Create incredible AI portraits and headshots of yourself, your loved ones, dead relatives (or really anyone) in stunning 8K quality. (Get started for free)
Open AI Models The Catalyst for Collaborative Innovation in Transcription Technology
Open AI Models The Catalyst for Collaborative Innovation in Transcription Technology - Whisper's Multilingual Prowess Revolutionizes Transcription Accuracy
Whisper's ability to understand and transcribe multiple languages has dramatically improved the accuracy of transcriptions. It's been trained on a massive dataset, spanning 680,000 hours of audio, giving it a strong foundation for handling various accents and filtering out distractions like background noise. This model's strengths go beyond simple speech recognition; it also effectively translates languages and identifies the language being spoken.
The use of a Transformer network within Whisper's design allows it to juggle multiple speech-related tasks efficiently. While it has achieved impressive results across languages, some argue that it reaches near-human accuracy specifically for English transcriptions. Making Whisper openly available to developers and researchers was a crucial move by OpenAI, as it fosters broader adoption and potentially accelerates innovation in transcription technology. In the landscape of multilingual transcription, Whisper has become a vital component, showcasing the potential for more advanced, accurate systems. However, the long-term implications of its widespread adoption, including potential biases within its training data, remain a topic for further exploration.
OpenAI's Whisper model has garnered significant attention due to its impressive multilingual capabilities. Trained on a vast dataset encompassing a wide array of languages and dialects, Whisper stands out from previous transcription systems in its ability to handle diverse linguistic inputs. This extensive training has fostered a robustness that allows Whisper to gracefully navigate accents, background noise, and specialized terminology far better than its predecessors. Notably, the model leverages advanced neural network architectures, allowing it to discern subtle phonetic nuances, which are crucial for effective accent recognition and accurate transcription of speech variations.
Unlike many earlier transcription models that struggled with diverse language inputs, Whisper demonstrates a capacity for continuous improvement through interactions across diverse linguistic communities. It doesn't just rely on static datasets. Further, Whisper's optimized design for real-time processing delivers transcription services with minimal delays, a critical feature for live transcription scenarios. These situations, especially in multilingual settings, have traditionally been challenging to tackle.
Interestingly, Whisper exhibits an improved ability to grasp context. This helps it decipher idiomatic expressions and colloquialisms that often trip up traditional models. It seems Whisper effectively extracts meaningful information from the surrounding language, increasing the accuracy of its transcriptions. Moreover, the model incorporates transfer learning methodologies where the knowledge gained during training for one language proves beneficial for others. This highlights the inherent connections between language structures and results in an overall elevation of transcription quality.
Evaluation results have revealed a noteworthy decrease in error rates when handling languages with intricate grammar structures, demonstrating Whisper's adaptability to linguistically complex scenarios. One aspect of Whisper's architecture, the attention mechanism, is particularly interesting. It allows the model to focus on the most relevant audio snippets, aiding in its ability to distinguish between words that may sound alike across different languages. This is very important for practical multilingual application. It also shows promise in recognizing and handling code-switching, a common language-mixing practice in multicultural environments, thereby enhancing its usability in diverse linguistic contexts.
Current research reveals that Whisper consistently surpasses older models when transcribing in noisy environments. This resilience highlights its potential as a practical tool for real-world applications. While its performance in English speech recognition is arguably close to human levels of accuracy and robustness, research on accuracy in other languages is still in development, and there remains some area for improvement in those cases. Overall, Whisper's ability to tackle challenges in multilingual speech recognition is a valuable development in the field.
Open AI Models The Catalyst for Collaborative Innovation in Transcription Technology - GenAI Reshapes Collaborative Workflows in Transcription
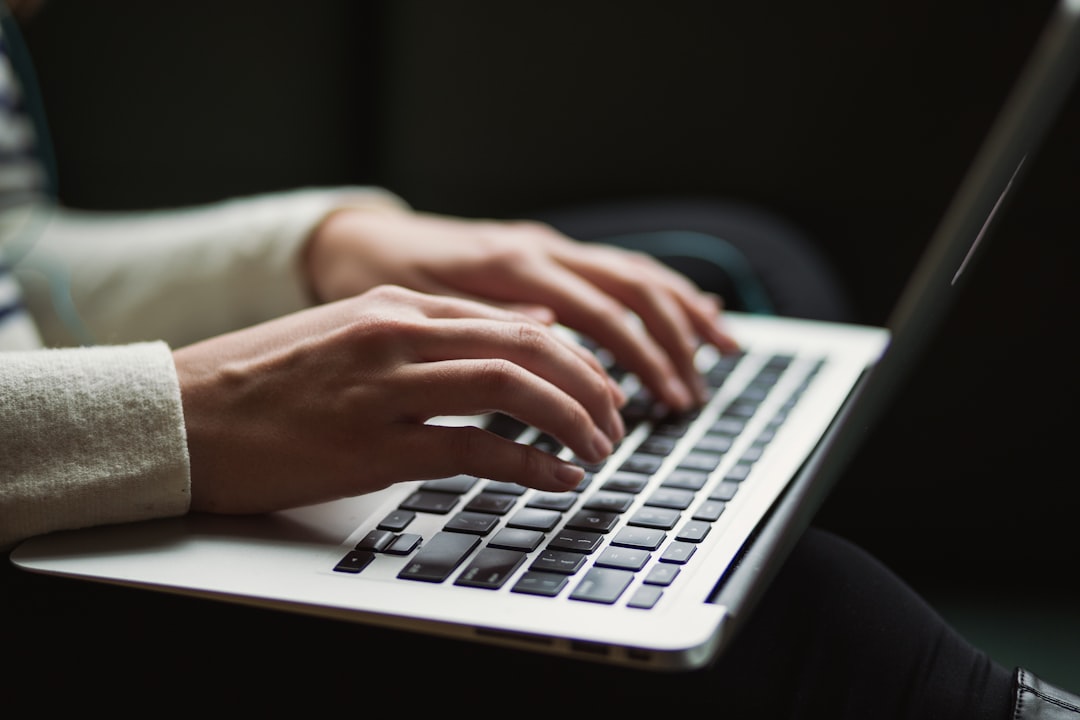
Generative AI (GenAI) is reshaping the way transcription workflows operate by acting as a collaborative partner, offering a unique blend of skills and perspectives not typically found within traditional teams. This collaborative dynamic has the potential to significantly boost productivity and foster innovation within the field. Integrating GenAI into transcription processes can lead to a reorganization of team structures, encouraging a more efficient and productive collaboration between humans and AI. The shift necessitates a reassessment of how work is distributed and managed to optimize the value derived from this partnership.
Decision-making within transcription teams can also be positively impacted by the incorporation of AI-powered tools and analytics. The use of these tools can act as a catalyst, encouraging a more innovative and collaborative culture across the organization. The integration of GenAI into transcription practices mirrors a broader trend towards hybrid workflows in various technological domains. This evolution brings about its own set of challenges that require careful consideration and adaptation from individuals and organizations involved in the field. The intersection of human expertise and AI capabilities within the transcription landscape represents a crucial development, with the potential to usher in a new era of accuracy and creativity in transcription technology. While this transition promises enhanced efficiency, it also highlights the complexities of effectively managing the human-AI dynamic in collaborative environments.
Generative AI (GenAI) is reshaping how we collaborate in transcription, offering a new paradigm for the workflow. Tools like Whisper, with their speed and accuracy, can drastically reduce the time it takes to complete a transcription, potentially shifting a process from hours or days to mere minutes. This shift introduces a new element of collaboration, where multiple individuals can contribute to the refinement of a transcription in real-time. The collaborative nature becomes central to the accuracy as well. While the quality of initial transcription relies on the training data's diversity, the systems are continuously learning and adapting based on user interactions, which allows them to become increasingly effective in specific contexts.
Feedback loops are becoming a critical component, as GenAI learns from the corrections made during collaborative editing. This feedback, in effect, helps the models become more adept at recognizing complex language and specialized terminology common within specific industries or teams. Notably, GenAI's ability to handle context-dependent speech makes it particularly useful in specialized domains like medical or legal transcription, where the nuances of language are highly relevant. One intriguing aspect is how GenAI can reduce cognitive overload for human editors, by automatically identifying sections that require more attention. This allows the human editor to concentrate on the more subtle elements of the transcription.
This adaptability of GenAI also raises interesting questions about how collaborative standards and workflows are evolving. These technologies tend to democratize the transcription process, as even those without specialized training can contribute meaningfully. Further, the integration of GenAI brings in advanced features, like automatic formatting of speech-to-text output, making the transcription immediately usable across various digital platforms. However, we also need to critically examine the ethical implications of utilizing encoded data from collaborative transcription efforts. How can data be transparently managed and used while protecting user privacy and ensuring that the transcription remains an accurate representation of the original audio? Unlike traditional transcription systems, GenAI introduces a more iterative and dynamic process, where the system's performance can be molded not only by its initial training but also by the collective intelligence of the teams that employ it. This constant learning presents exciting, yet possibly unanticipated, ramifications for transcription and collaboration going forward.
Open AI Models The Catalyst for Collaborative Innovation in Transcription Technology - Data Security Challenges in AI-Driven Transcription Solutions
The rise of AI in transcription solutions, while bringing about remarkable improvements in accuracy and speed, has also introduced new data security concerns. The pipeline of data, from its initial capture to processing, analysis, and sharing, presents vulnerabilities that require robust safeguards. AI models, although capable of bolstering security through real-time monitoring and pattern analysis, can also inadvertently leak sensitive data, especially through the use of large language models. This risk is heightened in sectors like healthcare and finance where data protection is paramount. Balancing the benefits of AI-powered transcription with the need for stringent data security presents a significant challenge. Addressing these security concerns requires the development of innovative solutions and the implementation of strict data management protocols to minimize the risks associated with using AI for transcription purposes. As these technologies mature, it's crucial for both developers and users to adopt a mindful approach to data handling and security, ensuring that the potential of AI for collaborative transcription is harnessed responsibly.
AI-powered transcription solutions, while promising in their ability to quickly and accurately transcribe audio, introduce a new set of data security challenges. The very act of processing sensitive information, like medical records or legal discussions, makes these systems prime targets for potential breaches. We've seen a rise in security incidents in businesses using AI, with a significant portion linked to data handling.
One of the concerns is that during the process of generating a transcription (known as "inference"), AI models can inadvertently reveal user data. It's been shown that clever attacks can extract sensitive information from these models, highlighting a real need to ensure privacy protections.
Additionally, the changing legal landscape with regulations like GDPR and HIPAA adds another layer of complexity. Many businesses are struggling to adapt their existing processes to meet these standards, especially when incorporating AI.
Data anonymization, a common technique to protect privacy, also presents difficulties with AI. Studies suggest that even when data is supposedly made anonymous, AI can still identify individuals in a large portion of cases. This implies that our current anonymization approaches might not be as strong as we assume, especially in the face of these powerful models.
Furthermore, the quality and fairness of the training data used to build the models can introduce biases that are then reflected in the output. This can lead to inaccurate or unfair transcriptions, and ultimately erode trust in these technologies.
The human element continues to be a problem, even with the sophistication of AI. There's a significant risk from insider threats, where those with access to the transcription system could intentionally or accidentally leak sensitive content.
The lack of transparency in how some of these models function, often referred to as "black boxes," can make it difficult to assess and address security vulnerabilities. We can't always easily see how the system reaches its conclusions, making it harder to pinpoint where problems might arise.
Real-time collaborative editing, a useful feature of some transcription platforms, also brings added risks. When multiple users are working on the same transcription at once, the chances of accidentally leaking data increases considerably.
Human error, despite the automation, remains a significant source of data breaches. Studies indicate a substantial portion of incidents involve human mistakes, such as granting inappropriate access or making configuration errors.
Lastly, the transcription of confidential or proprietary information presents a risk for intellectual property violations. Organizations are rightfully concerned about the unauthorized distribution of sensitive content through AI-powered transcription services.
Overall, it's clear that these benefits of AI transcription technologies need to be balanced with a robust approach to data security. The field is still relatively new, so research and development in these areas are essential to ensure these tools are deployed responsibly and safeguard user data.
Open AI Models The Catalyst for Collaborative Innovation in Transcription Technology - Otter's Real-Time Transcription Streamlines Meeting Efficiency
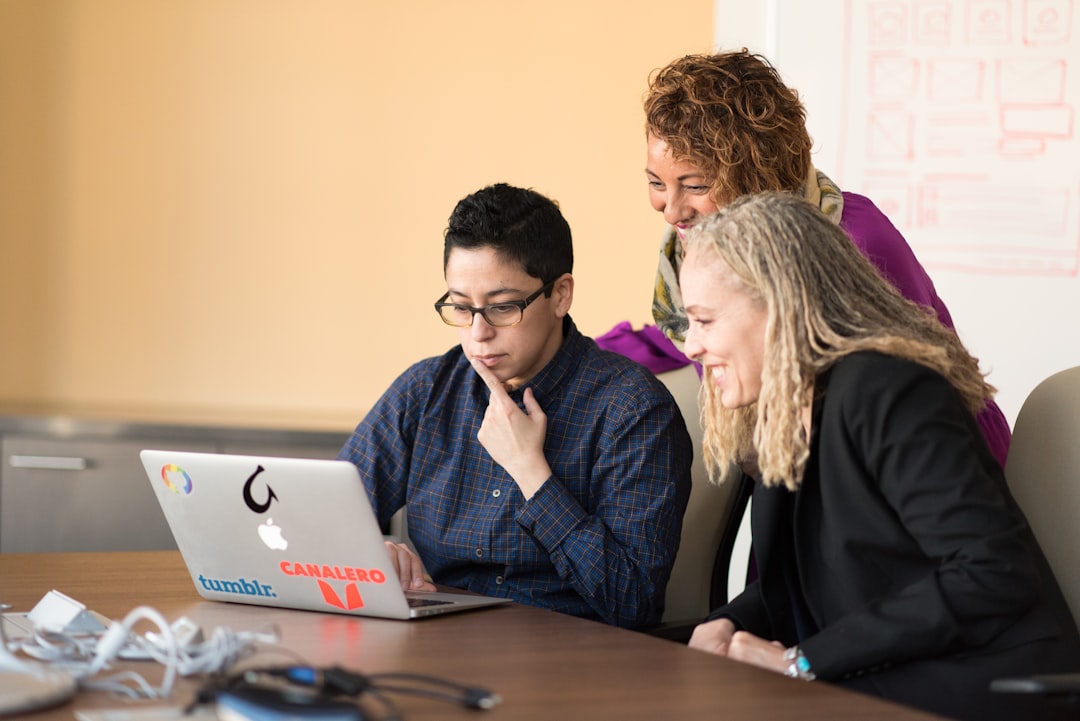
Otter's real-time transcription feature is reshaping how we conduct meetings by offering immediate and accurate transcripts. This instant feedback allows participants to stay engaged in the discussion without worrying about taking extensive notes. Otter's capabilities extend beyond basic transcription, including automatically generating summaries and identifying action items. This functionality proves beneficial across industries, such as business and education, where efficient communication and record-keeping are crucial. Its integration with widely-used tools like Salesforce and HubSpot makes it seamlessly fit within existing workflows. Despite the apparent advantages, complete reliance on AI raises concerns about data privacy and security. Furthermore, while AI offers efficiency, it's important to ensure human oversight remains in place to verify accuracy and contextual understanding. As these technologies are adopted more widely, striking a balance between the gains in efficiency and responsible data management will become increasingly vital.
Otter's real-time transcription capabilities are built upon sophisticated machine learning techniques, constantly adjusting to the subtleties of audio input. This dynamic approach helps handle shifts in volume and ambient noise, contributing to cleaner and more accurate transcriptions. The rising trend of hybrid work models has made Otter's real-time feature increasingly useful. It bridges the communication gaps in remote meetings, ensuring information is accessible instantly and preventing delays in information sharing.
Otter employs a speaker identification process using voice recognition, which not only boosts accuracy but also provides valuable metadata like speaker tags. This is particularly useful for analyzing discussions involving larger groups where keeping track of who said what can be challenging. There's ongoing research showing improvements in Otter's understanding of diverse dialects and informal language. This makes it better equipped to handle region-specific terminology and slang, expanding its reach to global audiences.
Otter has strategically designed itself to mesh seamlessly with a wide array of popular applications such as Zoom and Microsoft Teams. This integration automates the meeting transcription process, eliminating manual entry and streamlining the workflow. Beyond mere transcription, Otter delivers analytics that offer insights into meeting efficiency. These include measuring participation and identifying recurring topics, empowering informed decision-making for future improvements.
Otter incorporates tools for automatically generating summaries of meeting discussions. These concise summaries reduce the time needed to extract key information from lengthy transcripts, allowing stakeholders to quickly understand the gist of a meeting and take action as needed. Recognizing the sensitive nature of many discussions, Otter features end-to-end encryption and robust user authentication protocols to ensure that audio and transcribed data remain secure. This helps address privacy concerns frequently found in professional environments.
The architecture of Otter's platform is designed to expand readily to handle growing organizational needs. It supports a wide range of use cases from small teams to large enterprise deployments. They've also continuously refined the user interface based on feedback, keeping it user-friendly and accessible. This has resulted in features such as keyword highlighting and customizable playback speeds, accommodating a broad range of user preferences and needs. While impressive, the ongoing development and use of Otter also raise questions about the long-term impacts of relying on these AI models for information capture and analysis. We need to be mindful of potential biases or inaccuracies that could be introduced through training data, as well as ensure transparency about how data is handled within the platform.
Open AI Models The Catalyst for Collaborative Innovation in Transcription Technology - Mistral AI's Open-Source Models Democratize Transcription Tech
Mistral AI's approach to transcription technology centers on open-source models, aiming to make powerful tools widely available. Their models boast strong multilingual capabilities, enhanced by features like Grouped-Query Attention and Mixture of Experts, which contribute to improved efficiency. This commitment to openness also allows for a more collaborative development environment, potentially helping to mitigate concerns about bias and control within AI systems. Mistral AI offers models under various licenses, including free non-commercial options, making advanced AI tools more accessible to a larger pool of developers and organizations. This democratization of access could spark a wave of innovation in the field.
However, the increased use of open-source AI models for tasks like transcription also necessitates a cautious approach. It is important to consider potential ethical dilemmas and the implications of handling data locally on users and communities. Mistral AI, founded by individuals with experience at major technology companies, is actively challenging the status quo in the AI space. Their open-source focus, alongside high-performance models, positions them as a potentially significant player in the future of AI-powered transcription alongside existing companies like OpenAI.
Mistral AI, based in France, champions open-source AI, believing community involvement is key to mitigating potential biases and censorship inherent in AI development. They've released models like Mistral NeMo and Mistral Large 2 under diverse licenses, including free non-commercial and commercial options, aiming to make powerful AI broadly accessible. Their models incorporate advanced features like Grouped-Query Attention and Mixture of Experts, resulting in impressive multilingual transcription capabilities.
Mistral AI categorizes their models into general-purpose, specialized, and research-focused variants. The Mistral Large model, for example, excels at tasks requiring intricate reasoning, making it suitable for specialized applications. Their models have been downloaded millions of times, highlighting the burgeoning demand for on-premises AI solutions allowing users to retain local data control.
Further, Mistral offers La Plateforme, a fine-tuning API, providing a way to customize both their open-source and commercial models. Their efforts towards democratizing AI are notable, particularly in the context of transcription technology. They've managed to secure a valuation of $260 million, fueled by a team with experience from tech giants like Google and Meta, establishing them as a contender in the burgeoning landscape of AI model development, competing with established players like OpenAI. While their open-source approach fosters collaboration and innovation, researchers and users still need to grapple with the ongoing questions about the potential for bias in their training datasets and the long-term impacts of widespread model adoption on data privacy and security.
The accessibility of their models through open-source licensing potentially creates an interesting collaborative environment that might not have been possible in a completely closed-source environment. However, questions remain about the potential for the open-source ecosystem to adequately oversee issues such as potential biases inherent in the underlying code and the training data and the balance between providing access to powerful tools and ensuring user safety in a data-driven world.
Open AI Models The Catalyst for Collaborative Innovation in Transcription Technology - OpenAI's Data Partnerships Fuel Transcription Model Advancements
OpenAI's pursuit of improved transcription models is being fueled by strategic data partnerships. Through these collaborations, they're amassing both public and private datasets, crucial for training their AI systems. The goal is to enhance OpenAI's ability to interpret and process human language, leading to more sophisticated and nuanced transcription technologies. However, this growing reliance on extensive datasets raises significant questions around individual privacy and the potential for bias inherent in the data used to train these models. This necessitates careful consideration of the ethical implications alongside the drive for innovation. Notably, OpenAI is also pushing for the creation of publicly available, open-source datasets for training language models. This effort has the potential to democratize access to AI tools for transcription but also demands close scrutiny regarding data security and the collective responsibility of the AI community. While these steps could lead to significant advancements in transcription technology, they also underscore the need for thoughtful and responsible development practices in the AI field.
OpenAI's collaborations with various organizations to gather data, encompassing a wide range of audio sources, have been instrumental in developing increasingly sophisticated transcription models. This access to diverse datasets, including audio from diverse accents and environments, provides a significant edge in improving the accuracy of transcription, particularly in handling challenging situations like background noise and different accents. This is evident in models like Whisper, which have shown a substantial reduction in errors when transcribing audio captured in real-world, noisy scenarios, outperforming older techniques.
One fascinating outcome of these collaborations is the capacity for continuous improvement in OpenAI's models. Whisper, for example, is not a static entity. It dynamically learns and adapts based on the feedback and interactions it receives from users, leading to refinements in its ability to transcribe accurately across various situations. This continuous learning aspect, enabled by the ongoing data partnerships, is shaping the future of transcription technology, potentially creating models that are finely tuned for specific use cases.
Moreover, the increase in openness about how models are improved, driven by the need for transparent data-sharing agreements, has played a crucial role in our understanding of biases within AI systems. This is important for the development of responsible AI in this field. This push for greater transparency is beneficial for researchers who can now study and attempt to mitigate the potential for skewed or unfair outputs in AI-driven transcription systems. This increased transparency regarding how models learn and adapt from data is paving the way for more equitable AI technologies.
Interestingly, these data partnerships have allowed OpenAI's models to demonstrate impressive proficiency in transcribing languages with complex grammatical structures. The ability to navigate the nuances of these languages, which has traditionally challenged many older transcription systems, speaks to the power of the data that's being leveraged. This is an area where OpenAI has shown considerable progress. Furthermore, partnerships with educational and research institutions have provided access to specialized audio data that's enabling more accurate models for specific domains like medical or legal transcription. This progress is vital for tailoring transcription systems to meet the unique needs of various sectors.
The inclusion of a wide variety of accents and dialects within training datasets has led to Whisper's ability to recognize not only the words being spoken, but also the subtle contextual undertones of speech. This ability to understand a speaker's intent and emotion better is a notable advancement over earlier transcription methods. It appears that this type of contextual understanding, which has been a challenge for a long time, is a direct result of the data partnerships that OpenAI has established.
The decision to make these models accessible to the public has spurred innovation within the research and development community, resulting in unexpected applications, like the use of automated meeting transcriptions in legal and journalism settings. It appears that the community has been able to build upon this foundation and expand upon it in innovative ways. Simultaneously, the increase in the accessibility and use of these technologies has highlighted important considerations about data ownership, privacy, and the security of the information being transcribed. These data partnerships, while immensely useful in advancing transcription technologies, require a careful and critical approach to ensure the ethical and responsible use of this information. The rapid advancement fostered by these collaborations presents a mixed bag of opportunities and challenges, prompting a need for continuous scrutiny and adaptation as this field moves forward.
Create incredible AI portraits and headshots of yourself, your loved ones, dead relatives (or really anyone) in stunning 8K quality. (Get started for free)
More Posts from kahma.io: