Create incredible AI portraits and headshots of yourself, your loved ones, dead relatives (or really anyone) in stunning 8K quality. (Get started for free)
How Nvidia's AI Hardware Powers the Future of Professional Portrait Generation A Technical Deep Dive
How Nvidia's AI Hardware Powers the Future of Professional Portrait Generation A Technical Deep Dive - Nvidia's H200 GPU Powers 4K Resolution AI Headshots at 10x Speed
Nvidia's H200 GPU is revolutionizing AI-powered headshot creation, particularly in achieving 4K resolution at a speed that's ten times faster than older systems. This dramatic speed boost stems from the GPU's 141 GB of HBM3e memory and its exceptionally high memory bandwidth of 48 terabytes per second. These features are crucial for efficiently handling the complex computations required by generative AI, allowing for rapid image generation. This kind of speed improvement can be a game-changer for photography professionals, potentially leading to faster turnaround times and potentially lower production costs. The H200's capabilities not only stimulate competition among GPU producers but also suggest a future where AI has a widespread impact across various industries. While this brings advancements, it also raises questions about the industry's future direction. The sheer power of the H200 and its implications in AI are creating a new landscape for image generation.
The Nvidia H200, built on the Hopper architecture, boasts an impressive 141GB of HBM3e memory and a phenomenal 48 terabytes per second of memory bandwidth. This is almost double the bandwidth of its predecessor, the H100, signifying a significant leap in processing power specifically tailored for AI tasks, like generating high-resolution portraits. This massive increase in memory bandwidth is particularly crucial for handling the large datasets needed by generative AI models.
Interestingly, the sheer scale of the H200's capabilities was highlighted by a recent project where over 100,000 of these GPUs were deployed in just under three weeks. Such a deployment normally would take years to accomplish. The speed and scale of this project suggest a growing demand and remarkable efficiency in the deployment of these specialized AI accelerators.
It's notable that this type of rapid adoption is making the H200 a key player in the AI landscape, pushing the boundaries of what's possible in the field. However, this kind of dominance inevitably leads to increased competition. Other companies, like AMD with its MI300X, are pushing for a piece of this market. It remains to be seen if the advantages of the H200 in terms of performance, energy efficiency, and memory bandwidth will give it a lasting competitive edge.
A core aspect of the H200's design is its focus on efficiency and high-performance computing. It's specifically engineered to handle the complex mathematical operations required by modern AI, including deep learning algorithms critical for generating intricate and realistic images. Its architecture, featuring multiple NVLink connections, offers immense flexibility for distributing processing tasks across a large number of interconnected GPUs.
The Nvidia DGX H200, a system incorporating eight H200 GPUs, represents the culmination of this engineering. This system offers a massive 1128GB of GPU memory, emphasizing the massive data requirements of AI image generation, particularly at high resolutions like 4K. The growing energy consumption of AI models has prompted the search for more efficient solutions. The H200 tackles this problem directly by providing the computational power required while aiming for lower power consumption compared to previous generations.
Ultimately, the H200 reflects the rapid advancement of AI and the growing demand for efficient hardware designed to handle these complex tasks. Its ability to generate high-quality images and facilitate real-time adjustments of these images is changing the landscape of portrait generation. But we're also at a point where the rapid pace of change brings with it a lot of questions about the long-term consequences and the sustainability of these developments.
How Nvidia's AI Hardware Powers the Future of Professional Portrait Generation A Technical Deep Dive - RTX 4090 Hardware Requirements for Local Portrait Generation Models
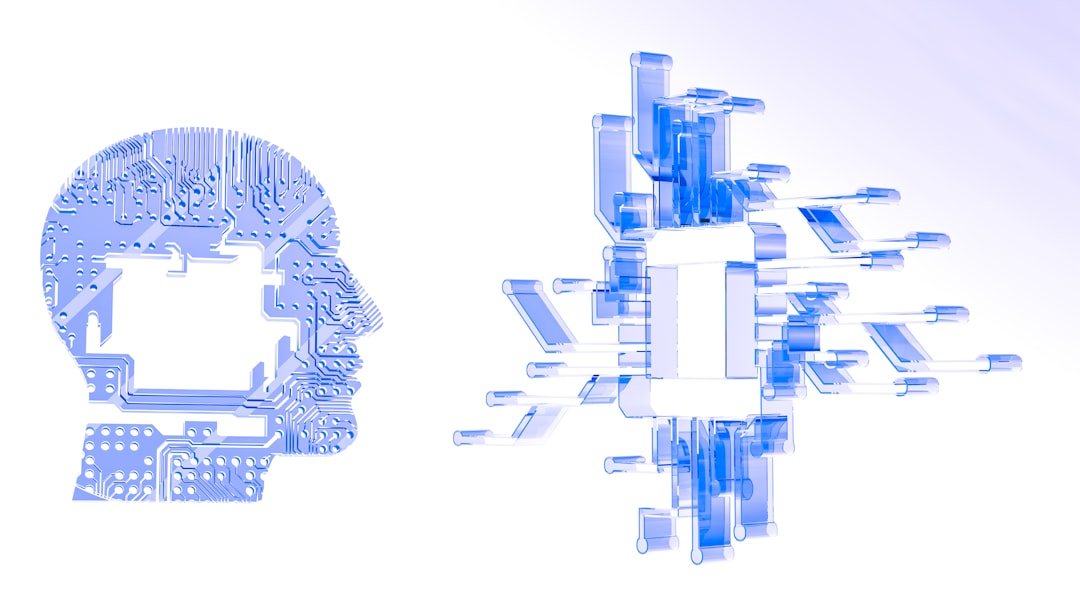
For individuals working with AI-powered portrait generation locally, the RTX 4090 presents a compelling hardware choice. Its Ada Lovelace architecture provides a substantial performance boost, roughly four times faster than previous generations, which is critical when working with the demanding computational needs of these models. However, this power comes at a price; the card necessitates a powerful system with a minimum 850-watt power supply to handle its 450-watt graphics power draw. This is a significant consideration for those seeking to integrate this into their workflow.
The RTX 4090 includes advancements in ray tracing and offers a large VRAM capacity. These features are important for ensuring that the model runs smoothly and provides the desired results. Additionally, the high processing power of this card suggests it is well-equipped to handle the evolving demands of future AI models. This suggests it might be a wise investment for those looking to future-proof their work, given that AI-related tasks in portrait photography are likely to become more sophisticated.
Ultimately, while the RTX 4090 presents a path to enhanced efficiency and detail within AI-driven portrait generation, it is not without its challenges. The high hardware requirements might make it unsuitable or too expensive for some photographers. Its potential to reshape the landscape of portrait photography through the use of sophisticated AI is significant, but we should also be mindful of the ongoing changes within this field and how these changes might affect cost and accessibility.
The RTX 4090, built on the Ada Lovelace architecture, presents a compelling option for local AI portrait generation models, offering a significant performance boost over its predecessors. While its 450-watt power draw necessitates a robust 850-watt power supply, it's designed to handle demanding AI tasks efficiently. Its 24GB of GDDR6X memory plays a critical role in supporting high-resolution portrait generation, allowing for detailed textures and complex renderings.
However, managing the card's thermal output is a key consideration, requiring a well-designed cooling solution to prevent throttling during computationally intensive operations. Optimizing performance further necessitates a PCIe 4.0-compatible motherboard for enhanced data transfer speeds when dealing with large datasets used by generative AI models.
Interestingly, the RTX 4090's integrated NVENC encoder can potentially be utilized to create video-based headshots or animated portraits, suggesting a more versatile application beyond static image generation. The hardware benefits don't stop there. Its Tensor Cores are optimized for deep learning computations, potentially allowing for real-time image enhancements during the generation process. This could be a big deal for photographers, enabling faster iteration cycles and lowering overall post-processing time.
For photographers interested in accurate color representation, the RTX 4090's ability to drive high-end quantum dot displays is a significant advantage. The richer colors and improved accuracy can create a compelling difference in the final output. Furthermore, leveraging multiple RTX 4090s in a system could further amplify production capabilities, opening up avenues for parallel processing. This scalability is especially valuable for studios needing high output and quick turnaround times.
Finally, tailoring portrait generation pipelines to fully exploit the RTX 4090’s capabilities requires developers to understand CUDA core optimization. This optimization could unlock substantial performance boosts, making it easier to manage the processing load when creating detailed, realistic portraits. While the RTX 4090's power suggests a significant leap forward, its implementation within a studio workflow requires careful consideration of hardware requirements and the need to optimize software for its unique architecture. The increasing need for high-performance hardware and the impact of AI on portrait generation is fascinating and poses many questions about the future of this industry. We're moving fast and there’s a lot to think about with such massive computational power.
How Nvidia's AI Hardware Powers the Future of Professional Portrait Generation A Technical Deep Dive - Technical Architecture Behind kahma.io Professional Portrait Creation
The core of kahma.io's professional portrait generation relies on advanced AI algorithms and Nvidia's powerful hardware, specifically the H200 GPU, to create incredibly detailed and lifelike 8K resolution portraits. Users provide a set of five or more source images, and the AI sifts through them to extract the nuanced features and expressions that make each portrait unique. This approach simplifies the portrait creation process, making it accessible to everyone, from professionals aiming for studio-quality headshots to individuals seeking personal digital avatars.
What sets kahma.io apart is the sophisticated AI that goes beyond basic image editing. It generates truly personalized digital twins and can be used to create unique artistic interpretations of images. In a field where professional portrait photography can be a significant expense, kahma.io offers a potential cost-effective alternative, enabling people to create high-quality portraits without the traditional high costs. However, it's important to consider the tradeoffs, as the quality and potential limitations of AI-generated portraits compared to traditional methods might not be suitable for all applications. It will be interesting to see how AI like this evolves and how the balance between cost and artistic control shifts in the future of portrait photography.
### Technical Architecture Behind kahma.io's Professional Portrait Creation
Kahma.io's system for generating professional portraits relies on a fascinating mix of AI techniques. A core component is the use of Generative Adversarial Networks (GANs), where two neural networks compete to create increasingly realistic images. This 'battle' between networks helps produce portraits that surpass traditional editing tools in terms of naturalness and detail. It's a clever approach, as the constant push for improvement results in more convincing output.
Interestingly, users can actively participate in the portrait creation process through a real-time feedback loop. This lets them tweak and refine the image on the fly, streamlining the editing process and reducing turnaround time without sacrificing quality. It's similar to how we interact with other generative AI, offering a conversational approach to creating a headshot.
The platform's ability to handle multimodal input is also intriguing. It combines visual information from uploaded photos with textual descriptions to create portraits that not only look realistic but also reflect specific moods or themes. This feature enables a richer level of control over the final product, something that's difficult to achieve with standard photography alone.
One remarkable aspect is how kahma.io manages to keep the AI efficient despite the complexity of the tasks. The developers have employed techniques like model pruning and quantization, which essentially optimize how the model uses memory. This means the technology can be used on a wider variety of devices, lowering the barrier to entry for users who might not have access to super powerful computers.
It appears they have trained the AI on custom datasets with specifically labeled human features and expressions. This detailed training likely explains the system's ability to capture subtle facial features and emotional nuances. It's this level of attention to detail that truly distinguishes it from simpler portrait creation tools.
The architecture also allows users to experiment with different portrait styles by manipulating latent vectors within a high-dimensional space. This 'latent space exploration' lets them quickly generate variations of a portrait without having to start from scratch each time. It's a kind of shortcut to creative exploration, allowing photographers to quickly cycle through various ideas.
Further, the system can be linked with cloud processing, enabling users to offload demanding computational tasks to more powerful remote servers. This effectively bridges the gap for people who don't have cutting-edge hardware at their disposal, letting them benefit from the platform's capabilities.
There's even an AI-driven feature for background customization. Based on the subject's attire or the desired theme, the system suggests or automatically creates backgrounds that seamlessly blend with the portrait. It's a clever way to ensure the portrait is integrated into its context.
Another fascinating aspect is the simulated lighting. The system can recreate different lighting conditions, allowing users to see how a portrait would look under varying environments. It's a feature that adds depth to the experience and lets users explore more nuanced artistic effects.
Ultimately, kahma.io's design aims to make high-quality portrait generation more affordable. By refining the AI models and applying smart compression techniques, they’ve successfully reduced the cost of producing these images, making it a more accessible option for both professionals and hobbyists. This combination of quality and cost-effectiveness reveals the real potential of AI in the photography landscape, especially when it comes to headshots or other portraits.
How Nvidia's AI Hardware Powers the Future of Professional Portrait Generation A Technical Deep Dive - Cost Analysis Professional Photography vs GPU-Powered AI Portraits
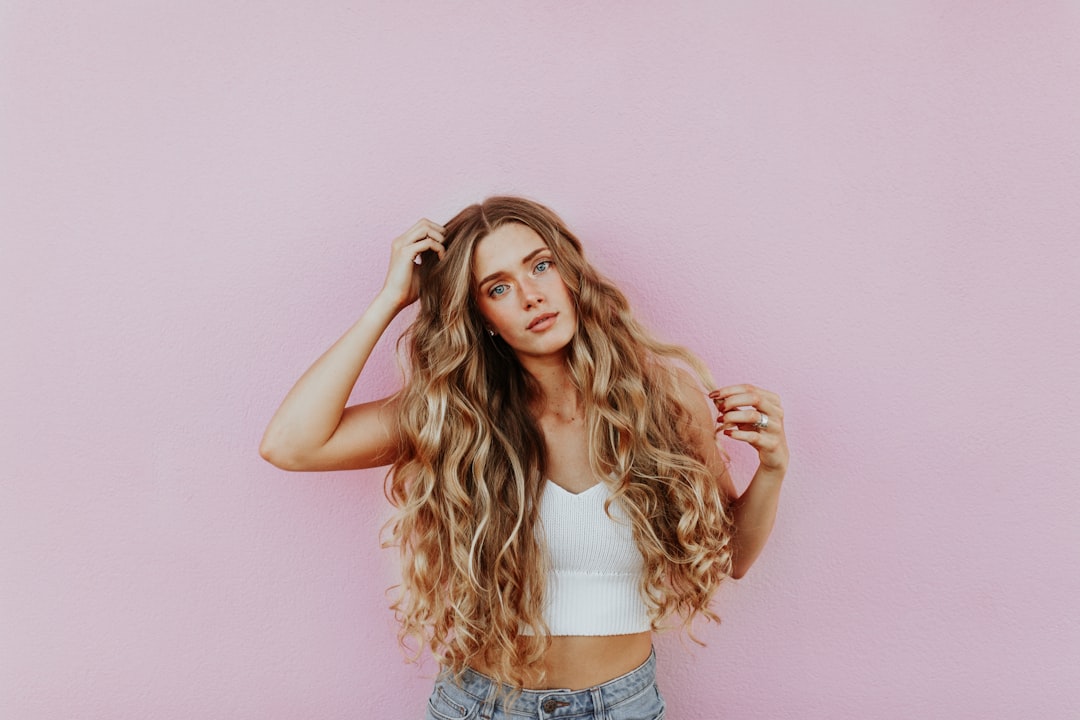
Examining the cost aspect of professional photography versus AI-generated portraits using powerful GPUs reveals a changing landscape. AI-powered portrait creation offers a more affordable path, particularly for budget-conscious individuals and businesses, without necessarily sacrificing image quality. The speed and convenience of generating portraits through AI are undeniable, minimizing the scheduling and logistical hurdles common in traditional photography. Additionally, AI's ability to refine images, enhancing clarity and vibrancy, is an attractive benefit.
However, while AI excels in generating high-quality, customizable portraits, it doesn't entirely replace the human element. The personal connection and artistic vision that professional photographers bring to the process are unique and difficult to replicate with current AI technology. The future likely involves a collaborative approach, where AI tools empower photographers and augment their creative abilities, rather than supplanting them altogether. The dynamic between AI and human photographers is a crucial development to watch as it might fundamentally alter the roles and skills valued within the photography industry. This evolving landscape emphasizes the continuing need to consider the nuances of each approach when choosing between professional photography and AI-driven portrait creation.
Professional portrait photography can be quite expensive, with costs varying widely. Factors like a photographer's experience, their location, and the complexity of the shoot, including revisions and set design, can easily push the price from a few hundred dollars to well over two thousand. In contrast, AI-generated portraits through platforms like kahma.io offer a significantly more affordable approach. These platforms often charge a fraction of the cost of traditional photography, making them a very attractive option for individuals and businesses on a budget.
Traditional photography involves many resources—studios, lighting setups, backdrops, and more—that can significantly increase costs. AI portrait generation, however, relies primarily on computational resources, which can result in lower overall operational expenses. This efficiency, while offering significant cost savings, doesn't negate the value that human photographers bring to the table. Their creativity, combined with the ability to build a connection with their subjects, is something that current AI systems find difficult to replicate in a nuanced manner.
Professional photographers often dedicate years to mastering their craft, learning lighting techniques, composition, and sophisticated post-processing. AI systems, while incredibly fast and efficient, are still lacking in the depth of understanding and artistry that comes from a seasoned professional's eye. Though they can churn out a vast number of portrait variations in just seconds—changing hairstyles, expressions, and backgrounds—traditional photography requires far more time to achieve similar changes, often taking hours of work to adjust even minor details.
AI portrait generators are trained on datasets containing millions of images, allowing them to learn a vast range of facial features, poses, and lighting styles. A photographer's portfolio, while curated, is restricted by their experience and the pool of subjects they have worked with. This difference in the training data sets might be a point of discussion as AI photography becomes more pervasive.
It's interesting that, even with advanced hardware like the H200 GPU, the costs associated with AI portrait generation remain lower than the ongoing operational costs of running a traditional photography studio. This computational cost advantage might become increasingly relevant as AI model complexity increases. AI systems frequently utilize automated feedback loops, allowing users to refine their images in real-time. In traditional photography, feedback is typically given after the shoot, extending the process and adding to the costs.
While AI portrait generation offers an affordable and accessible alternative to traditional photography, some might question the authenticity of digitally generated images compared to tangible photographs. In professional settings, especially corporate headshots, the perceived value of a traditional, physically printed photo can carry more weight in terms of social and professional context. The ongoing question of "authenticity" in portraits is worth exploring as AI image creation technologies progress.
How Nvidia's AI Hardware Powers the Future of Professional Portrait Generation A Technical Deep Dive - Memory Usage and Processing Time for High Quality AI Headshots
The rise of AI-powered headshot generation has dramatically altered the landscape of portrait photography, particularly in terms of the memory and processing time required to achieve high quality. Creating a high-quality AI headshot can be a lengthy process, with render times ranging from a few hours to nearly a full day, depending on the complexity of the desired output and the underlying technology. Nvidia's latest GPUs, with their massive memory capacities and incredible processing speed, have significantly sped up this process. GPUs like the H200, for instance, provide a significant advantage, handling the complex computations needed for AI image generation with remarkable efficiency. While this technology is making high-quality portraits more accessible and affordable, the trade-offs between speed, cost, and the artistic finesse of traditional photographers still warrant careful consideration. As AI continues its rapid development, it's important to monitor how it will reshape the industry and the role of human artists in portrait photography. The future of portrait generation is likely to involve a dynamic interaction between human creativity and AI capabilities, with both influencing the field in significant ways.
The creation of high-quality AI headshots involves a complex interplay of memory usage and processing time, which can vary significantly depending on several factors. For instance, the intricacy of an AI model and the desired resolution can impact memory needs, ranging from a few gigabytes to over 30GB. This variability underscores the importance of well-designed optimization strategies to manage system resources efficiently, ensuring a balance between speed and image quality.
Generating these portraits also takes time, varying from a fraction of a second to several minutes. More complex models, particularly those incorporating multi-modal inputs, demand more computational power, potentially making real-time applications challenging. The sheer size of the datasets used to train these models also impacts both memory and processing. While larger datasets enhance realism and detail, they necessitate exponentially greater memory and training time, sometimes taking weeks or even months on top-tier GPUs. This presents a significant barrier for smaller studios or individuals with limited resources.
Fortunately, powerful GPUs like Nvidia's H200 have unlocked the potential of parallel processing, drastically speeding up the generation process. Dividing the workload across multiple GPUs allows studios to reduce image creation times from hours to just a few minutes. However, advanced techniques like real-time ray tracing, while enhancing realism, add to the computational burden, leading to increased memory use and longer processing times. Specialized hardware often becomes necessary to maintain performance.
Fortunately, there are strategies to improve efficiency. Model quantization, for example, reduces memory requirements for AI applications without drastically compromising quality, making it possible to run advanced AI algorithms on less-powerful computers. This can broaden the accessibility of this technology for those with more modest budgets.
Additionally, AI portrait generation can support higher color depths, like 10 bits or more, leading to smoother gradients and more realistic skin tones. Managing such high-fidelity data effectively demands substantial processing power, making the choice of hardware critical for achieving professional standards. Real-time feedback loops, while enhancing user engagement, also require careful management of processing and memory resources to avoid lag during modifications.
The cost-effectiveness of AI systems is another crucial aspect. While the initial investment in hardware can be significant, AI portrait generation can reduce long-term operating costs compared to traditional photography. This is because traditional studios face constant expenses associated with studio space, equipment maintenance, and materials. AI systems can minimize or eliminate these ongoing costs.
Finally, as AI models become more complex, the demands on memory and processing resources will only increase. It's essential for developers and photographers to anticipate these future needs and be prepared for continual upgrades to both hardware and software to keep pace with the rapidly evolving capabilities of AI in portrait photography. This area holds numerous challenges and advancements that will be fascinating to watch in the years to come.
How Nvidia's AI Hardware Powers the Future of Professional Portrait Generation A Technical Deep Dive - Data Security Standards for AI Portrait Generation on Nvidia Hardware
The increasing use of Nvidia hardware in AI portrait generation necessitates a strong focus on data security. Nvidia's recent architectural advancements, such as Confidential Computing in Hopper and its refinements in Blackwell, aim to protect the sensitive data used during the portrait generation process. By implementing features like isolated, hardware-protected environments for processing, both the input data and the AI models themselves are safeguarded from unauthorized access. This is vital because AI models, particularly those related to generative AI in portrait photography, can be quite powerful and sensitive. Nvidia's focus on 'Trustworthy AI' emphasizes ethical development and transparency, crucial for building trust in these powerful technologies. The challenge for the future is maintaining this focus on security and integrity as the field develops, ensuring both photographers and clients are confident that their data is protected and the AI processes are used responsibly. The ability to balance powerful AI and robust data security is paramount for wider adoption and acceptance of AI in this domain.
Nvidia's work with AI safety and security is interesting, particularly as it relates to generating realistic headshots. They've joined the NIST AI Safety Institute Consortium, which shows they're aware of the need for responsible AI development, which is encouraging. The Hopper and Blackwell architectures have introduced features like Confidential Computing, aimed at improving the security of AI applications running on their GPUs, which could be crucial as the use of AI for portrait creation increases. This technology allows sensitive data, like facial features, to be protected during the process of creating images.
However, there's a growing need for standards around this technology. For example, the cost of high-quality professional portraits can be surprisingly high, potentially over $1,500, a figure that includes things like studio rental, post-processing, and specialized equipment. AI-based methods, though, promise lower costs by relying on specialized hardware. This shift also brings up questions about data privacy. Since AI-based methods are often working with sensitive personal data like facial images, we need to be sure they comply with regulations like GDPR, otherwise, we could see significant penalties.
Nvidia's GPUs, particularly the H200, are essential for handling the resource demands of these AI models. Training an AI model capable of creating realistic portraits can require huge amounts of GPU memory, upwards of 40GB, to handle datasets with millions of images. Although these models are faster than traditional methods, generating a single, high-quality headshot can still take from 30 seconds to several minutes.
Interestingly, AI headshots are starting to incorporate real-time feedback loops, allowing users to tweak the generated images as they're being created. It's similar to interacting with other kinds of AI, but doing this requires the system to manage data effectively to avoid delays and ensure a good user experience. But as AI tools become more capable, questions surrounding the authenticity and ethical implications of AI-generated portraits will likely rise.
The ability of Nvidia GPUs to support parallel processing in AI-based portrait generation is an interesting capability. It means studios can handle bigger workloads without sacrificing speed or quality, giving them a potential advantage. It also brings up questions about the photography industry's changing dynamics. AI-driven automation could affect the role of traditional photographers in the field.
The growing use of AI for portrait creation in the cloud also raises the issue of data breaches. Implementing measures like encryption and controlled access becomes crucial for protecting personal data. In the future, we can expect even greater improvements in AI-based portrait generation. The newer generations of GPUs will likely enhance memory management techniques, potentially enabling ultra-high resolution images to be created in real-time. These developments could dramatically alter how we create and perceive digital portraits. It will be fascinating to see how this technology impacts the field of portraiture in the years to come.
This all suggests that we're in a period of rapid change in portrait generation, driven in part by advances in GPU technology. As AI becomes more sophisticated, the lines between traditional portrait photography and AI-generated ones will likely blur. The benefits of AI—speed, affordability, and customization—are undeniable, but also come with potential drawbacks and ethical questions that need consideration. It'll be interesting to observe the evolution of these technologies and how photographers and consumers respond to this changing landscape.
Create incredible AI portraits and headshots of yourself, your loved ones, dead relatives (or really anyone) in stunning 8K quality. (Get started for free)
More Posts from kahma.io: