Create incredible AI portraits and headshots of yourself, your loved ones, dead relatives (or really anyone) in stunning 8K quality. (Get started for free)
How AI-Powered Tools Transform Regular Photos into Striking Silhouettes A Technical Analysis
How AI-Powered Tools Transform Regular Photos into Striking Silhouettes A Technical Analysis - Neural Network Edge Detection Reshapes Raw Photography into Clean Lines
Artificial intelligence is transforming the way we process photographs, particularly through the use of neural networks for edge detection. These networks, especially deep convolutional neural networks, are adept at extracting the fine details within an image, focusing on gradients and subtle changes in brightness that define edges and shapes. This allows for improved object recognition and analysis of form within a photograph.
The field has seen the development of more efficient neural network designs, such as low-complexity residual networks. These networks manage to achieve high accuracy in detecting edges while requiring fewer computational resources. This is particularly important for applications where real-time processing is crucial, such as live image manipulation or editing.
Another interesting direction is the development of neural network architectures that draw inspiration from the human visual system. This bio-inspired approach aims to create more sophisticated edge detection, mimicking the way our brains interpret visual information and integrate edge cues. This could lead to a more nuanced and natural look in processed images, offering a higher degree of artistic control to photographers and image editors.
These advancements suggest that neural networks are poised to redefine photography. We can anticipate improved clarity, definition, and a greater capacity to extract and manipulate the essence of an image. The intersection of computational power and creative vision, through AI-driven edge detection, could transform traditional photography into a much more dynamic and expressive medium.
Neural networks have revolutionized how we perceive and manipulate edges in photographs. These networks, trained on extensive image datasets, can identify and highlight the contours of objects with a precision that surpasses older, gradient-based methods like Sobel or Canny. This ability to delineate objects against complex backgrounds stems from the network's capacity to learn intricate patterns and adapt to diverse image conditions. Traditional methods often struggle with low-contrast or noisy images, leading to inaccurate or incomplete edge detection. However, neural networks demonstrate better robustness in such challenging situations, improving the overall quality and usefulness of the resulting edges.
The sophistication of neural networks extends beyond basic edge detection. They can perceive edges at multiple scales and orientations, allowing for more nuanced extraction of features that aid in guiding the viewer's focus. Additionally, the integration of color segmentation with edge detection opens up possibilities for more intricate image manipulations, such as generating refined silhouettes that maintain a nuanced understanding of both image structure and hue.
Interestingly, this enhanced edge perception allows neural networks to go beyond simple object isolation. They can identify subtle anomalies within the image, potentially aiding in the automatic removal of watermarks or distractions. This capability streamlines the post-processing workflow, reducing the need for manual intervention and enabling photographers to achieve a cleaner, more focused result. Furthermore, the potential for real-time applications is becoming more evident as neural networks are integrated into camera systems, giving photographers the ability to observe edge-enhanced previews in real-time before capturing an image.
However, it's important to acknowledge the computational cost associated with some of these sophisticated neural network implementations. While edge detection is a powerful tool, future research will likely focus on developing methods that achieve similar accuracy with lower computational requirements, especially for use in devices with limited processing power. Nevertheless, the potential of neural networks to reshape the way we edit and perceive photographs remains exciting, promising a future where even seemingly mundane images can be transformed into striking visual expressions.
How AI-Powered Tools Transform Regular Photos into Striking Silhouettes A Technical Analysis - Converting Color Depth Maps into Minimalist Black Forms using Machine Learning
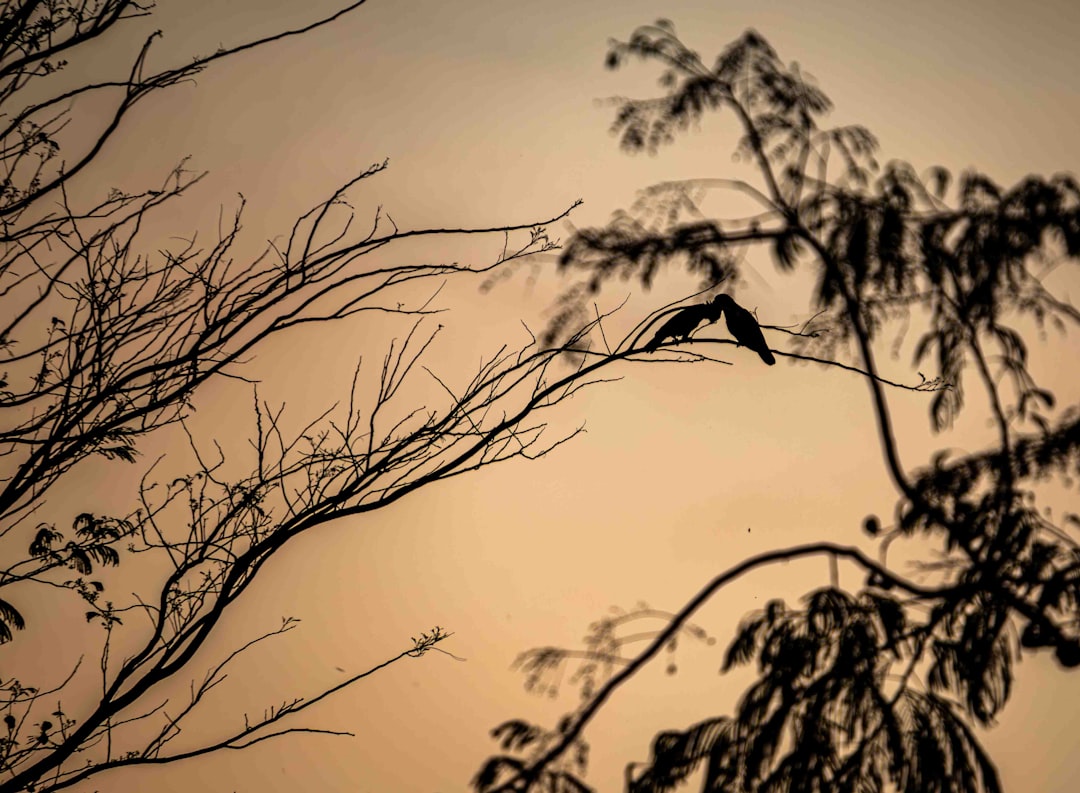
The ability to convert color depth maps into minimalist black forms using machine learning offers a novel approach to image manipulation and artistic expression. Depth maps, essentially grayscale representations where brightness corresponds to distance from the viewer, serve as the foundation for this transformation. AI algorithms are then employed to interpret these maps, enabling the creation of strong, visually compelling silhouettes that preserve the original image's spatial relationships. This process bridges the gap between technological capabilities and artistic intent, facilitating the crafting of visually arresting works that prioritize shape and structure over intricate details.
While the integration of AI into photography creates intriguing possibilities, it also prompts reflection on the authenticity and future direction of visual expression. The capacity to manipulate depth data so effectively allows for a new level of control over image composition, but it can also blur the line between original photography and AI-generated art. It's important to consider how this new technology might reshape creative practices, potentially pushing artistic boundaries while prompting us to reconsider our notions of photographic representation. The ongoing evolution of AI will undoubtedly play a pivotal role in determining the future of image creation, and the methods presented here signify a significant advancement in this journey.
Depth maps, essentially grayscale images where brightness corresponds to distance from the camera, are a valuable resource in photography. However, they can be further processed to create even more compelling visuals, specifically minimalist black forms. This transformation involves using machine learning models that interpret the depth information in a novel way. These models learn to distinguish objects within the scene based on their depth, effectively segmenting the image into foreground and background elements.
The power of AI in this process lies in its ability to handle diverse lighting conditions and complex backgrounds, which can be challenging for traditional photo editing methods. It can discern subtle differences in depth to intelligently prioritize certain elements, thus influencing the composition of the silhouette. Think of it as having the AI 'understand' the spatial hierarchy of an image, choosing what to emphasize and what to fade into the background.
The potential applications are wide-ranging. Creating visually compelling logos or promotional materials is one area where these simplified forms can shine. The minimalist aesthetic inherently removes distractions and draws attention to core elements, often enhancing brand identity.
Additionally, these techniques can also significantly speed up the editing process. By implementing machine learning models that allow real-time previewing of silhouette transformations, photographers can experiment and refine their edits quickly. The computational methods at play often involve clever dimensionality reduction techniques—a way of prioritizing the most salient features while discarding less important information. This ensures the resulting silhouette is impactful without excessive detail.
An interesting side effect of this process is the potential for accessibility. The simplification of an image into a black form can provide a different means of interaction with photographs, especially for individuals with visual impairments. Such minimalist representations could be used in tactile art, providing a new way to experience and understand photographs.
Beyond the practical benefits, there's also the fascinating interplay of AI and photography theory. Understanding how Fourier transforms or other mathematical techniques underpin these silhouette transformations offers deeper insight into image structure and recognition. In a world of increasingly sophisticated image processing, this knowledge can elevate a photographer's abilities, pushing the boundaries of creative expression through AI-powered tools. While the computational requirements for these advanced algorithms can be demanding, the potential for enhancing image creation and accessibility, particularly in photography, is undeniable and presents exciting avenues for exploration.
How AI-Powered Tools Transform Regular Photos into Striking Silhouettes A Technical Analysis - Automated Light Source Analysis Creates Natural Shadow Perspectives
AI-powered tools are increasingly capable of analyzing light sources within a photograph, leading to the creation of remarkably realistic shadows. This automated analysis provides a level of control previously unavailable to photographers, allowing them to manipulate the interplay of light and shadow with precision. The result is the ability to transform ordinary images into compelling silhouettes with a depth and dimensionality that mimics how light behaves in the real world.
This capacity to analyze and refine light interactions significantly benefits photographers, streamlining the creative process and fostering new forms of artistic expression. The ability to seamlessly integrate natural-looking shadows within an image unlocks a greater level of control over the visual narrative. However, it's important to consider that while AI can enhance shadows, achieving perfectly natural-looking results in complex scenarios can remain challenging. It will be interesting to observe how this technology develops and influences the future of photography, particularly regarding the creation of visually appealing silhouettes. We might see a shift in how photographers think about composition and light as they gain access to powerful new tools that enable them to manipulate these elements more creatively and with greater control. The ongoing evolution of AI and its integration into image editing software is sure to have a significant impact on how we perceive and interact with photographic images.
AI-powered tools are increasingly capable of analyzing light sources within an image and using that information to generate realistic-looking shadows. This is achieved by employing algorithms that model how light interacts with three-dimensional shapes, essentially creating a digital simulation of how a light source would cast a shadow on a particular object. The ability to produce natural-looking shadows enhances the overall depth and dimensionality of silhouettes, making them appear more integrated within the scene rather than simply being a cut-out.
Expanding on this, AI systems can analyze the entire dynamic range of the image, which is the difference between the brightest and darkest parts. This helps them refine the contrast between the silhouette and its background, resulting in a more impactful and clearly defined silhouette. Further, these tools are starting to model the surrounding environment when determining how light impacts the objects in a scene. This context-aware approach yields shadows that are more consistent with the lighting conditions present in the photograph, further improving the realism of the generated silhouettes.
However, this is not just about static shadows. Some systems are developing the ability to analyze how shadows shift over time, which opens exciting possibilities for generating dynamic, motion-based effects for photography and even for generating elements for motion pictures or other sequential media. Another area where these tools are improving is their ability to differentiate between the key objects within a scene and those that are simply background. This selective focus improves the quality of the silhouettes, minimizing distractions that could make the image appear cluttered or confusing.
Many of these tools use complex layering of different algorithms in order to get the most realistic effects. This can involve multiple stages of analysis and fine-tuning to ensure that the shadows harmonize with the overall aesthetic of the photograph. This algorithmic layering also improves upon the ability to learn the various ways that light sources interact with objects. The algorithms are trained on enormous datasets of photos, allowing them to learn patterns and techniques that lead to more nuanced, contextually appropriate shadow generation. This approach also can tailor shadows to match the style or look that the photographer desires.
Further, these automated tools are often designed to be flexible, allowing for parameters like shadow softness or the direction of the light to be adjusted manually. This level of control offers photographers a great deal of creative flexibility to achieve the exact desired outcome. However, challenges remain. The task of accurately reconstructing shadows in situations with unusual lighting conditions or in images that have a very high contrast between light and dark can be difficult. It is this frontier that researchers and engineers are actively exploring to push the boundaries of these technologies further. It is very likely that we'll see continued improvements in the next few years, making these tools even more powerful for both amateur and professional photographers.
How AI-Powered Tools Transform Regular Photos into Striking Silhouettes A Technical Analysis - Background Separation Algorithms Enable Precise Subject Isolation
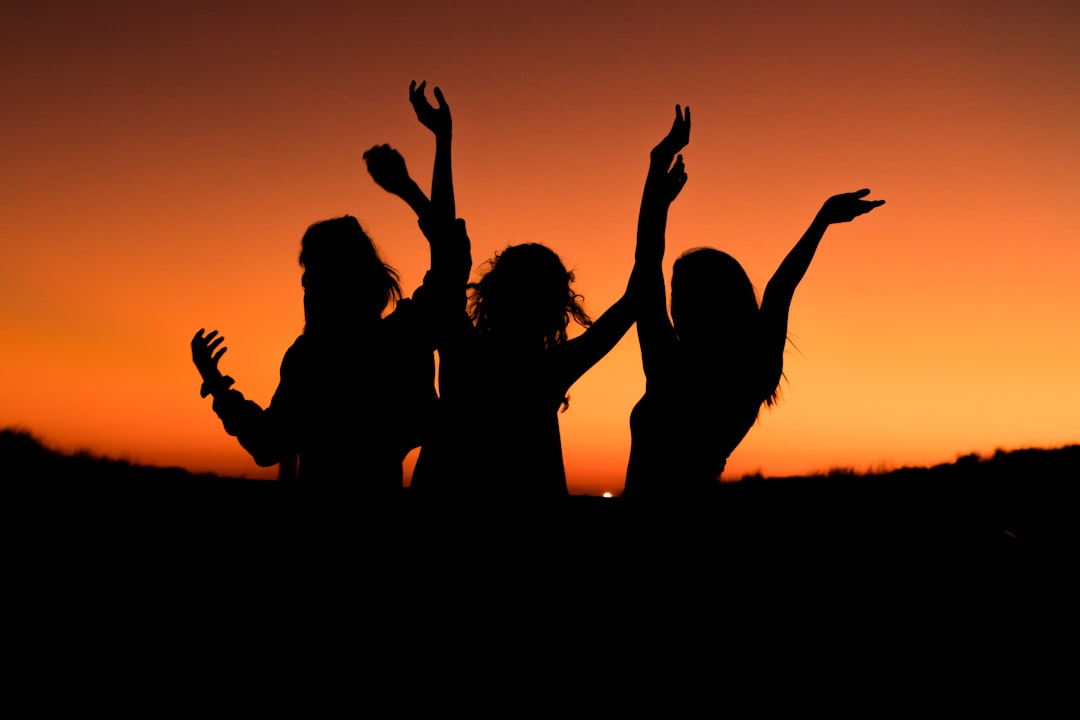
Background separation algorithms are revolutionizing how we isolate subjects in images, providing a level of precision previously unattainable. These algorithms, powered by machine learning, excel at distinguishing between the subject of a photograph and its surrounding environment. This capability significantly improves the efficiency of photo editing by automating a process that used to be painstakingly manual. Photographers can now spend less time meticulously removing backgrounds and more time focusing on the creative aspects of their work.
The applications of background separation extend across various fields. Graphic designers can quickly and easily create clean compositions, social media users can enhance their profiles with polished imagery, and the possibilities continue to grow. AI-driven image manipulation is clearly transforming how we approach photography.
It's important to note, however, that the effectiveness of background separation algorithms varies. The complexity of a scene, especially scenes with intricate details or challenging lighting, can impact the precision of the separation. Additionally, the quality of the underlying machine learning algorithms used plays a crucial role in determining the outcome. There's a clear need for continued research and development in this area to refine these algorithms and broaden their applications. Further advancements could yield more robust and accurate background separation, leading to even more compelling and visually arresting images.
AI-powered background separation algorithms are increasingly sophisticated, employing machine learning to identify not just the edges of objects, but also finer details like textures. This allows for precise subject isolation, even in scenes with intricate, busy backgrounds. These algorithms demonstrate a level of adaptability to various lighting conditions, adjusting their approach to maintain accurate subject separation in diverse environments. This dynamic adjustment is key to keeping the integrity of the subject during the isolation process, preventing unwanted distortions or artifacts.
Adding to the sophistication is the incorporation of depth estimation alongside background separation. This gives the algorithms a 3D understanding of the scene, enabling them to separate objects based on their relative distance and depth, much like how human vision works. Furthermore, some tools can perform background separation in real-time, which is proving revolutionary for tasks like live event photography or filmmaking. Being able to get immediate visual feedback on the results during the process itself significantly changes how on-the-fly editing is approached.
The impact of these advancements on workflow efficiency is notable. The automation provided by these algorithms has dramatically reduced the time spent on manually removing backgrounds, allowing photographers and editors to dedicate more time to creative pursuits. Interestingly, some AI tools are even starting to suggest potential subjects for isolation based on established compositional principles, influencing how photographers think about framing and arranging elements within the scene even before capturing the image.
However, the algorithms are also proving remarkably effective in challenging scenarios. Images with motion blur or low contrast between the subject and background—situations that frequently cause older techniques to stumble—are now handled with surprising success by these AI-powered methods. This enhanced robustness points to a significant step forward in image editing. Furthermore, the precision of the algorithms extends to specialized tasks such as watermark removal. The AI can identify and remove unwanted watermarks without affecting the underlying image, demonstrating a nuanced level of manipulation for image restoration.
In some implementations, anti-aliasing techniques are integrated into the background separation process. This smooths out the edges after separation, ensuring a more natural and aesthetically pleasing transition between the subject and its new background. It's often a difficult aspect of image manipulation to get right, so this is a welcome improvement. Finally, the burgeoning use of generative adversarial networks (GANs) alongside these background separation algorithms opens up exciting new possibilities. It potentially leads to more photorealistic results when making modifications. We are starting to see the ability to seamlessly blend in altered backgrounds or make other contextual changes. This significantly expands the range of creative manipulation available for image enhancement.
How AI-Powered Tools Transform Regular Photos into Striking Silhouettes A Technical Analysis - Pattern Recognition Technology Preserves Key Visual Elements During Conversion
In the process of turning ordinary photographs into striking silhouettes, pattern recognition technology acts as a vital guide, ensuring that crucial visual elements are preserved. This technology, powered by machine learning and neural networks, leverages sophisticated algorithms to pinpoint and maintain the essential aspects of an image. These algorithms are trained to recognize and retain features that contribute to the overall structure and artistic value of the original image. Consequently, the resulting silhouette is not just a simple black and white cutout, but retains a degree of the original image's inherent qualities, enhancing its visual impact.
It's important to note that the success of this technology is not always uniform. The complexity of the original photograph, particularly the presence of intricate details or challenging lighting, can affect the accuracy with which essential elements are preserved. Moreover, the quality of the underlying algorithms plays a substantial role in the final outcome. Ongoing research and development in this domain are critical for improving the precision and robustness of these techniques. As the field advances, we can expect a higher level of refinement in image transformations, leading to even more creative possibilities within photography.
AI-driven image manipulation, particularly in the context of silhouette creation, heavily relies on pattern recognition technologies to preserve essential visual elements during the conversion process. These technologies analyze the intricate interplay of colors, textures, and spatial relationships within an image, enabling algorithms to maintain a sense of the original photograph's depth and character, even when reducing it to a simplified, black-and-white form.
For instance, when transforming a full-color image into a silhouette, techniques like high dynamic range (HDR) processing are often utilized to balance the distribution of light and shadow. This careful balancing act ensures that the silhouette retains a sense of depth and contrast that's essential for conveying the original scene's visual impact. Further, algorithms are becoming more sophisticated in their ability to identify and retain important textural features in both the foreground and background elements of an image, a capability that adds a degree of complexity to otherwise minimalist black-and-white forms.
Interestingly, some newer image enhancement tools incorporate a degree of adaptive learning, where the algorithms dynamically adjust based on user interactions. As a photographer applies edits or tweaks the parameters of a particular effect, the algorithms track these changes, learning patterns and improving the suggestion of future adjustments. This can streamline the workflow by offering more intuitive editing recommendations tailored to individual user preferences.
Another area where pattern recognition is pivotal is in real-time image processing. Background separation, for example, has become far more accurate due to advancements in machine learning algorithms. Now, photographers can see the effects of their edits immediately, significantly altering how edits can be implemented in settings where the visual feedback loop is crucial, like live event photography or filmmaking.
Beyond standard background separation, cutting-edge algorithms are incorporating depth estimation to understand the three-dimensional structure of a scene. This provides algorithms a more nuanced grasp of how subjects relate to their surroundings, especially helpful in images with intricate layering or overlapping elements. This improved understanding of spatial relationships helps create a more natural and coherent separation, crucial for maintaining visual clarity.
However, pattern recognition extends beyond simple background manipulation. Some systems can now accurately recognize and remove watermarks from images without leaving distracting remnants. This is made possible through sophisticated pattern recognition techniques that distinguish watermark patterns from the underlying photograph data. This ability to clean up images and remove unwanted artifacts showcases how pattern recognition is being used for more than just aesthetics.
Moreover, the rise of generative adversarial networks (GANs) is revolutionizing how we approach background manipulation. GANs have the ability to learn from vast datasets of images and subsequently generate entirely new backgrounds that seamlessly blend with existing foreground subjects. This capability offers a new frontier in creative expression, allowing photographers to imagine and build scenes in entirely new ways.
While AI-powered enhancements continue to evolve, a productive synergy is emerging between automated algorithms and manual human creativity. Photographers can often fine-tune the results of AI algorithms, offering a degree of artistic control and allowing for a personalized touch. This collaborative approach enables a wider exploration of compositional possibilities, bridging the gap between technical advancements and artistic vision.
The ongoing advancements in pattern recognition, paired with the growing capabilities of machine learning, are influencing the future of photography. These developments will likely create more intuitive editing tools, and provide opportunities for increased levels of creative freedom. However, with this freedom comes the need to carefully consider how AI is changing the nature of visual representation in photography. It will be important to remain critical of these tools and ensure their proper use.
How AI-Powered Tools Transform Regular Photos into Striking Silhouettes A Technical Analysis - Real-time Processing Allows Live Preview of Silhouette Effects
The advent of real-time processing has significantly altered how we interact with image manipulation, specifically in the creation of silhouette effects. Photographers and editors can now see the effects of their adjustments as they are made, providing immediate feedback that enhances the editing process. This dynamic interaction allows for a more fluid and intuitive workflow, where users can experiment with different effects and fine-tune them on the fly.
AI-driven algorithms are crucial in this real-time environment. They can quickly analyze various aspects of an image, including lighting conditions, depth perception, and subject isolation, contributing to the overall quality of the resulting silhouette. This helps ensure the final silhouette is both visually arresting and retains a sense of the original image's composition.
However, it's important to recognize that creating perfect silhouettes in complex scenes can still be difficult. The accuracy of real-time processing in such situations remains a challenge, and further advancements in AI are needed to handle the nuances of diverse image conditions. Despite these hurdles, this evolving area of image processing shows tremendous potential for both improving the photographer's workflow and allowing for a more expansive range of creative expression. The ability to experiment and instantly see the results creates a more engaging and dynamic approach to photography.
Real-time processing, particularly leveraging the parallel processing capabilities of GPUs, is becoming increasingly important in photography, especially for live previews of silhouette effects. This ability to see the changes instantaneously is crucial for photographers, especially during dynamic shoots, offering a level of responsiveness that was not possible before. The computational demands of real-time processing are substantial, and it's interesting to see how the algorithms are being optimized for different hardware configurations.
Furthermore, the algorithms used for silhouette creation are becoming more adaptive. They can now adjust their behavior based on the unique characteristics of each image, automatically fine-tuning parameters to optimize detail preservation and contrast enhancement. It's as if the algorithm is "learning" the photo as it's processing it, providing more precise results. It will be interesting to see how this area progresses, especially as we develop even more sophisticated machine learning tools.
Moreover, some systems are starting to delve into temporal analysis within the real-time processing pipeline. This allows for creating motion-based silhouettes in videos, reacting to changes frame-by-frame. While still in its early stages, it is a fascinating area that opens up numerous opportunities for video editing and creative storytelling. It's exciting to consider the creative possibilities that could arise from this integration of real-time processing with video editing.
The actual process of silhouette creation also utilizes increasingly sophisticated multi-layered perception. The algorithms often differentiate between depth cues and surface textures, allowing them to create a nuanced representation in the final silhouette. This is important because it helps to ensure that the important elements and contours are still visible even when the image is reduced to a very simplified form. Understanding how the algorithms decompose the image into its basic components will be an interesting area for further research.
Another important part of silhouette generation is preserving the edges. The algorithms are being designed to optimize the balance between abstraction and form. They need to create a smooth, artifact-free edge while maintaining a clear delineation of the subject. We've seen this happen with other AI-powered image editing functions, and the optimization of the edge detection and refinement in this context will be interesting to monitor.
There is also significant improvement in how the tools manage shadow dynamics. AI-powered tools can now generate not just static, but dynamic shadows as well. These shadows can respond to changes in lighting during the editing process, leading to a more consistent and visually cohesive outcome. This improvement is a great example of how the realism of silhouette manipulation is continuously enhanced by these technologies.
Interestingly, the growing integration of depth sensors into modern cameras is changing the way silhouettes are created. The ability to capture a 3D understanding of the scene provides valuable information for generating more realistic silhouettes, and photographers can modify them in real-time based on the depth of elements within the frame. It's an exciting area to monitor as it brings a new level of interaction and flexibility to the photographer.
These advanced methods are also tackling issues like noise reduction. Neural networks are effectively minimizing the presence of noise in silhouette images, especially in those generated from high ISO images, which are traditionally prone to a grainy appearance. The removal of noise creates cleaner edges and sharper silhouettes, even in difficult shooting conditions. It's a small but important enhancement to the overall process of silhouette creation.
We're also starting to see the influence of perceptual psychology on the algorithms. The systems are designed to better replicate principles like figure-ground organization, making the final silhouettes easier for viewers to understand and interpret. It's a reminder that image creation isn't simply a matter of pixels, but also a deep understanding of how human perception influences image recognition.
Finally, the advancement of pattern recognition in AI-powered tools has enabled a significant step forward in watermark removal. These algorithms are now capable of intelligently identifying and removing watermarks without compromising the quality of the photo. It shows a sophisticated ability to differentiate between the unwanted watermark patterns and the underlying original content, allowing for seamless image restoration.
While still in its early stages, it's evident that the combination of real-time processing, adaptive algorithms, and an increasingly nuanced understanding of image structure is significantly enhancing silhouette generation and the entire realm of image editing. The future of photography looks to be defined by a fascinating combination of AI-powered automation and human creativity. It's an interesting time to observe this field evolve and consider how it's redefining how we create, manipulate, and interact with photographs.
Create incredible AI portraits and headshots of yourself, your loved ones, dead relatives (or really anyone) in stunning 8K quality. (Get started for free)
More Posts from kahma.io: