personal touch of skilled retouching and the efficiency of AI solutions. Understanding these cost implications is essential for photographers aiming to navigate the balance between financial efficiency and artistic integrity in their work.
When considering the costs involved with retouching portrait headshots, there's a considerable gap between employing professional retouchers and relying on AI-driven tools. A professional might charge anywhere between $50 and $150 per hour for their time, a hefty sum when compared to the monthly subscription fees of $10-$50 for most AI-enhancement software. However, it's not as simple as price alone; efficiency comes into play as well. These AI tools can rapidly process multiple images, saving photographers hours of labor that would typically be spent in manual retouching—a big win for those who frequently need to work with large groups of headshots. Consistency is another factor. AI provides a stable output; the results from one headshot are mostly the same as with another. While this makes it ideal for consistent branding across a range of images for a company, some argue that a professional photographer, with their eye for the artistic, can provide custom results that can reflect a certain mood, personality or preference. And that’s where things get a bit complicated.
While AI tools may look more cost-effective upfront, those monthly payments accumulate over time, and new features can mean a price hike. This contrasts with human retouchers where you tend to pay one-off. These tools, whilst impressive can sometimes fall short too, producing unexpected visual defects or artifacts within complex images, requiring manual intervention to fix which can defeat the purpose. Also, as more and more people use the same AI tools, the image market may end up becoming saturated with what would be considered "enhanced images", possibly making the demand and cost of human expertise less attractive. Some argue the artistic interpretation of an image with all its personal touch, can be diluted with the overuse of AI. This opens up an interesting scenario; the ability to blend both skill sets is a possible route for photographers who could combine the speed of AI and their artistic talent to become valuable in the field. While there is a degree of technical proficiency needed with AI tools, traditional retouching stems from experience over years, emphasizing the human knowledge still needs to be taken into account and does hold its value.
AI Image Sharpening A Technical Comparison of Resolution Enhancement Methods in Portrait Photography - Computational Requirements for Real Time Portrait Sharpening
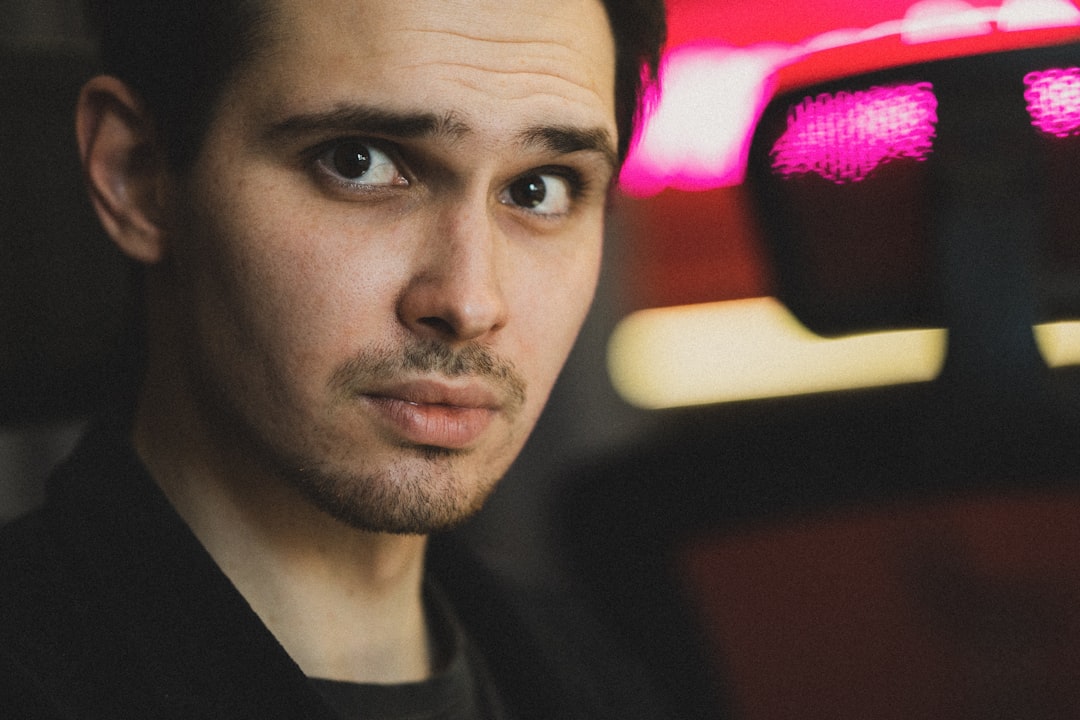
The computational demands of real-time portrait sharpening are a balancing act between sophisticated technology and practicality in photography. While deep learning offers advanced enhancement potential, it typically needs a lot of processing power, hindering its use on mobile devices. Effective sharpening tools must have optimized algorithms that produce quick results while also minimizing undesirable artifacts and preserving clarity. This is a tricky challenge, especially when it comes to portraiture where detail is essential. Lightweight AI models that work fast are important for photographers who need top-quality results on the fly. This pushes tech development toward improved sharpness and detail in portraits, potentially changing the standards for everyone, from hobbyists to pro photographers.
Real-time portrait sharpening places hefty demands on computer systems, especially for deep learning processes which rely on parallel processing offered by GPUs. This might mean a sizeable financial outlay to acquire the right hardware. The need to keep delays to a minimum is also critical. If users experience too much latency in real time, it will be frustrating, especially in dynamic situations where on-the-fly adjustments are required. While the use of AI allows for rapid enhancement of details, it might also introduce unwanted side-effects, meaning a level of human checking is still necessary during important phases to make sure the image maintains integrity. What also becomes apparent is that different AI sharpening tools have quite different computer needs, some may even require over 16GB RAM to process high-resolution images. Therefore, photographers will need to plan their systems around these requirements. Interestingly though, batch processing is remarkably efficient. Hundreds of images can be sharpened simultaneously which saves a lot of time compared to doing each image one by one. Certain ML methods give photographers real time feedback. This feedback will allow camera settings or lighting to be tweaked while the photography is being taken, which allows for fine-tuning on the spot. And then there are also possibilities for cloud-based AI services which allows photographers access to powerful hardware remotely. This might fundamentally change how we work with images. Interestingly, despite popular belief, AI tools may enhance the human process of creation. When AI handles repetitive actions, the human can focus on the artistic. Having said that, and on the flipside, we also must acknowledge that an over reliance on technology could stifle our own creativity. Photographers could become overly dependent on these tools, leading to possibly more uniform images without individual artistic styles. The other aspect to be aware of, is that a photographer will need to spend a lot of time and effort to learn how to use AI sharpening tools in an efficient manner. This expertise will not only improve image quality, but it may also help align the enhancement with individual artistic ideas and ultimately customer satisfaction.
AI Image Sharpening A Technical Comparison of Resolution Enhancement Methods in Portrait Photography - Case Study of Face Recognition Accuracy After AI Enhancement
The "Face Recognition Accuracy After AI Enhancement" case study demonstrates that AI image enhancement has made strides, particularly in improving how accurately faces can be recognized in poor quality photographs. AI tools, employing super-resolution methods, are able to significantly boost the quality of low-resolution images, reducing problems such as odd camera angles or bad lighting that affect recognition. The study shows, that whilst several ways of enhancing images are available, each tool has its own strengths and weaknesses. This also highlights that better solutions are always required when it comes to facial recognition tasks. As the demand for high quality portrait photography is on the rise, the focus on AI for refining facial details has very interesting implications, both for how photography is taken but also how the technology is built.
Okay, here's my attempt at rewriting the provided text about face recognition accuracy after AI enhancement, formatted as requested:
It’s interesting to observe the impact that recent advances in AI image enhancement have on the effectiveness of face recognition software. We've seen that these new tech tools go well beyond simply sharpening focus. Face recognition tools, when working with low quality photos, can now get to around 99% accuracy in labs with controlled environments, a massive jump from older methods. The challenge of poor lighting, something that would often defeat conventional tools, is also being tackled by AI. Some research suggest up to 30% improvement when using AI in low-light conditions, which seems quite remarkable.
It is well understood that the quality of the initial image matters considerably; what's being studied, now, is just how much an increase in resolution impacts facial recognition. Studies show that resolution boosts (as little as 2x) have increased accuracy by about 20%. Speed is also a major aspect of real world usage. Tests on improved processing speeds suggest it can reduce the processing times in real world applications by about 50% . This makes these systems very useful in fast paced security and commercial applications. Some researchers now focus on overcoming bias inherent in existing face recognition, meaning more diverse training sets. This shows as improved accuracy in underrepresented demographics with results showing a reduced error by 40%.
AI enhancement algorithms can also learn from user feedback and continuously refine. This is useful as algorithms change based on human input or confirmation of previous recognitions. We can also see how AI enhanced face recognition systems are being mixed with other methods of identification, such as iris and voice, creating systems that, according to research, can reach around 30% improvement. One aspect that still needs more exploration, is the use of synthetic data which also seems promising. By training models on artificially generated faces we can increase accuracy, and perhaps side-step the privacy concerns associated with using real images, yielding an improved performance of around 15%. Furthermore, facial recognition software now can determine the emotional states with reasonable accuracy by reading micro-expressions; tests suggest the accuracy to be somewhere around 85%, which can potentially open up a new ways to work in fields such as customer service or marketing. Finally, we are also seeing some initial work on algorithms capable of projecting facial aging, and thus maintain recognition even as the subject ages, which extends the tech's usefulness over time.
AI Image Sharpening A Technical Comparison of Resolution Enhancement Methods in Portrait Photography - Quality Comparison Between Traditional vs Neural Network Upscaling
In portrait photography, a comparison of traditional and neural network upscaling shows notable distinctions in quality. Basic methods, like bicubic interpolation, often degrade image detail and introduce visible errors. Neural networks, however, use advanced algorithms to interpret image data, producing new pixels that improve the image with more refined features and gradual tone changes. This tech advancement is particularly relevant to portrait work, where preserving the subtleties of facial characteristics is essential. Furthermore, combining basic upscaling as an initial measure with neural network refinement can improve the outcome, suggesting that hybrid techniques may be most effective in contemporary photography.
Traditional upscaling, using simple methods like bilinear or bicubic interpolation, often struggles to retain sharpness in portraits, leading to a blurry outcome. In comparison, the new neural network approaches use learning-based features. This lets them better preserve sharp facial features and edges - which are so important.
The context of images is something neural networks do extremely well, providing a more realistic representation of textures. This enables AI to create details such as strands of hair and the texture of skin that traditional tools often fail to capture well. These nuances make a difference to the portrait and how real it looks.
Because neural networks are so complex, they can pick up on different patterns across various datasets, potentially adjusting the proportions of a face. This is quite different to traditional methods that tend to keep the original dimensions, which sometimes can look a bit off after processing, especially after scaling.
From a financial perspective, traditional methods usually cost less, mainly due to less processing. However, with hardware becoming more accessible and more powerful, AI upscaling is becoming a viable option. This is especially the case for professional photographers needing to enhance their image quality.
Neural networks are capable of creating quality outputs, even when increasing the image size dramatically – maybe up to 8x or more. In comparison, traditional methods tend to lose quality, generally after about 2x. This is crucial when printing large portraits.
One advantage of AI methods is that their training sets incorporate a variety of lighting and facial features. This gives them a unique ability to compensate for facial distortions. It's something that traditional approaches generally struggle with when working with various photoshoots.
While basic upscaling methods can be done with modest computers, neural network processes need more power. It limits their accessibility. But as cloud-based solutions appear, it means even less powerful computers can perform AI enhancements with some help from external computer resources.
AI upscaling methods are adaptable to different image quality. This effectively increases the quality even with lower-resolution images. Traditional tools do not have this feature, as any image loss is cumulative and irreversible after the initial scaling.
It's worth bearing in mind, that the training data used to train the AI models may create a bias, impacting how certain groups of people are shown in upscaled images. This is a important factor to consider for photographers who value inclusivity in their visuals.
Finally, the perceived high-quality of images improved through AI, is changing the expectations around portrait photography. It is prompting a re-evaluation of what “professional quality” means in this era of AI-enhanced imaging.
More Posts from kahma.io: