Create incredible AI portraits and headshots of yourself, your loved ones, dead relatives (or really anyone) in stunning 8K quality. (Get started for free)
The Real Cost of AI Headshots vs
Professional Photography A 2025 Price Analysis
The Real Cost of AI Headshots vs
Professional Photography A 2025 Price Analysis - Direct Cost Breakdown Studio vs AI Platform Prices 2025
As of May 2025, looking at the direct price tags for getting headshots via AI platforms versus going to a professional photo studio shows some key differences in how money is spent. For organizations exploring AI solutions, setting up or accessing a capable system can involve an initial cost potentially starting around twenty thousand dollars, which can increase significantly depending on the level of customization or specific output requirements. In contrast, professional photography typically offers pricing that might seem more straightforward upfront, though the actual expense can still vary quite a bit depending on the photographer's skill level and the specifics of the shoot commissioned. Beyond these immediate, clear costs, the AI route might also carry less visible expenses down the line related to system maintenance or ensuring it fits correctly with existing operations. Making a truly informed decision requires looking past just the initial figures to understand the full financial picture for each approach.
Examining the raw financial outlay for obtaining a professional headshot in mid-2025 reveals a distinct divergence in structures between traditional studios and AI-driven platforms. On the user end, the immediate transaction cost for an AI-generated image is often remarkably low, sometimes merely tens of dollars per output. This stands in stark contrast to securing a session with a human photographer, which typically requires an upfront investment ranging from the lower to mid-hundreds of dollars, covering the photographer's time, expertise, and operational costs like equipment and space, potentially running a photographer's setup into five figures. The AI platforms, while offering these cheap per-image rates or subscription tiers (the latter introducing variable long-term costs for frequent users), are underpinned by significantly higher foundational expenditures; developing and maintaining the sophisticated AI models and infrastructure requires substantial capital, with estimates for such systems potentially reaching into the $100,000-$200,000 range or more for complex implementations, a cost structure vastly different from a traditional studio's overhead.
From an engineering standpoint, the low user cost of AI reflects the efficiency of distributing a high initial development cost over a massive number of users and generated images. The user is paying for compute time and algorithm access rather than dedicated human creative labor per output. This model facilitates rapid turnaround, often seconds versus the days or weeks traditional editing workflows might require. However, focusing purely on the lowest per-image price point overlooks potential trade-offs. While AI quality has advanced impressively, blurring the line for many users and raising questions about authenticity, the nuanced capture of expression or the subtle artistic interpretation a skilled human photographer can bring is often cited as lacking. Furthermore, the prevalent subscription models of AI platforms, while seemingly convenient, can accumulate into a significant total expense over time compared to the finite cost of a single photography session package. The disruption this creates in the market is evident, leading to discussions among photographers about decreased demand and prompting some studios to explore hybrid models incorporating AI tools to remain competitive on price or efficiency. Understanding the direct cost, therefore, necessitates looking beyond the sticker price to the underlying service model, the foundational technology investment, and the inherent value (or potential lack thereof) delivered.
The Real Cost of AI Headshots vs
Professional Photography A 2025 Price Analysis - Technical Requirements Mac vs Cloud Processing for Business Headshots
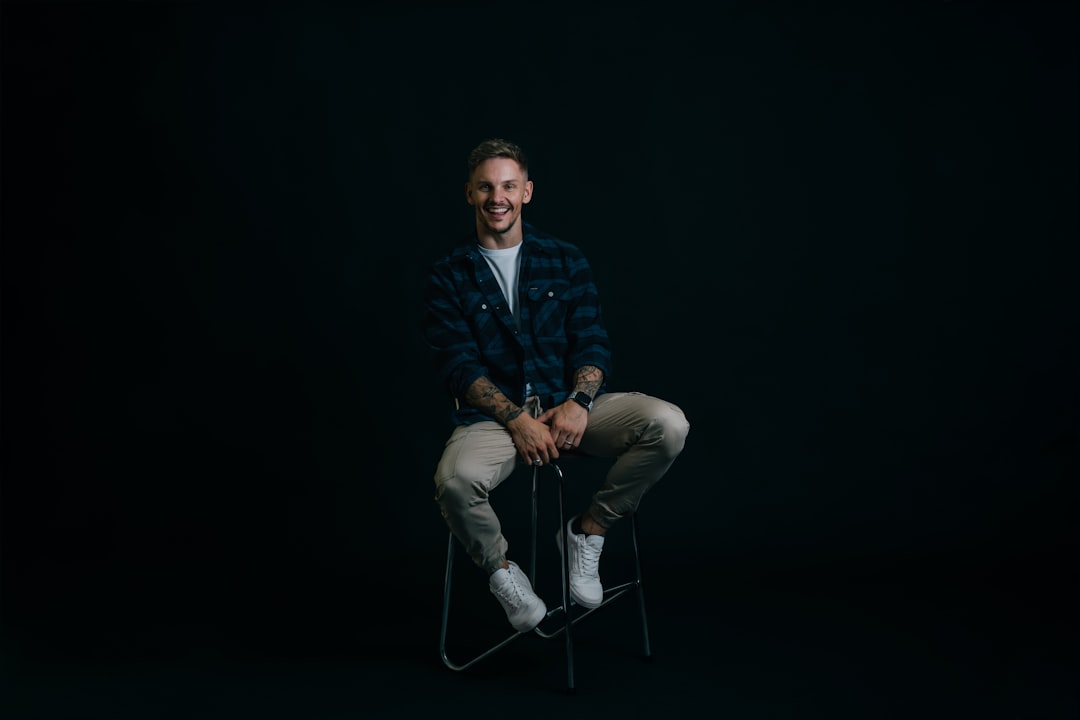
As of mid-2025, the technical considerations for creating business headshots involve navigating between relying on dedicated local computing power, often associated with systems like Macs, and leveraging the distributed capabilities of cloud processing. Using powerful local hardware means investing in robust machines and specialized software, allowing for detailed control over the image editing process. This approach puts the retoucher directly in charge of subtle manipulations, though it ties productivity to the capacity and availability of individual workstations and the time needed for manual adjustments.
Cloud processing, on the other hand, represents a shift towards using remote server farms to handle computationally intensive tasks. This is the engine behind many AI-driven image generation services. It offers the potential for rapid results and processing large volumes without burdening individual users' machines. However, this technical shift often comes with trade-offs in direct control and fine-grained customization. The output depends on predefined algorithms and processing parameters, which might deliver efficient results but potentially struggle to capture truly unique expressions or apply the nuanced artistic judgment a human might exercise. Ultimately, the choice hinges on balancing the technical pathway's inherent control limitations against its potential for speed and scale when producing professional images.
Examining the technical underpinnings of generating headshots, whether through computational processes or human-led photographic sessions, reveals fundamentally different resource demands and workflows.
The engine powering AI headshot generation typically requires substantial computational muscle. This often means accessing powerful, often cloud-based, server arrays equipped with multiple high-performance GPUs to crunch the data and run complex generative algorithms. In stark contrast, the technical requirements for a traditional photography session lean heavily on optical equipment, lighting setups, and the human operator's learned expertise in manipulating these tools. There isn't a direct need for this level of digital processing horsepower during the capture phase itself.
Concerning throughput, AI platforms are designed for rapid, parallel processing. Once the input images are uploaded and the parameters set, a series of potential outputs can be rendered remarkably quickly, sometimes in mere seconds. The traditional photographic workflow, however, is inherently sequential and involves a more significant elapsed time. It progresses from physical capture, through selection, then often requires post-processing steps like digital editing and retouching, which, depending on complexity and workload, can introduce delays measured in days or even weeks before final delivery.
From a data output perspective, the typical delivery format differs. Professional photographers, particularly when commissioned for business or specific purposes, often provide images in less-compressed formats such as TIFF or even original RAW files. These formats retain a much richer dataset, allowing for extensive manipulation or ensuring quality for large-format printing. AI-generated outputs, while convenient for digital distribution, are commonly delivered in highly compressed formats. This speeds up transmission and reduces storage burden but can potentially sacrifice fine detail and introduce artifacts, limiting flexibility for subsequent use.
Regarding flexibility in image creation, AI systems currently operate within parameters defined by their training data and interface options. While offering variations, the range of creative control is generally bounded by pre-set templates or algorithmic constraints. A human photographer, conversely, possesses the technical and artistic agency to adjust lighting setups dynamically, experiment with angles, guide subtle expressions, and react in real-time to the subject and environment, enabling a much higher degree of bespoke tailoring for a truly unique result.
The quality curve for AI output appears highly dependent on the sophistication and specific training of the underlying models. Results can be inconsistent, sometimes producing uncanny artifacts or failing to accurately represent the subject's likeness or desired mood. Human photography, while also subject to variability, is influenced by the photographer's cumulative experience, technical mastery of their equipment, and an intuitive understanding of light, composition, and human interaction refined over time. The peaks of human-driven quality can still surpass automated generation in capturing authentic presence.
Considering the total cost of ownership from a technical angle, AI platforms necessitate ongoing investment in infrastructure maintenance, software updates, and algorithm refinement to stay current and functional. These represent recurring operational costs. A professional photographer's technical costs, beyond initial equipment acquisition, primarily relate to depreciation, occasional calibration, and potential upgrades, often more predictable and less subject to continuous software development cycles.
The capacity of AI systems to produce a high volume of images with a consistent aesthetic profile is a direct consequence of algorithmic processing. However, this very consistency can lead to outputs that, while technically proficient, may feel uniform or lack the subtle nuances of genuine human emotion or unique individual expression that a skilled human can perceive and capture through interaction and timing.
The process of acquiring a headshot through traditional photography involves direct communication and collaboration. The subject receives guidance on posing, expression, and posture, and there's typically a feedback loop allowing adjustments during the session or post-session editing. AI workflows lack this interactive component. The technical pipeline receives input and outputs results without the ability for real-time, human-to-human communication that can shape the final product based on subtle personal preferences or reactions.
The availability of relatively low-cost AI headshot solutions has introduced a technical challenge for traditional photographers. It prompts many to explore integrating AI tools into their own workflows, perhaps for preliminary culling, basic edits, or even stylistic processing, seeking to leverage computational speed to remain competitive on turnaround time or potentially offer different service tiers.
From a longer-term perspective, while the immediate transactional cost for AI headshots can be minimal, accessing these services often involves recurring subscription fees or per-image costs that can accumulate. This contrasts with the technical cost model of professional photography, which is typically a finite, project-based expense once the session and delivery are complete, providing a durable set of assets whose value is tied to their inherent quality and potential for diverse future applications, rather than ongoing service access fees.
The Real Cost of AI Headshots vs
Professional Photography A 2025 Price Analysis - Turnaround Time Study 15 Minutes AI Generation vs 48 Hour Photography
The examination of turnaround time presents a significant difference between the rapid production of AI-generated images and the more involved process of professional photography. AI systems can generate outputs in as little as fifteen minutes, offering an almost instant result compared to the forty-eight hours a traditional photographer typically requires for post-processing and delivery. This speed is a clear draw for users needing swift visual assets, but it prompts a necessary reflection on the nature of what is being produced. While highly efficient, the automated process inherent in AI generation may struggle to imbue images with the subtle emotional context, genuine expression, or artistic interpretation that a human photographer, through interaction and skilled judgment, can capture. The notable speed differential poses a challenge to conventional photographic practices, forcing a re-evaluation of what constitutes value in a headshot when speed is placed in direct opposition to potentially deeper qualitative elements.
Moving from the raw technical requirements, we observe a stark difference in the operational cadence between algorithmic image generation and traditional human-driven photography, particularly when focusing on the sheer speed of output. AI platforms, leveraging their underlying computational architecture, can deliver potential headshot images remarkably fast, often within a mere fifteen minutes from input to preliminary results. This stands in sharp contrast to the workflow of professional photography, which typically involves scheduling, shooting, selecting, and a significant post-processing phase that can easily stretch over forty-eight hours or substantially longer, depending on the workload and complexity.
This speed difference is directly tied to the capacity for simultaneous processing. An AI system, once initiated, can theoretically churn out a high volume of variations concurrently, drawing on distributed computing resources to manage throughput at scale. A human photographer's capacity is inherently limited by the sequential nature of interacting with a subject, setting up equipment, and the time required for focused, individual post-processing attention per image – typically yielding a handful of select final images per session, not thousands.
From a technical quality standpoint, the rapid generation process in AI can sometimes manifest as variability in the final output. While sophisticated models exist, the algorithmic process doesn't always guarantee consistency, occasionally producing subtle, unnatural features or failing to capture likeness with high fidelity across all outputs. This can contrast with the more predictable quality level achievable by a seasoned human photographer who applies learned techniques and aesthetic judgment consistently.
The economic model underpinning this speed and scale involves significant upfront technical investment in the necessary infrastructure and ongoing maintenance for complex AI models. Estimates for establishing or licensing high-quality generative systems can run into the low six figures or beyond. A professional photographer's technical costs, while substantial for equipment, represent a different investment profile, focused on tools for capture and refinement with depreciation and occasional upgrades being the primary long-term considerations, rather than massive computational infrastructure.
The process itself fundamentally impacts creative control and the outcome. AI-generated images operate within the bounds of their training data and predefined parameters, offering variations but potentially lacking the subtle, unique tailoring a human photographer can bring. A human can make real-time adjustments to lighting, posing, and capture timing based on nuanced observation of the subject and the desired mood. The collaborative feedback loop present in a human session, allowing adjustments mid-shoot, is absent in the AI pipeline.
Furthermore, the technical delivery methods often reflect this operational difference. To facilitate rapid distribution and manage storage, AI outputs are frequently provided in compressed formats, a technical decision that prioritizes speed and efficiency but can potentially sacrifice the fine detail and flexibility retained in the less-compressed or RAW files often delivered by professional photographers, which are intended for more demanding applications.
While the immediate transactional cost per image might appear minimal for AI outputs, the service delivery often involves recurring subscription fees or cumulative per-image costs that can, over time, potentially exceed the finite, project-based cost of a professional photography session, particularly when factoring in the potential need for multiple AI batches to achieve a satisfactory result due to quality variability or lack of fine control.
Ultimately, the difference in turnaround time highlights the divergence in technical approach and its impact on process, output characteristics, and economic structure. The algorithmic speed prioritizes volume and rapid accessibility, while the human-led process emphasizes control, consistency derived from experience, and nuanced creative tailoring through interaction. This technological bifurcation continues to prompt shifts in the market, challenging existing service models and workflows within the visual production landscape.
The Real Cost of AI Headshots vs
Professional Photography A 2025 Price Analysis - Quality Comparison Natural Lighting vs Digital Lighting Generation
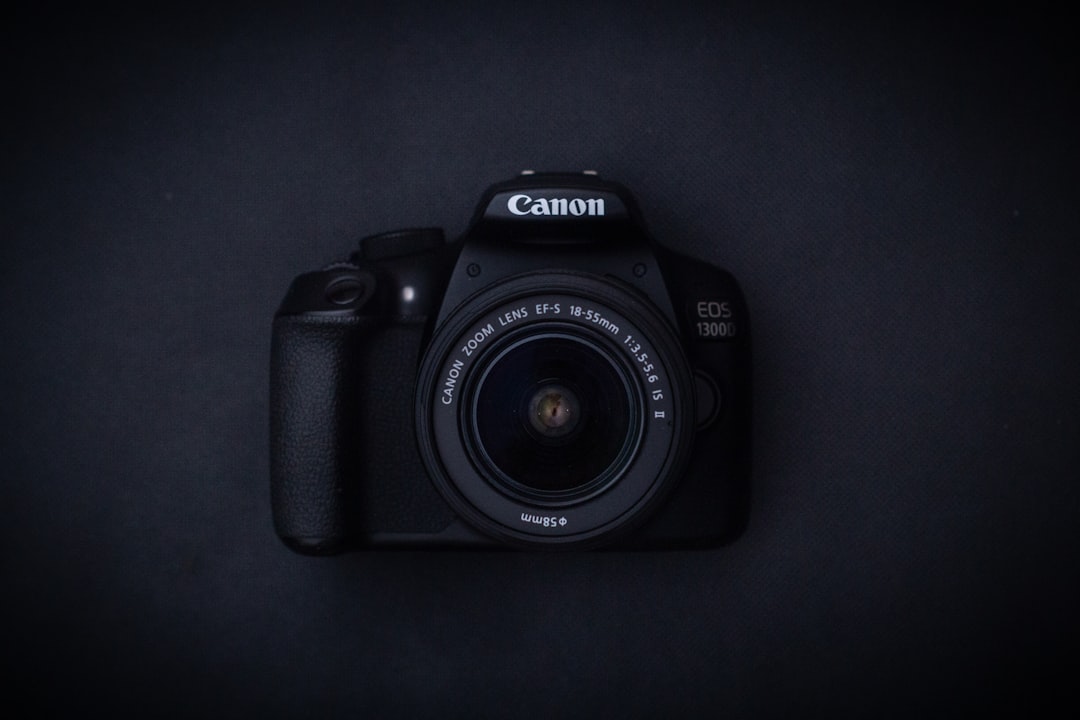
When considering the quality differences arising from how light is used in photography, especially for portraits or headshots, the contrast between utilizing natural light and generating light digitally or artificially is quite pronounced. Natural illumination is frequently appreciated for its capacity to imbue images with a sense of authenticity and a broad spectrum of moods and tones, varying beautifully throughout the day and often rendering subjects with a flattering, soft quality. It also benefits from being freely available, avoiding the expense of specialized equipment or ongoing power consumption. Yet, its reliance on environmental factors means consistency can be elusive, and shooting is often dictated by external conditions and specific times.
Conversely, employing artificial lighting grants photographers a high degree of deliberate control over the visual outcome. This method allows for tailoring light precisely, enabling the creation of specific, repeatable effects and ensuring uniformity regardless of the time or weather. The ability to meticulously manage highlights, shadows, and reflections is a key strength. However, building out a capable artificial lighting setup requires a notable financial commitment for the necessary gear, which can be a significant consideration compared to the zero direct cost of using natural light. Ultimately, the choice between these approaches for quality depends on the desired aesthetic effect, the need for consistency versus accepting variability, and the practical constraints and budget of the production.
1. Examining the output, natural illumination displays inherent spatial and temporal variations, producing dynamic qualities. Engineered digital lighting setups, conversely, often aim for control and consistency via fixed parameters, which can result in a visually more uniform look lacking some of that spontaneous variance.
2. Analyzing color rendition, images captured with natural light sources, possessing a broad spectrum, generally appear to exhibit greater accuracy in hue representation compared to those generated or photographed under artificial illumination sources with potentially narrower or segmented spectra.
3. Observing shadow formation, natural light, often interacting with a wider environment, tends to create softer, more diffuse gradients and transitions. In contrast, more directional or localized digital lighting sources, if not carefully shaped, can generate harsher, more defined shadows that may be less aesthetically pleasing in portraiture.
4. Regarding the luminance range captured, photography utilizing ambient natural light frequently records a broader span of detail in both bright highlights and deep shadow regions. Artificial or digital lighting, if not balanced appropriately, can sometimes lead to quicker 'clipping', where detail is lost in the extremes of the tonal scale.
5. The distinctive visual properties linked to specific times of day – such as the low angle and warm tones of the "golden hour" – are unique phenomena of natural light. Digital or algorithmic lighting models, lacking a real-world temporal dimension, must explicitly simulate these complex conditions, which can be technically challenging to replicate authentically.
6. Achieving optimal results with natural light involves a photographer's real-time assessment and subtle adjustments to subject positioning and environmental factors. Automated or AI-driven lighting applications, operating on predefined algorithms, lack this instantaneous, intuitive response to the nuances of human expression or physical arrangement.
7. The interaction of natural light across surfaces can enhance the perception of texture and contribute to a sense of depth and volume. Simplistic or inadequately modeled digital illumination may sometimes reduce this effect, rendering forms with less tangible surface quality.
8. Images derived from well-utilized natural lighting often possess a foundational aesthetic quality that may demand less extensive post-processing manipulation to achieve a final desired look compared to images generated with less sophisticated or less tailored artificial lighting.
9. The process of utilizing natural light in photography inherently involves a dynamic interplay where light quality informs real-time decisions about composition and subject interaction. Applying digital lighting often occurs as a step removed from the physical presence and moment, limiting this direct, interactive feedback loop.
10. The technical effort and resources required to construct artificial lighting environments capable of mimicking the diverse qualities and complexities of natural light scenarios can be considerable, involving specialized hardware or advanced digital modeling, a non-trivial task distinct from merely leveraging available ambient light.
The Real Cost of AI Headshots vs
Professional Photography A 2025 Price Analysis - Hidden Expenses Travel Costs vs Server Time Charges
Beyond the clear price tags for AI headshots or a photography session, a layer of less visible expenses significantly impacts the true cost, particularly for automated systems. These hidden AI costs stem heavily from the necessary technical infrastructure, involving substantial and often underestimated cloud service fees, including charges for data movement or underutilized processing power. There are also persistent costs tied to maintaining complex AI models, preparing and managing the vast datasets they require, and the energy consumption inherent in intensive computing, sometimes raising environmental considerations. Unlike the relatively upfront or project-defined costs of traditional photography – which might include travel or specific setup fees but are generally factored into the initial agreement – the operational expenses behind AI can accumulate less predictably, sometimes leading businesses to face surprisingly high bills and unforeseen budget challenges as usage scales or technical demands evolve. Understanding these ongoing and hidden infrastructure costs is critical when assessing the long-term financial reality compared to the more bounded expense of a dedicated human photographer.
Beyond the readily apparent price tags, engaging with AI systems for tasks like generating headshots introduces a stratum of costs that are less visible but financially significant. From an engineering perspective, these costs are intrinsically linked to the computational infrastructure and algorithmic processes underpinning the service.
1. The fundamental financial architecture for AI computation often relies on complex, pay-per-use models in cloud environments. Charges aren't a simple flat rate but tied to granular consumption metrics – CPU cycles, GPU time, memory allocation, network bandwidth. This leads to variable and sometimes unpredictable expenses, especially as usage patterns fluctuate or spike.
2. A substantial sunk cost lies in the development and training of the generative models themselves. This requires immense computational resources and engineering effort over prolonged periods, processing vast datasets. While amortized across users, this foundational investment influences the overall service pricing structure.
3. Ongoing technical operations like model monitoring for performance drift, periodic retraining with new data, software dependency management, and infrastructure scaling to meet demand represent continuous operational expenses necessary to keep the platform viable.
4. The process of preparing input data for AI models—curation, cleaning, formatting—is often a non-trivial technical task with associated computational costs, particularly when dealing with large volumes or diverse sources of images.
5. Achieving a satisfactory outcome with AI generation may require multiple iterations due to algorithmic variability or the need to explore different parameters. Each generation attempt consumes compute resources and contributes to accumulating costs, turning a seemingly cheap process into a more expensive one through repeated trials.
6. Demanding higher resolution outputs or processing larger batches of inputs simultaneously increases the computational load proportionally. This scales the required server resources and directly translates into higher charges under usage-based billing models.
7. Storing input data, intermediate files generated during processing, and the multiple variations of output images requires persistent storage, typically in the cloud. These storage costs, often billed per gigabyte per month, add a layer of expense that grows with data volume and retention policies.
8. Accessing the AI models and transferring image data between the user and the cloud infrastructure incurs network egress fees and API call charges, adding transactional costs that can become significant at scale.
9. Troubleshooting issues with AI outputs, understanding why an image didn't meet expectations, or navigating complex platform interfaces can consume user time and potentially incur further compute cycles for regenerating images, effectively adding a 'debugging' cost.
10. Accurately predicting the total financial outlay for using AI services over time is technically challenging. It requires forecasting usage patterns, understanding opaque billing metrics, and accounting for potential variability in outcomes that necessitate costly re-runs, making precise budgeting difficult.
Create incredible AI portraits and headshots of yourself, your loved ones, dead relatives (or really anyone) in stunning 8K quality. (Get started for free)
More Posts from kahma.io: