Create incredible AI portraits and headshots of yourself, your loved ones, dead relatives (or really anyone) in stunning 8K quality. (Get started for free)
7 AI-Powered Techniques to Enhance Letterhead Images for Professional Photography Portfolios
7 AI-Powered Techniques to Enhance Letterhead Images for Professional Photography Portfolios - AI-Powered Resolution Upscaling for Letterhead Images
AI-powered resolution upscaling has become a valuable tool for photographers creating professional portfolios, especially when it comes to letterhead images. These AI tools can take lower resolution images and intelligently boost their resolution, leading to sharper and more detailed versions. This improved clarity is essential for achieving professional-quality prints and digital displays.
Photographers are given the flexibility to use different file formats like JPEG and PNG when using these AI tools. Additionally, users have control over the level of upscaling, with typical options being 2x or 4x the original size. The upscaling process itself has advanced considerably with sophisticated algorithms that focus on adding pixels in a way that preserves the image details. This is particularly important for brand consistency and avoiding a loss of the original visual elements. Furthermore, the convenience factor is enhanced because some tools operate locally, eliminating the need for an internet connection during the upscaling process. This aspect is important for photographers working on projects where connectivity may be a concern.
AI upscaling leverages deep learning methods like convolutional neural networks (CNNs) to reconstruct images at higher resolutions. These models learn from massive datasets of images, identifying patterns to add detail that traditional techniques often miss. Some models can achieve impressive increases in resolution, boosting a small image many times over without introducing noticeable pixelation. This potential is very useful for photography, but it does come with a caveat. The performance of AI upscaling heavily depends on the quality and diversity of the training data. If the training dataset is limited, the output might distort or misrepresent certain image types, potentially causing issues when upscaling complex letterhead designs.
Upscaling methods, particularly those employing super-resolution techniques, can differentiate between textures and patterns in an image. This ability is critical for enhancing fine details like logos or typography, ensuring a sharper and more polished result in both print and digital formats. Moreover, some advanced algorithms attempt to reconstruct elements that are obscured in the original, low-resolution image. This is particularly helpful when working with faded or low-quality scans of letterhead documents, potentially restoring lost details.
While upscaling has many applications, including removing watermarks by using AI to fill in sections based on surrounding pixel patterns, ethical implications must be carefully considered. Utilizing these techniques for copyright infringement is a concern. We also see that upscaling's computational needs are significantly reduced, making it accessible on typical hardware. This improvement makes it more practical for photographers to enhance their portfolios, potentially without requiring specialized expertise.
Furthermore, some AI upscaling systems have gained the ability to tailor their processing based on the type of image they're analyzing. This customized approach can lead to more effective enhancement of complex letterhead designs compared to simpler images. This tailoring, however, is not always effective. Upscaling can introduce artifacts if not managed carefully, hence a careful balancing act between image enhancement and preserving its original properties is required. Lastly, AI techniques are being integrated into upscaling to automatically correct colors during the process. These advancements can help to optimize color representation based on industry standards, ultimately boosting the fidelity of enhanced letterhead designs.
7 AI-Powered Techniques to Enhance Letterhead Images for Professional Photography Portfolios - Automated Watermark Removal Using Machine Learning
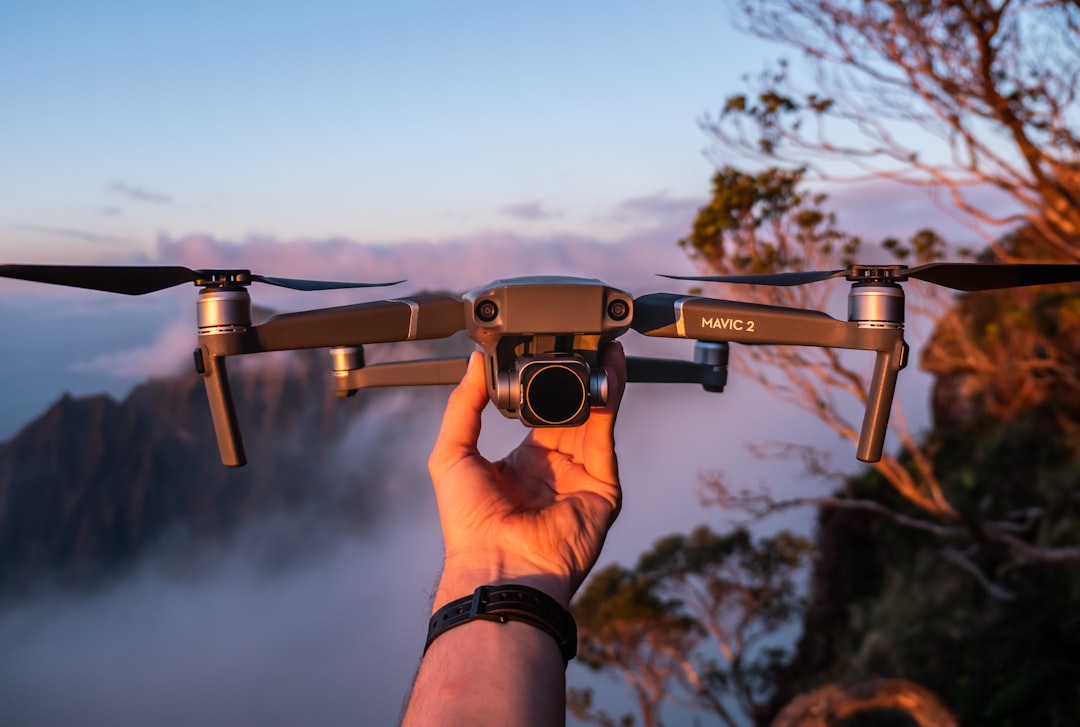
AI-powered watermark removal offers a compelling solution for photographers seeking to clean up images, especially within their portfolio context. These techniques utilize machine learning, particularly deep learning methods like convolutional neural networks (CNNs), to intelligently identify and remove watermarks from photographs. The process often leverages inpainting approaches to seamlessly fill in the areas where the watermark was, resulting in a visually seamless image.
Some advanced methods go beyond simply recognizing and erasing a watermark. They can even operate without requiring a "clean" version of the image to compare against, making them more versatile. These advancements often involve self-supervised learning or the integration of graph neural networks, which can help optimize the removal process. However, the power of this technology brings up important ethical considerations regarding its potential for misuse, specifically in copyright infringement. It's crucial for photographers to utilize such tools responsibly and be aware of the potential consequences of altering images. As this field matures, balancing the benefits of watermark removal with the ethical obligations related to image integrity will become increasingly important.
Machine learning approaches to automated watermark removal are essentially mimicking the actions of a photographer using editing software, but with significantly enhanced speed. Deep learning models can analyze the intricate pixel patterns within seconds, whereas manual techniques would require hours. This speed advantage is a game-changer, particularly in the context of large image sets.
Certain sophisticated algorithms can not only reconstruct the surrounding visual aspects but also the underlying semantic meaning around a watermark. The outcomes of this process can often be nearly indistinguishable from the original image prior to watermarking. This is where we enter a fascinating and somewhat problematic realm, as the effectiveness of this method raises questions about ethical use.
Some of the most cutting-edge watermark removal techniques employ generative adversarial networks (GANs). In this setup, two neural networks engage in a sort of competition—one seeks to generate convincingly realistic outputs, while the other aims to detect any trace of artificiality. Through this ongoing competition, the technology is continuously refined, leading to improvements in the quality of the removed watermarks.
The effectiveness of automatic watermark removal hinges on the complexity and positioning of the watermark itself. Simpler or uniformly distributed watermarks are more readily eliminated without impacting overall image quality. However, intricate or unusually placed watermarks pose more of a challenge.
Researchers have consistently noted that the datasets used to train these algorithms must be meticulously curated. Insufficiently diverse training data can lead to a form of algorithmic bias, where the technology excels at removing watermarks from certain image types but fails on others. This could result in inconsistencies in image quality.
Interestingly, during the watermark removal process, some methods can also uncover and amplify compression artifacts already present in lower-quality images. This means that careful post-processing review is required by the photographer to avoid introducing distortions that weren't originally visible.
The computational demands of automated watermark removal are typically much lower than traditional photo editing techniques. This accessibility means that photographers, even small-scale or freelance professionals, can take advantage of this sophisticated technology without the need for high-end hardware.
A recent study suggests that deep learning models built for watermark removal can operate efficiently on mobile devices. This implies that photographers can perform quick edits while on the go. However, performance and output quality might vary based on the processing capabilities of each device.
One surprising finding is that these algorithms also learn from their 'mistakes.' When a watermark removal attempt is unsuccessful, the model learns from this experience and adjusts its internal processes to avoid the same error in future attempts. This ability for the technology to adapt over time is a fascinating and often-overlooked aspect of its inner workings.
Finally, the legal considerations surrounding automatic watermark removal cannot be overlooked. While this technology certainly simplifies the removal process, it can create challenges related to ownership and copyright. This potential for conflict could lead to disputes over digital asset management, a concern that's particularly pertinent in photography.
7 AI-Powered Techniques to Enhance Letterhead Images for Professional Photography Portfolios - Enhancing Color Depth and Saturation with Neural Networks
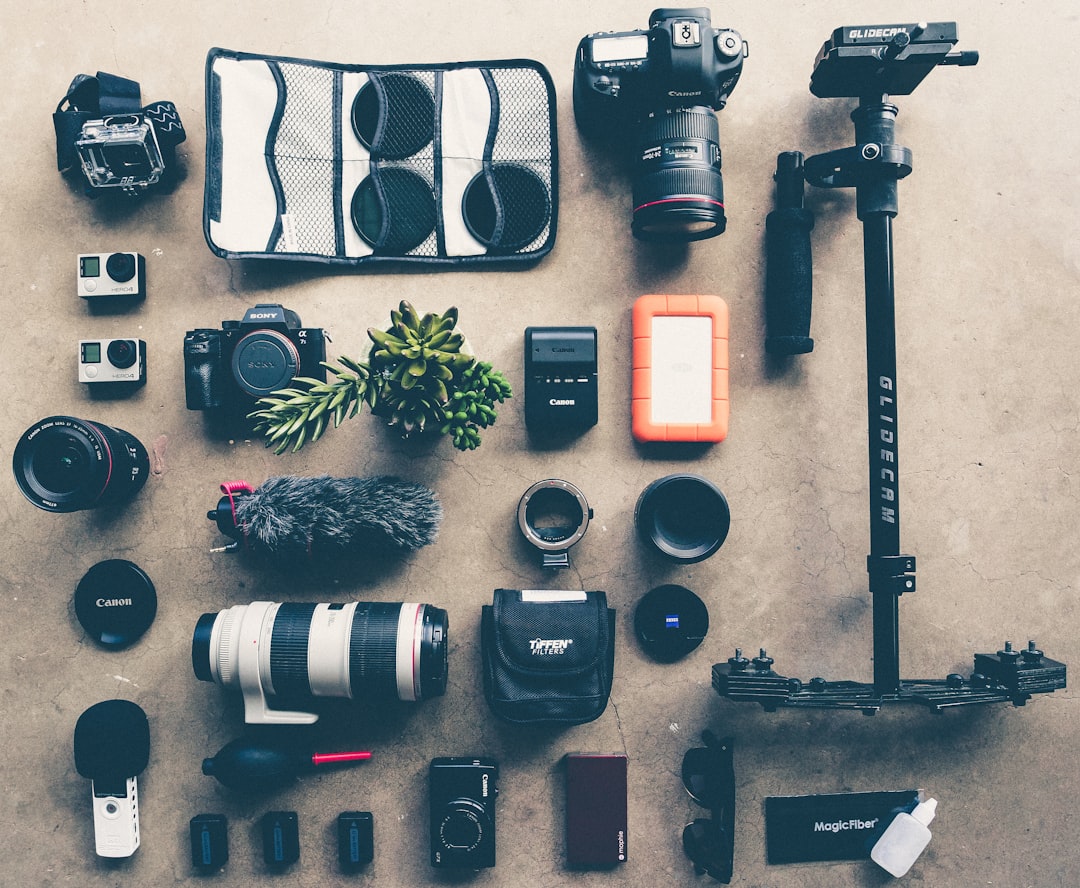
AI-powered color enhancement, specifically focusing on increasing color depth and saturation, provides a powerful tool for photographers aiming to enhance their portfolios. Neural networks trained on large image datasets can learn to identify and refine color information, ultimately leading to richer and more vibrant images. These networks go beyond simple color adjustments, dynamically improving the color fidelity often lost in various photographic situations, such as underwater shoots where natural light can distort colors. The potential of this technology to heighten the visual impact of a photograph is undeniable, potentially boosting its emotional resonance with viewers. However, caution is warranted. The quality of training data significantly affects the results. Limited datasets can cause color distortions, leading to unnatural or unrealistic color palettes rather than the desired improvements. Striking a balance between enhancing visual appeal and retaining image integrity is paramount. The continuing evolution of this technology requires users to adopt a critical perspective to avoid unintended, and potentially unrealistic, modifications to images.
In the realm of image enhancement, neural networks are increasingly being used to improve color depth and saturation, drawing inspiration from how humans perceive color in relation to its surroundings. This approach involves not just tweaking individual hues but also thoughtfully adjusting their saturation based on the nearby colors, leading to a more visually harmonious outcome.
Many of these AI-driven color enhancement methods rely on the RGB color model—the cornerstone of digital image representation. By intelligently adjusting the intensity levels of the red, green, and blue channels, neural networks can amplify the image's overall saturation. This can yield more visually striking pictures without straying into unnatural territory, but this comes with a challenge – achieving this balance is important.
Some advanced neural networks leverage an adaptive approach. They assess the image's initial color balance before making any changes. This custom-tailored approach ensures that the color enhancements remain faithful to the scene's lighting conditions, thus avoiding unrealistic or overly artificial results. An interesting development, but does it always work?
Expanding the dynamic range is another area where neural networks excel. They can adjust the contrast between the brightest and darkest parts of an image, resulting in a more nuanced and detailed presentation of colors. This heightened dynamic range brings out hidden detail in shadows and highlights, leading to a notable improvement in overall image quality. But the question remains, can they always replicate the natural dynamic range that a skilled photographer might achieve?
Interestingly, some machine learning models are trained using a diverse collection of artworks and photographs, absorbing the color principles and aesthetics used in art history. This learned knowledge allows for the emulation of artistic styles in the context of enhanced letterhead images. How closely can these emulations truly mirror the artistry of masters?
These models often incorporate gamma correction into their processes. Gamma correction adjusts the luminance of colors in a way that better aligns with how human eyes perceive brightness and contrast. This subtle but essential adjustment leads to a more intuitive and effective enhancement of perceived color saturation. However, the question of human vs. AI in the perception of color still is of some interest to me.
Neural networks can also use a technique called semantic segmentation to locate specific elements in images, such as logos or text. This ability to precisely identify and enhance critical areas allows for targeted saturation adjustments, enhancing crucial aspects without compromising the overall visual consistency of the image. This is a critical aspect for brand identity within letterhead designs. However, a certain amount of research is still needed to address how effective semantic segmentation is at consistently identifying such key aspects.
While neural networks hold great promise in color enhancement, they can also introduce color distortions, particularly in images with extreme bright or dark areas. Excessive enhancement can result in an unrealistic or unnatural look. This highlights the need for careful control over enhancement settings. One can see how a balancing act becomes critical.
Some color enhancement techniques use frequency-based methods like the discrete cosine transform (DCT). These methods can selectively enhance color channels without affecting the image's structure. This minimizes the risk of unwanted distortions and maintains a sharper, more accurate presentation of the original details. A sensible approach, perhaps, but does this method always avoid unwanted consequences?
Technological advancements in hardware and algorithms have led to improvements in the speed at which neural networks can perform color enhancement. This improved processing speed is making real-time color editing a tangible reality. This could lead to more efficient workflows and improved results, but whether it truly revolutionizes the workflows remains to be seen.
7 AI-Powered Techniques to Enhance Letterhead Images for Professional Photography Portfolios - AI-Driven Noise Reduction for Clearer Letterhead Photographs
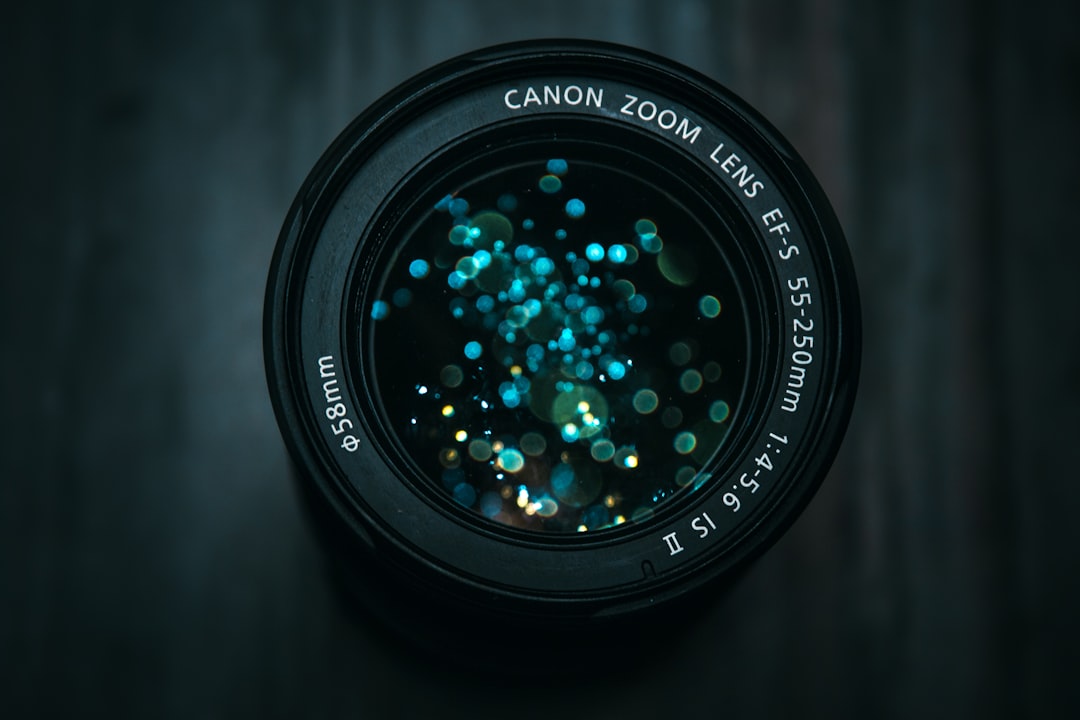
AI-powered noise reduction is becoming increasingly important for photographers aiming to enhance letterhead images. These tools utilize sophisticated algorithms to identify and remove noise from photographs, restoring clarity and detail. This is especially valuable for letterhead images, where crispness and professional presentation are crucial. Many readily available online tools offer simple noise reduction features, making it easier for photographers, even those without extensive editing experience, to improve image quality. However, the effectiveness of noise reduction can vary depending on the specific image and the algorithm used. While AI-driven noise reduction offers significant benefits, it's important to approach it cautiously, as the process can sometimes introduce unintended artifacts or distort details if not carefully managed. Overall, implementing noise reduction can significantly improve the aesthetics of letterhead images in photography portfolios, contributing to a more polished and professional look for brands and businesses.
AI-powered noise reduction presents a compelling approach for enhancing the clarity of letterhead photographs. These tools are particularly adept at discerning the difference between genuine image details and random noise, which can significantly improve the appearance of letterhead images by removing graininess and unwanted artifacts while maintaining important features like fine lines in logos or text.
Many noise reduction algorithms rely on frequency domain analysis, breaking down the image into different frequencies to isolate and eliminate noise components. This provides a more scientific approach to noise reduction, leading to measurable improvements in image quality. Notably, some advanced AI models employ adaptive noise reduction techniques, customizing the reduction level for different parts of the image. For instance, they can selectively reduce noise in the background while preserving sharp text, effectively maintaining overall image integrity.
Furthermore, advancements in AI have led to temporal noise reduction, a process useful for videos or animations. By considering information across successive frames, these algorithms can more efficiently eliminate noise, a task more challenging with static image processing methods. However, the performance of these algorithms hinges heavily on the diversity of the training data. AI models trained on a wider range of images generally demonstrate better performance across various conditions, including complex letterhead designs with diverse elements.
Moreover, AI-driven noise reduction strives to preserve the sharp details and essential features within an image. This attention to detail is a crucial advantage over traditional noise reduction techniques, which often lead to noticeable blurriness. The development of real-time processing capabilities within some algorithms allows photographers to instantly assess the impact of noise reduction on their images, optimizing their workflow.
Another intriguing aspect is the use of guided filters, which can intelligently adapt the smoothing level based on the local image characteristics. This leads to more aesthetically pleasing results, especially in intricate letterhead designs where uniform noise reduction could negatively impact the overall visual impact. Interestingly, some AI noise reduction algorithms extend beyond noise removal, incorporating image restoration techniques. This means they can potentially repair damaged areas or artifacts in older letterhead images, potentially rescuing previously unusable photographs.
However, limitations do exist. For instance, noise reduction might struggle with images taken at extremely high ISO settings, where excessive noise can overwhelm the algorithms' ability to distinguish between genuine details and noise. This can sometimes lead to a loss of texture and fine detail. Thus, managing the noise reduction level becomes crucial to prevent unwanted distortions and preserve the original photograph's integrity. The continuing development of these algorithms suggests that AI will play an increasingly important role in maintaining the quality of images, and specifically, those used in professional contexts like a photography portfolio.
7 AI-Powered Techniques to Enhance Letterhead Images for Professional Photography Portfolios - Intelligent Background Replacement for Professional Portfolios
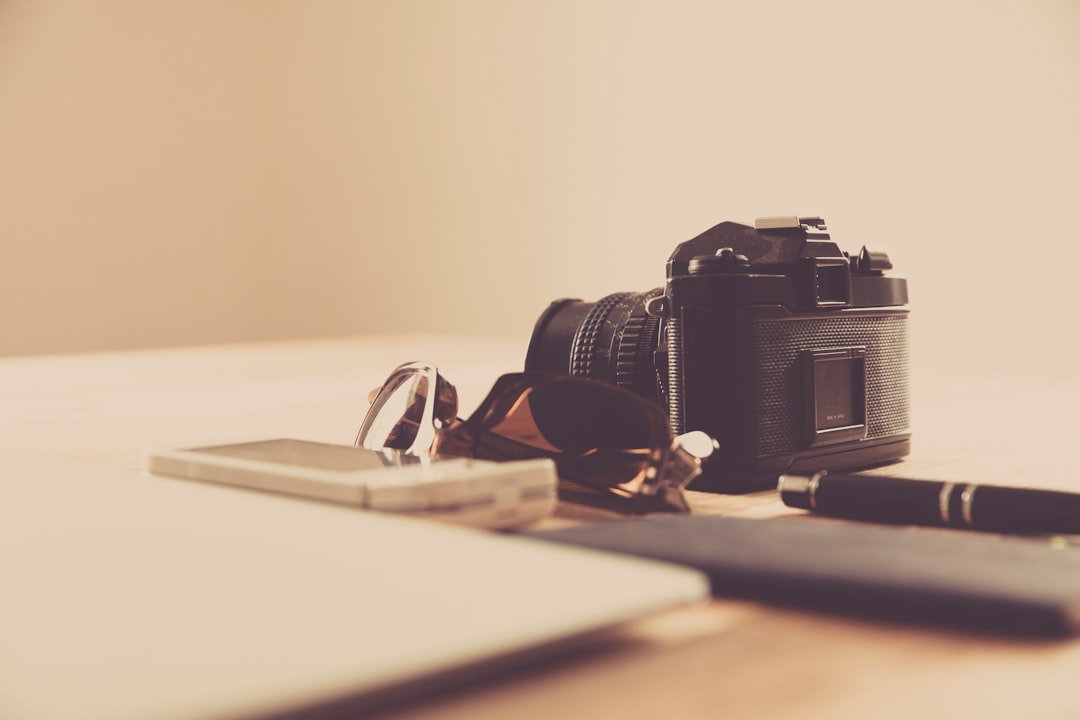
AI-powered background replacement is transforming how photographers build their professional portfolios. These intelligent tools automate the tedious process of changing backgrounds, making it easy to switch out distracting or uninteresting environments. With tools like PhotoDirector, the user can easily upload images and describe their desired background changes, and the AI quickly implements them. This capability empowers photographers, regardless of their skill level, to quickly create visually impactful images that better showcase their work. While these technologies significantly improve efficiency and add a new layer of creativity to portfolio curation, they also raise concerns about relying too heavily on artificial enhancements and potentially losing the authenticity of the original image. The ongoing challenge for professional photographers is to strike a balance between employing these tools for enhancement and preserving the integrity of their photography.
AI-driven background replacement is revolutionizing how photographers edit and present their work, particularly within professional portfolios. These tools are leveraging sophisticated deep learning models to not just manipulate pixels, but understand the semantic context of an image. This is particularly important for creating backgrounds that are visually coherent with the subject and help enhance the brand or message being communicated in a portfolio. While these tools offer promise, it's important to acknowledge that the algorithms are still in a phase of development.
The capability to replace backgrounds in real-time is gaining momentum. This ability to quickly visualize changes is a significant time saver, improving the efficiency of portfolio creation. Photographers can now experiment with different scenes during a shoot, or later during the post-processing phase. This, in turn, aids in selection of more impactful and visually compelling compositions.
These AI-based systems are exhibiting remarkable adaptability by learning across diverse image domains, such as both indoor and outdoor environments. This ability to generalize across various scenarios leads to more versatility, a highly desirable feature when creating a professional portfolio that might include shots in vastly different locations or settings.
There's also a focus on gradient-preserving techniques that are being refined within many algorithms. Maintaining natural gradients and subtle tonal changes is often overlooked, yet essential for seamless visual integration of the subject with a new background. Ignoring this can lead to a picture that looks overly-processed or unnatural.
Furthermore, the use of generative models, such as GANs, is pushing the boundaries of background replacement. These models are able to generate very realistic backgrounds, but more importantly, ensure that the overall image composition remains visually cohesive. The output can be surprisingly believable. It will be interesting to see how this area evolves further.
Some AI tools attempt to estimate the depth of a photograph to determine the location of the subject in relation to the original background. This is a step towards producing images with more realistic depth cues like shadows and highlights, that might be lost with simplistic editing approaches. However, it's still a challenge to ensure that these features are consistently reproduced accurately.
It's also becoming common to see AI systems that learn from user feedback. The system gets better over time by seeing what users prefer, which might potentially tailor portfolios to fit a specific aesthetic. This aspect of adaptive learning is interesting to consider.
The isolation of subjects from their original backgrounds is a fundamental capability of these techniques. This can be helpful to focus the viewers' attention on what is most important within a professional portfolio. It can also be helpful to bring more attention to the details or textures within a particular photograph.
Some background replacement tools have started to include more realistic bokeh simulations. The ability to control the blurring of the background (while maintaining a natural feel) allows for fine-tuning the depth-of-field within a portfolio image. However, replicating the natural look of bokeh in various lighting conditions is an ongoing challenge for AI.
There's also a growing emphasis on how AI tools can learn and retain the photographer's unique style during background replacement. This is important for maintaining a certain consistency within a portfolio, as it can enhance a photographer's personal brand or overall aesthetic. While still in the early stages, I am curious to see how these AI tools can develop the ability to emulate a specific artistic style.
The ongoing research and development in this area is quite exciting. It will be fascinating to see what improvements and innovations we see in the years ahead, particularly when it comes to professional portfolios.
7 AI-Powered Techniques to Enhance Letterhead Images for Professional Photography Portfolios - Automated Image Composition Correction Using AI Algorithms
AI-powered image composition correction is transforming how photographers refine their work. These systems employ intricate deep learning models to analyze an image's structure, making adjustments to things like the alignment of elements, the cropping of the image, and the overall balance to create a more visually pleasing result. The benefit is that photographers can spend less time on manual fixes, and they can ensure greater consistency within their portfolios.
However, this dependence on AI raises questions about whether personal artistic expression might be diminished, and whether image styles might become too uniform. Photographers need to carefully consider how to use AI enhancements while still holding onto their own unique style. Finding the right balance between using AI for improvements and preserving their artistic vision is critical to the ongoing evolution of the craft.
AI algorithms are increasingly being used to automatically improve the composition of photographs, which is particularly useful in photography portfolios. These algorithms typically analyze the elements within an image—identifying key subjects, their placement, and the overall arrangement—and then suggest cropping adjustments to enhance the aesthetic balance and harmony. The goal is to improve the visual appeal without sacrificing important details.
Some AI tools incorporate adaptive learning, meaning they can tailor their composition suggestions based on the photographer's individual style or preferences. This is quite interesting as it suggests that over time, the algorithm can develop a better understanding of a photographer's artistic vision, ultimately leading to a more consistent and cohesive portfolio.
Advanced edge detection methods are also often used in these tools to better differentiate between foreground and background elements. This ability to recognize edges and lines can sharpen certain features, which is particularly important for elements like logos, text, or other design details found in letterhead images. The ability to enhance those key features while improving the overall composition could be very beneficial.
The availability of improved processing power has made it possible for many of these AI algorithms to perform their analyses in real time. Photographers can now see composition recommendations as they make adjustments, which dramatically speeds up the editing workflow. However, there are still limitations.
Some of the more advanced tools incorporate augmented reality (AR) during the photography process. These AR features overlay virtual guidelines onto a live scene, guiding the photographer to frame the shot more effectively. This could help ensure a well-composed image even before it's taken.
Beyond just 2D analysis, some AI models use multi-dimensional data to consider depth and perspective within an image. This capability for a more thorough analysis may allow for more sophisticated and precise corrections that previously weren't possible. Similarly, certain tools analyze how viewers naturally focus their attention within an image by using eye-tracking data. The intent here is to help photographers optimize their compositions to capture viewer attention more effectively.
Researchers are developing AI models that can understand the meaning or 'semantic context' of an image. This is more advanced than simply identifying objects, as it can analyze the story behind the photograph and suggest compositions that enhance that story without interfering with its intended message or purpose.
It's worth noting that AI composition correction tools are designed to integrate with a wide variety of platforms and software. This flexibility means that photographers can seamlessly incorporate these features into their existing workflows.
However, as with many AI-powered image editing technologies, there are ethical considerations. It's important to be mindful of the potential for altering a photograph beyond the original artistic intent. The question of authenticity in digitally manipulated images is a discussion that will likely continue as these AI tools become even more sophisticated.
7 AI-Powered Techniques to Enhance Letterhead Images for Professional Photography Portfolios - Smart Contrast and Brightness Adjustment for Letterhead Clarity
"Smart Contrast and Brightness Adjustment for Letterhead Clarity" utilizes AI to refine the way photographers handle the visual appeal of their letterhead designs. These tools use sophisticated algorithms to automatically fine-tune contrast and brightness, which improves clarity while ensuring that important elements like logos and text remain sharp and readable. This automation streamlines the editing process, but also provides the ability to manually adjust settings after the initial AI adjustments, allowing users to achieve a truly professional result. This raises the issue of how to balance these AI-powered enhancements with the need for photographers to maintain a distinct creative voice. As these AI tools continue to develop, it's crucial for photographers to strike a balance between embracing innovation and preserving the authenticity of their unique styles within their portfolios.
AI-powered tools are increasingly capable of automatically adjusting contrast and brightness in images, which can be particularly valuable for enhancing the clarity of letterhead designs. These tools often analyze the image's dynamic range, making independent adjustments to different areas of the image. For instance, they can brighten text regions without overexposing other elements, resulting in a more refined presentation.
Furthermore, some of these tools incorporate perceptual models of human vision, attempting to mimic how we naturally perceive contrast and brightness. This approach helps ensure that the adjustments look natural and maintain a professional aesthetic. The algorithms often rely on histogram analysis to understand the distribution of pixel values in an image. By analyzing this data, they can intelligently adjust contrast, making sure letterheads stand out, even in more complex backgrounds.
One interesting aspect is the focus on preserving edges and fine details. This is particularly important for letterheads with detailed logos or typography, as traditional adjustments can sometimes lead to blurring. The new AI-based techniques employ localized adjustments, effectively minimizing these issues. Additionally, some AI models are now trained on color theory and design principles to align their contrast and brightness adjustments with graphic design standards. This can lead to more aesthetically pleasing results when enhancing a letterhead.
The capability of adapting based on image context is also developing. For instance, tools are emerging that can optimize adjustments for digital vs. printed media, resulting in the best possible outcome for each. It's worth mentioning that some algorithms have gained the ability to recover details lost in shadows when brightening images, which can be useful for letterhead images that might have been taken under suboptimal lighting conditions.
Interestingly, user feedback is increasingly being integrated into these systems, allowing them to learn and adapt over time. This feedback mechanism can refine the algorithms' ability to enhance letterheads, effectively creating a personalized experience for individual photographers or designers. Additionally, tools are being developed to manage color shifts that often occur when adjusting brightness. Maintaining the original color palette is critical for brand identity in letterheads.
Finally, it's exciting to see the increase in real-time processing capabilities for contrast and brightness adjustments. Photographers can now experiment with different settings and immediately see the results, leading to a more efficient workflow and faster iteration towards the desired outcome. While the field is still evolving, these AI-powered features demonstrate a promising direction for improving image quality and streamlining the enhancement process for professionals.
Create incredible AI portraits and headshots of yourself, your loved ones, dead relatives (or really anyone) in stunning 8K quality. (Get started for free)
More Posts from kahma.io: