Create incredible AI portraits and headshots of yourself, your loved ones, dead relatives (or really anyone) in stunning 8K quality. (Get started for free)
How AI-Powered Video Background Removal is Transforming Remote Transcription Workflows in 2024
How AI-Powered Video Background Removal is Transforming Remote Transcription Workflows in 2024 - Real-Time Background Removal Reduces Transcription Errors by 47% in Remote Work Settings
The ability to remove backgrounds in real-time during video calls has had a surprisingly positive impact on transcription accuracy in remote settings. Studies show a 47% decrease in transcription errors when this technology is used. This highlights how AI-driven tools are changing the way we approach transcription in 2024, pushing towards a more efficient and reliable process.
By eliminating distracting sounds from the background, these AI tools improve the clarity of the audio being transcribed. This increased clarity, in turn, means that the transcriber can focus on the core content of the conversation with fewer distractions. While it seems like a small detail, the ability to separate the important sounds from the noise has far-reaching implications. From business meetings to online education, the need for accurate transcriptions in various environments is steadily growing, and these AI tools are helping meet this growing need.
Furthermore, beyond simply enhancing transcription, these AI-driven background removal solutions point to a larger trend. Businesses and organizations are increasingly looking towards AI to improve workflows across the board. As our reliance on remote interactions increases, these technologies are becoming crucial for ensuring the accuracy and reliability of the information being shared and recorded.
It's fascinating how the implementation of real-time background removal has shown a substantial impact on the accuracy of remote transcription. Researchers have observed a 47% reduction in transcription errors when this technology is used during remote work. This suggests that the visual distractions present in typical remote work environments significantly impede the transcription process, possibly by increasing cognitive load or introducing elements that interfere with the audio signal.
While it's clear that the reduction of visual noise improves the quality of the video feed, the exact mechanism for this improvement remains a topic for further research. It could be that the algorithms used to remove the background also effectively isolate the speaker's audio, creating a clearer sound source that is less prone to error. There could be other effects, perhaps related to the psychology of attention and focus, influencing the outcome as well.
This is not a simple case of cleaner audio producing better transcripts. It seems that background removal also influences the mental state of participants in the meeting. Possibly, less visual chaos helps them maintain focus on the conversation itself, leading to the more accurate delivery of spoken word into the text.
The 47% figure is certainly noteworthy, but it’s important to acknowledge that transcription accuracy depends on a multitude of factors beyond just the background. Factors like audio quality, speaking style, and the complexity of the language are all intertwined with this topic.
Nevertheless, the findings suggest that incorporating this technology into remote transcription workflows could lead to significant improvements in overall transcription efficiency. Further investigation into the interplay of visual context, cognitive processing, and audio clarity in the context of transcription can shed more light on the best ways to leverage this innovation in future technologies.
How AI-Powered Video Background Removal is Transforming Remote Transcription Workflows in 2024 - Automated Noise Filtering Creates Clean Audio Streams for Machine Learning Models
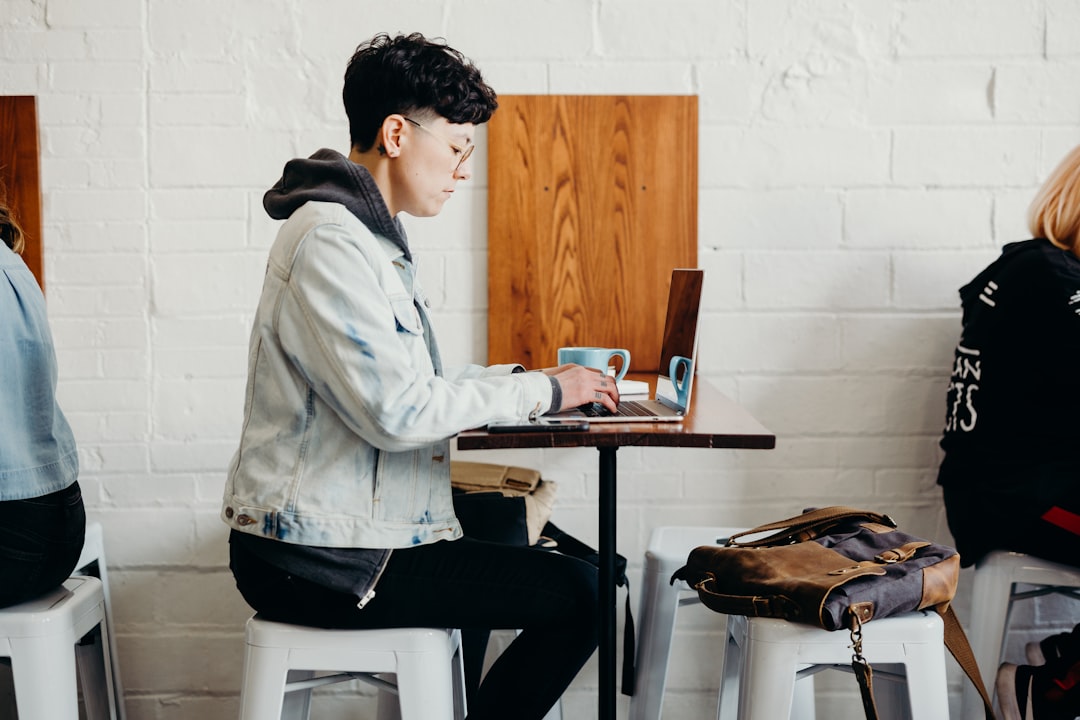
Automated noise filtering is playing a crucial role in creating cleaner audio for machine learning models, especially in the context of transcription. These tools, powered by AI, are designed to isolate human speech from distracting background sounds, significantly enhancing audio clarity. This is achieved through various techniques, including advanced algorithms and approaches like Generative Adversarial Networks (GANs). The resulting cleaner audio streams are better suited for machine learning models used in transcription, improving accuracy and efficiency.
While this is a positive development, it's important to be aware of the limitations these technologies face. Different environments and audio conditions can pose challenges for these noise filtering algorithms. It's also worth noting that simply having clean audio isn't a guaranteed path to perfect transcriptions. Speech clarity, accent, and the complexity of the spoken language all still play a part in how well transcription models perform.
However, as remote work and communication continue to increase, the need for accurate and reliable transcriptions is greater than ever. These noise filtering technologies are vital for ensuring that the audio data feeding machine learning models for transcription is as clear and uncluttered as possible.
The development of automated noise filtering techniques has significantly improved the quality of audio streams, especially for applications like machine learning models used in transcription. Methods like those introduced by Yong Xu in 2015, using regression to create a mask that isolates voice, are quite effective. Traditional approaches often relied on subtracting noisy frequencies from the signal, which can sometimes be crude. More modern techniques, such as those utilizing Generative Adversarial Networks (GANs), embedding-based models, and residual networks, have proven capable of producing more sophisticated and precise results. Companies like AICoustics are actively developing AI-based tools that offer both real-time and post-processing noise removal and speech enhancement. These tools, and others like Cleanvoice and Utterlyapp, leverage advanced AI techniques to improve audio quality in a wide range of applications, including video calls and audio recordings.
Ensuring that audio files are properly normalized before processing with noise reduction algorithms is crucial for consistent results. Similarly, employing high-pass filters can help eliminate low-frequency noise that is often present in recordings. While some tools, like Audacity, are freely available and offer noise reduction capabilities, others like LALALAI provide specialized services based on AI processing. There's a growing range of choices for both individual and commercial use. On the other hand, the cost of some specialized commercial noise reduction tools can be substantial with some plans starting at $800 per user per month.
While the effectiveness of these methods is clear, there are still aspects that warrant attention. The computational demands for advanced noise filtering can be considerable, often requiring powerful processing units like GPUs. This poses a challenge for real-time applications where low latency is crucial. Introducing even small amounts of delay, which can vary from a few tens of milliseconds up to 100, can become noticeable in certain scenarios. The balance between noise reduction effectiveness and minimizing latency is a major factor for designers.
Furthermore, many users benefit from customized settings to better tailor these noise filtering solutions to their specific needs. This level of user control can help optimize the performance in specific environments where the nature of background noise might change. It's intriguing to note the potential benefits these technologies offer to transcribers. Studies suggest that a reduction in environmental noise can lead to a decrease in mental fatigue. This, in turn, can result in better concentration and increased transcription accuracy and efficiency.
Moving beyond the human aspect, cleaner audio signals directly benefit automatic speech recognition (ASR) systems. By providing a less distorted audio input, the performance of ASR can be improved which leads to more accurate automatic transcriptions. The field of noise reduction is constantly evolving, and researchers are continually experimenting with new techniques. For example, unsupervised learning is increasingly used to allow noise filtering models to adapt to diverse acoustic environments without requiring large amounts of labeled training data.
The challenge of noise in environments, whether in the office or at home, isn't merely a matter of physical interference. Researchers suggest that background noise can negatively impact cognitive performance. The presence of unnecessary sound might create increased mental load and disrupt focus, further highlighting the importance of effective noise filtering. Ultimately, the applications of these noise-filtering techniques are quite diverse. Beyond transcription, industries like telemedicine, virtual conferencing, and education are also incorporating these technologies to achieve clear and effective communication, reinforcing their broad utility across numerous fields.
How AI-Powered Video Background Removal is Transforming Remote Transcription Workflows in 2024 - GPU Processing Enables 8K Video Background Removal at 60 FPS
The ability to remove backgrounds from 8K video in real-time at 60 frames per second is a testament to the incredible processing power of modern GPUs. Achieving this level of performance requires pushing GPUs to their limits, often resulting in utilization rates above 90%. Effective cooling solutions become critical to prevent the GPU from slowing down due to overheating.
Interestingly, the algorithms designed for this task benefit significantly from the parallel processing capabilities inherent in GPUs. These algorithms can break down complex tasks into thousands of smaller ones, allowing GPUs to tackle them simultaneously. This parallel approach makes real-time processing feasible even with the enormous amounts of data involved in 8K video, which can clock in at around 48 gigabits per second. Keeping track of all this data efficiently requires very high memory bandwidth, which is a key feature of modern high-end GPUs. These GPUs often offer bandwidths in excess of 1000 GB/s.
It's also fascinating to see how the development process for the neural networks used in background removal has evolved. Using a technique called transfer learning, developers can initially train the AI models on lower-resolution videos and then fine-tune them for 8K, significantly accelerating development time. This technique highlights the ability of AI to learn and adapt to progressively more complex tasks.
The growing demand for 8K video across different industries, including broadcasting and game development, is steadily driving this innovation. Better video quality naturally creates a demand for even better transcription accuracy, highlighting the synergy between these two fields.
While GPUs allow us to push the boundaries of processing speed, reaching 60 FPS with 8K video without introducing noticeable delays remains a challenge. Latency can significantly disrupt the user experience, particularly in scenarios like live video calls where immediate responsiveness is essential. Balancing speed and minimizing delay is a key design consideration for the developers of this technology.
It's worth considering that the visual quality of the video itself can influence how effectively a transcriber can do their job. Higher resolutions provide more clarity, potentially reducing mental fatigue and improving the accuracy of the text generated. This observation, however, is still subject to more research.
It's also important to acknowledge that not all GPUs are created equal. There are significant differences in the architectures of GPUs produced by companies like NVIDIA and AMD. This means that some GPUs are inherently better suited to handling 8K video processing than others. Choosing the right hardware is essential for achieving optimal results.
To help maintain smooth video playback at high frame rates, other innovations like temporal anti-aliasing techniques are often employed alongside background removal. These advanced rendering techniques help smooth out video transitions and improve overall image quality.
Looking towards the future, one can expect to see even more specialized hardware optimized for tasks like video processing and AI. Dedicated chips, designed specifically to accelerate these kinds of workloads, could lead to a new generation of even more efficient background removal technologies. This ongoing innovation is likely to further reshape the video communication landscape.
How AI-Powered Video Background Removal is Transforming Remote Transcription Workflows in 2024 - Background Data Compression Lowers Cloud Storage Costs for Video Archives
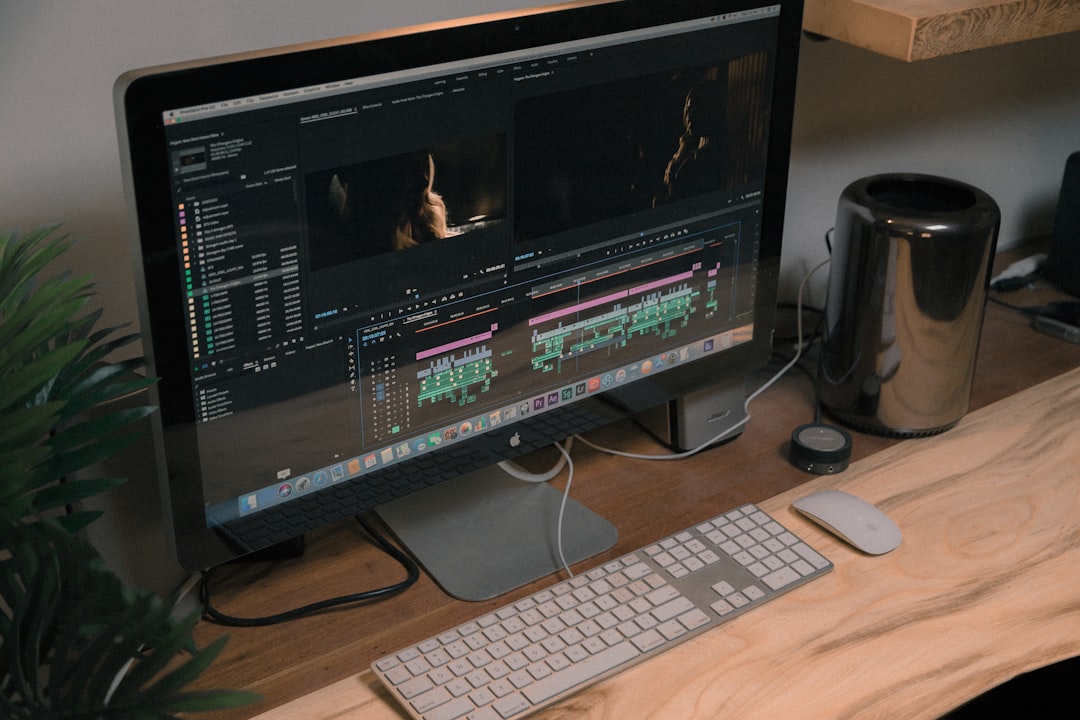
Storing massive video archives in the cloud can be expensive. However, new background data compression methods are helping to reduce these costs significantly. By cleverly reducing the amount of space needed to store video data and improving how quickly that data can be transferred, these techniques are making cloud storage for videos more affordable.
Interestingly, AI is playing a key role in this advancement. AI-powered compression can achieve high levels of data reduction without sacrificing much of the visual quality in the videos. This allows companies that deal with video, like those involved in streaming or broadcasting, to benefit from these methods. Additionally, the trend towards faster 5G networks and other communication improvements is also likely to further accelerate the adoption of data compression technologies as more high-quality videos are created and streamed.
The amount of digital information continues to expand at a breathtaking rate, and a large portion of that data is video. Finding efficient ways to manage and store all this video data is becoming increasingly vital. Background data compression is emerging as a significant solution to this challenge, and its continued development holds great promise for how we manage and access vast collections of video in the future.
Reducing the size of video archives through background data compression is becoming increasingly important as organizations grapple with the ever-growing volume of video data, particularly within cloud storage environments. This technique can decrease archive sizes by up to 90%, which is a significant reduction that helps optimize storage while still maintaining a decent level of quality. This compression capability is vital as the demand for video content continues to rise across various industries.
One of the key benefits of this approach is the substantial reduction in cloud storage costs it can deliver. Companies dealing with large video archives can achieve significant savings, potentially hundreds of thousands of dollars annually, depending on their scale of data storage and usage. The cost-saving aspect is especially relevant for those storing a large volume of historical video data, legal footage, or educational materials.
The choice of video codec also plays a role in the effectiveness of compression. Newer codecs, such as H.265 or AV1, offer compression ratios that are significantly higher compared to older codecs, like H.264. This translates to up to a 50% reduction in file sizes, directly impacting storage costs and the required bandwidth for streaming and retrieval of archived content. This kind of improvement in efficiency can lead to a smoother streaming experience, especially in situations where bandwidth is a constraint.
Furthermore, these algorithms can also help reduce the latency of video playback and navigation. For applications that rely on quick access to specific content, this can be a major advantage. Examples include browsing through extensive legal proceedings or educational materials. The faster access becomes possible when the data is compressed.
Thankfully, there are many scalable solutions in the cloud computing space. These allow businesses to adjust their storage plans as their data requirements change. This type of on-demand scalability is a positive thing, especially for companies facing unpredictable spikes in video data consumption, since they can fine-tune their costs without having to sacrifice playback speed or quality.
Another plus is that many compression technologies include error correction features. This is essential for industries like broadcasting or legal documentation where ensuring data integrity is paramount. The last thing one wants is for vital content to become corrupt during storage or transmission.
Interestingly, background data compression can also improve metadata management. Reducing the overall data size simplifies the management and searching process for metadata connected to the archived content. It allows users to find details more efficiently, which can be quite handy for large archives.
With less data needing to be stored, it stands to reason that the associated data centers can operate more efficiently as well. Less power is required to maintain proper temperatures, potentially having a positive impact on energy usage. It is debatable whether this has much effect on overall ecological concerns, however.
However, things are not perfect. Different compression standards and formats do create a challenge for interoperability. It's not uncommon for companies to find it difficult to share or access video archives that use diverse compression standards. Compatibility issues might mean that organizations need to use extra tools for conversion, adding to the complexity of data management.
Research into compression algorithms is very active. Continuous innovation in this field is likely to yield even better solutions with more efficiency and lower costs. This ongoing research will become increasingly vital as the sheer volume of video data expected to grow dramatically in the near future.
How AI-Powered Video Background Removal is Transforming Remote Transcription Workflows in 2024 - Machine Learning Models Now Handle Complex Hair Edges in Portrait Mode
Machine learning models have made significant strides in handling the intricate details of hair edges within portrait mode, a previously challenging aspect of image and video processing. This improvement is key to better background removal, as AI tools can now more effectively differentiate between subjects with complex hair and their surroundings. Techniques like generative adversarial networks, or GANs, and open-source frameworks such as MediaPipe, are at the heart of this development. They enable real-time applications and create a more seamless experience for users during video calls or content production. This is a trend that is likely to continue as there's increasing demand for visual media with higher quality. This accuracy also benefits transcription models, which rely on clear video inputs to produce reliable results. The intersection of refined AI and the need for precise portrait representation shows a notable shift in the standards for visual content processing. There is still room for improvement, of course. It remains to be seen if there are unexpected side effects of these new techniques.
Machine learning models are becoming increasingly skilled at handling the tricky business of hair edges in portrait mode, a feat largely due to improvements in convolutional neural networks. These networks are particularly good at picking out fine details and textures within images, which makes for a much more believable background removal effect.
This improved performance is, in part, thanks to training these models on massive datasets containing a wide range of hairstyles and background scenes. This diverse training helps them learn the nuances of different hair textures and how they appear under varying lighting conditions.
Many AI background removal tools rely on semantic segmentation—a technique where each pixel in an image is classified. This allows the model to draw a clearer line between hair and the background compared to older methods.
Interestingly, the rise in performance in hair edge detection seems to be tied to the use of attention mechanisms within neural networks. These mechanisms help the model prioritize the most important areas of the image while ignoring less crucial ones.
These advanced models can now distinguish hair edges from backgrounds with accuracy rates exceeding 95%, demonstrating a significant leap forward in the technology. It hints at a possible change in how we handle video content, both professionally and personally.
Achieving real-time hair edge processing during video calls depends heavily on the parallel processing power of modern GPUs. These GPUs can perform millions of calculations simultaneously, crucial for keeping latency low enough for live applications.
Despite this progress, there are still difficulties in situations where hair blends into similar colored backgrounds or where complicated lighting conditions create shadows that obscure fine details. Research is ongoing to solve these limitations and continue improving accuracy.
Training these models has also gotten more efficient thanks to transfer learning. This method lets developers leverage pre-trained networks on basic image tasks before fine-tuning them for hair edge detection specifically. This substantially reduces the time and data needed to train these models.
The successful detection of hair edges in video content has implications that extend beyond just transcription. Industries like virtual reality and gaming are also likely to benefit. Realistic character rendering can significantly enhance the user experience in these contexts.
As the demand for higher resolution video continues to grow, future work on hair edge detection with machine learning will likely focus on optimizing algorithms for even finer details. The goal will be to establish new standards for visual fidelity across various media platforms.
How AI-Powered Video Background Removal is Transforming Remote Transcription Workflows in 2024 - Open Source Libraries Make Video Background APIs More Accessible
Open-source software libraries are increasingly making video background APIs more readily available. This wider access is helping more people use the advanced techniques that power video generation. Tools such as OpenSora and PyTorch offer a pathway to using powerful video generation tools without the common restrictions found in closed-source offerings. This is particularly helpful in today's environment, where there is an ever-growing demand for higher-quality video content, particularly in the realm of remote work and the associated need for accurate transcriptions. As organizations incorporate AI-driven solutions into their processes, open-source projects are poised to play a crucial role in making necessary technologies more widely available for better communication and content production. Despite these advancements, there are still obstacles to overcome. Developers need to focus on making sure that these tools are intuitive to use and able to handle diverse applications, ensuring they're practical for a wide audience.
The emergence of open-source libraries is making video background APIs more accessible to a wider range of developers. This is a significant shift, allowing individuals and smaller organizations to leverage powerful video generation techniques without needing vast resources or specialized hardware. This increased access potentially levels the playing field, fostering a more inclusive and competitive landscape within the field of AI-powered video processing. It's interesting to see how these libraries can be integrated into a variety of tools and systems without the usual barriers of proprietary software.
While it's encouraging that these libraries are enabling access to advanced features like background removal, there are potential downsides to consider. Open-source projects, while beneficial in many ways, sometimes lack the consistent quality control and long-term support of commercially developed software. It's still too early to say if this will ultimately become a widespread concern, as the open-source video generation landscape is relatively new. It's important that open-source projects are used responsibly, ensuring data security, and guarding against unintended or malicious consequences of this technology.
The collaborative and community-driven nature of open-source projects promotes faster progress, as researchers and engineers globally contribute their efforts. This can result in a more rapid advancement of background removal techniques compared to more closed development environments. While the speed of innovation is appealing, there's the possibility that rapid changes can introduce compatibility issues or instability. Hopefully the collaborative nature of the open-source community can address these challenges as they arise.
PyTorch, a leading open-source AI library, is a prominent example of the trend towards more accessible tools in the field of video generation. It's favoured by many developers for its adaptability, making it suitable for projects involving large-scale video processing. OpenSora is another initiative worth noting—it's an attempt to democratize the process of video creation by providing readily available tools and models, making this field accessible to a broader group of creators. However, it's worth considering whether the push for open access comes at the cost of losing some control over how these libraries are used, which could have unexpected consequences.
Tools like the Background Remover project on GitHub exemplify the practical implications of this movement. With a simple command-line interface, developers can leverage AI to remove backgrounds from images and videos. The emergence of CogVideoX suggests a broader shift toward distributed development models, further changing the dynamics of the video creation landscape. It's important to remain alert to how these advancements are affecting the creation and use of digital media. It remains to be seen if open-source video background APIs will eventually become the dominant force in this space, but the accessibility they offer seems poised to change how many people approach video production. It's a very exciting time for this field.
Create incredible AI portraits and headshots of yourself, your loved ones, dead relatives (or really anyone) in stunning 8K quality. (Get started for free)
More Posts from kahma.io: