Create incredible AI portraits and headshots of yourself, your loved ones, dead relatives (or really anyone) in stunning 8K quality. (Get started for free)
AI-Enhanced Personalization 7 Cutting-Edge Techniques for Creating Unique Phone Backgrounds in 2024
AI-Enhanced Personalization 7 Cutting-Edge Techniques for Creating Unique Phone Backgrounds in 2024 - AI-Powered Style Transfer for Unique Artistic Backgrounds
Imagine transforming your everyday photos into works of art simply by blending them with the aesthetic of a chosen image. This is the magic of AI-powered style transfer, a technique that's revolutionizing the creation of unique and personalized phone backgrounds. It works by dissecting the essence of a chosen artistic style—analyzing brushstrokes, color palettes, and textures—and then applying it to your own image. Users guide this process by picking a base photo, a style reference, and then the image they want to transform.
The recent advancements in AI, particularly deep learning algorithms, have made style transfer both more effective and user-friendly. This means anyone can easily craft personalized artistic backgrounds. We're starting to see this capability embedded in smartphone apps, allowing people to easily experiment with artistic styles and create backgrounds that truly represent their personal taste. While there are still limitations and room for improvement in this evolving technology, AI style transfer definitely provides a compelling path towards truly unique and personalized phone backdrops. It's likely to have a growing influence on how we approach personalization and design trends in the future.
AI-powered style transfer uses sophisticated algorithms, particularly convolutional neural networks (CNNs), to dissect and mimic artistic styles. These networks are adept at differentiating between the subject matter and the artistic elements within images, allowing for impressive transformations. The process itself can be remarkably fast, allowing for instantaneous stylistic changes in mobile apps. This swiftness encourages experimentation with various artistic looks, fostering a sense of playfulness.
Research suggests that using AI for image stylization can significantly boost user interaction as individuals create visually appealing and personalized outcomes. The ability to express individual tastes and creativity through unique styles often translates into increased time spent within editing platforms. Furthermore, AI trained on extensive art collections can not only recreate existing styles but also blend them together, generating hybrid styles in a way that may be difficult for a human artist to achieve organically.
Interestingly, even with the same starting image, AI-driven style transfer can yield remarkably diverse outcomes based on user input. This variability allows for a virtually endless spectrum of unique artistic interpretations, showcasing the algorithm's capacity for creative exploration. Preserving the quality of the original image during style transfer has been a major focus of development, with newer techniques like adaptive instance normalization improving resolution significantly compared to earlier methods.
Some AI-driven style transfer tools allow users to adjust parameters like stroke intensity or color palette, offering a greater degree of control over the final aesthetic. This user interaction enhances the creative process, making the outcome more personally satisfying. It's also possible to use AI to adapt a stylized image for various devices, ensuring a user's phone background appears clear and optimized across different screen sizes and resolutions.
While AI has shown promise in removing watermarks, concerns arise regarding intellectual property. Certain techniques have a remarkable ability to reconstruct images with surprisingly high fidelity, raising questions about the legality and ethics of such practices. The convergence of AI and traditional photographic techniques opens up previously unimaginable artistic possibilities, pushing the boundaries of image manipulation and offering a thrilling new frontier for photography enthusiasts.
AI-Enhanced Personalization 7 Cutting-Edge Techniques for Creating Unique Phone Backgrounds in 2024 - Deep Learning Algorithms for Automatic Image Enhancement
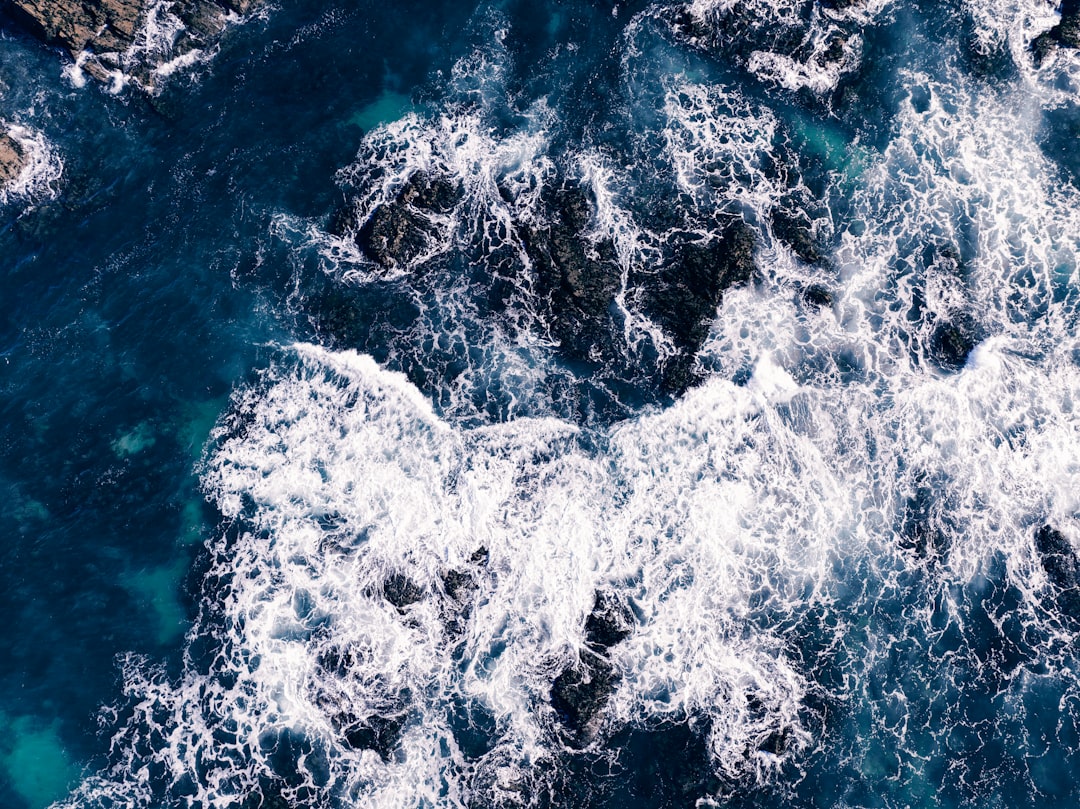
Deep learning algorithms are revolutionizing how we enhance images automatically, leading to significant improvements in photo quality. Techniques like GANs and CNNs are particularly effective at enhancing images, especially those taken in poor lighting. They can intelligently adjust brightness, contrast, and reduce noise, often surpassing the capabilities of older, more traditional methods. This level of control allows for more precise adjustments, ensuring important details within the image remain visible and impactful. Moreover, combining these cutting-edge deep learning approaches with existing image processing techniques creates a powerful synergy, opening up new possibilities for optimizing images for specific purposes. This is particularly relevant in the growing field of personalized phone backgrounds, as these algorithms allow users to tailor images to their exact preferences, leading to more visually appealing and unique outcomes. As deep learning algorithms continue to refine their ability to enhance images, we can expect to see increasingly sophisticated and impressive results in image quality and creative control.
Improving image quality automatically using deep learning is becoming increasingly sophisticated. Techniques like upscaling with GANs can take low-resolution images and, in a way, "fill in" the missing details to create higher-resolution versions without introducing distracting artifacts. It's a remarkable feat that's changing the landscape of both personal and professional photography.
Some algorithms go beyond simple upscaling by incorporating semantic segmentation. This allows them to distinguish between subjects and backgrounds in a photo, paving the way for more refined enhancements. This is exciting because it could let us make very precise edits, maybe boosting the vibrancy of a sky while leaving the foreground untouched, creating a more tailored experience.
Another area where deep learning shines is denoising. By training algorithms on image sets with and without noise, we've developed models that can effectively remove noise, especially in low-light shots. This is a big win because noise can be a major issue in these conditions, and these algorithms can help produce cleaner images without sacrificing detail.
Many of these advanced techniques employ a strategy called multi-scale processing. Basically, they examine an image at different levels of detail simultaneously. This approach allows for a more comprehensive understanding of the elements within a photo and results in more accurate and natural-looking enhancements.
Content-aware editing is another intriguing application. AI models can learn to recognize specific types of content within images – whether it's a sky in a landscape or a face in a portrait. They then apply targeted adjustments to these elements. This intelligent approach can significantly reduce the amount of manual editing required, saving users a lot of time.
We're also seeing the emergence of material transfer techniques. Essentially, these algorithms allow us to take the texture of one image and smoothly apply it to another. It's a creative tool that can potentially merge images together in interesting and harmonious ways, yielding composite images that feel cohesive.
It's important to note that AI-driven image enhancement isn't just about achieving hyper-realism. Some approaches prioritize artistic interpretations over absolute accuracy. This is a valuable development as it expands the creative options available. Users can choose between achieving a photorealistic look or applying more stylized filters, allowing for a broader range of expression.
Interestingly, many tools now include A/B testing features. This is useful because it allows us to compare the results of different enhancement methods directly within the application. This feature makes it easier to evaluate and select the output that best meets our needs.
The field of generative deep learning is also pushing the boundaries of what's possible. Researchers are developing methods to synthesize entirely new images based on existing datasets. It's an area with significant potential for background creation or for generating unique artistic elements by blending characteristics from different sources.
Finally, the optimization of deep learning models for mobile devices is enabling real-time enhancements. Photographers can now apply filters and edits directly while capturing images. This capability is truly transformative as it allows for immediate, spontaneous creativity without having to rely on extensive post-processing later. It is changing how we think about photography, encouraging a more improvisational and instinctive approach.
AI-Enhanced Personalization 7 Cutting-Edge Techniques for Creating Unique Phone Backgrounds in 2024 - Neural Network-Based Image Upscaling Techniques
Neural networks are bringing a new level of sophistication to image upscaling. Unlike older techniques that simply average pixel values to enlarge images, neural network approaches analyze the image itself to figure out missing details and fill them in intelligently. This creates higher-quality results with less of the blurring or pixelation that used to be common in upscaled images. Specific tools like SuperImage and Resize AI are designed to be easy to use while also prioritizing data privacy, making it possible to improve image quality on your own devices. This kind of advancement opens doors for creating distinctive and high-quality phone backgrounds, a feature increasingly sought after in the world of digital personalization. While upscaling technology still has room to grow, it's a promising development in image processing, potentially changing the way we perceive and personalize visual content, especially when used to create customized phone backgrounds.
Neural network-based image upscaling methods employ sophisticated algorithms to analyze low-resolution images and predict the missing high-resolution details. This is unlike traditional methods like bicubic interpolation, which simply uses a weighted average of nearby pixels. The beauty of this approach is that it can produce results that feel natural and don't have the common artifacts you see with older methods.
Interestingly, these neural networks can learn to understand the different components of an image. This is achieved using techniques like semantic segmentation, allowing for the selective enhancement of specific features. Imagine being able to make a sunset sky pop while keeping the colors in the foreground realistic—this is the type of precision we're starting to see.
One of the more clever approaches to upscaling involves analyzing the image across multiple resolutions. This multi-scale processing is beneficial because it lets the network see the larger picture as well as the tiny details. This approach often results in enhanced images that are more balanced, where minor and major elements are all treated optimally.
Moving beyond simple enhancement, these techniques can even transfer the textures from one photograph onto another. This is an exciting development because it enables creative blends and composites that maintain visual harmony. Think about layering a textured sky onto a landscape photo, keeping the overall image feeling cohesive. This is quite a step up from older methods.
Modern upscaling tools often incorporate features that allow users to directly compare different outputs side-by-side. This A/B testing approach gives users more control over the final image, aligning with the increased focus on personalization in digital imagery.
One of the problems that these algorithms address well is image noise, especially in situations with limited light. By training neural networks on a huge amount of images with and without noise, we've developed powerful denoising tools. These tools efficiently reduce noise without compromising the crucial details, significantly improving image quality, especially in photos taken under challenging conditions.
The impact of AI isn't limited to still images. These techniques can be used to improve the quality of videos by ensuring temporal coherence across frames. This approach avoids the jittery and distorted appearance that you often see when traditional video upscaling is used.
While the automation of image enhancement is quite impressive, it's not always about a one-size-fits-all solution. Many tools allow users to customize parameters such as saturation and contrast, providing a balance between automated processing and user-driven creative control. This is a key aspect of how these tools have become so valuable.
There are some ethical questions that we need to think about. The ability of AI to rebuild images can blur the lines of authorship and copyright. This raises questions about the ownership of modified images, especially those that are significantly upscaled or reconstructed. The legal and ethical implications of these powerful image processing tools require careful consideration as AI technology matures.
As deep learning models continue to be optimized for mobile devices, we're starting to see upscaling and enhancement being applied in real-time during image capture. This shift towards real-time processing is changing the creative landscape. It empowers photographers to be more improvisational and instinctive, rather than having to rely on post-processing later on.
AI-Enhanced Personalization 7 Cutting-Edge Techniques for Creating Unique Phone Backgrounds in 2024 - Advanced Watermark Removal Using Generative Adversarial Networks
Advanced watermark removal using Generative Adversarial Networks (GANs) is pushing the boundaries of image editing. Unlike traditional approaches that often struggle with intricate or randomly placed watermarks, GANs leverage the power of image-to-image translation and deep learning to achieve a higher level of removal. By essentially learning how to reconstruct the original image, these networks don't just hide the watermark, they attempt to fully restore the area, creating results that can be remarkably realistic. The use of Conditional GANs (cGANs) has further advanced this technique, resulting in even more photorealistic outcomes. While this technology can unlock more creative freedom for photographers in customizing their work, the remarkable ability to recover images without trace has brought into sharp focus important questions about image ownership and copyright. This advancement highlights a need for greater awareness regarding the ethical aspects of AI-powered image manipulation, especially as these technologies continue to improve and find their way into mainstream applications. It’s crucial for both users and developers to carefully consider the implications of such powerful tools, ensuring that the use of AI in image editing remains within legal and ethical boundaries.
Generative Adversarial Networks (GANs) have become quite powerful for removing visible watermarks, essentially using two neural networks that compete against each other. One network generates images attempting to replicate the original, watermark-free version, while the other evaluates the results, acting like a critic. This continuous back-and-forth forces the generator to refine its output, often leading to surprisingly realistic and clean results that surpass older watermark removal methods.
It's remarkable how well GANs can reconstruct detailed images even when the original is heavily obscured by watermarks or is of low quality to begin with. The deep learning model seems to learn the fundamental structure of an image, predicting and regenerating the parts hidden by watermarks with impressive accuracy. It's as if it "fills in" the gaps with information derived from the overall image pattern.
One of the best aspects of using GANs for watermark removal is their speed. On advanced hardware, these algorithms can process high-resolution images incredibly fast, making it possible to edit in real-time for both photography enthusiasts and professionals. This real-time capability opens up new possibilities for how we interact with images.
However, research shows that the training datasets are crucial for a GAN's performance. Using a very diverse and large set of training images allows the network to generalize better and achieve more convincing watermark removal. This emphasizes that the quality of the output is strongly tied to the quality and diversity of the images used to train the GAN.
Intriguingly, GANs don't just remove watermarks; they can learn to recognize and adapt to different watermark styles and positions. This adaptive ability makes them increasingly capable over time, better equipped to handle a wide range of watermark removal challenges.
While very capable, GANs do have some limitations. If a watermark is very subtly integrated into the image (like blending with the background), even the most sophisticated models can find it difficult to produce satisfactory results. This highlights the challenge of reconstructing information that isn't explicitly present in the input image.
A fascinating approach within GAN-based watermark removal is feature extraction. The network isolates key parts of the image, like edges and textures, which helps keep the image integrity throughout the removal process. This helps preserve the overall visual quality of the restored image.
The legal implications of GAN-based watermark removal are a serious consideration. Since the technology can produce practically indistinguishable copies of copyrighted images, existing legal frameworks are challenged to keep up with these AI-powered capabilities regarding ownership and intellectual property.
There's also a growing trend to involve users more directly in the process. Some GAN systems are designed to let users control how aggressively a watermark is removed, offering a balance between restoration and preserving the original content. This tailoring allows for a more personalized experience.
The uses of GANs go well beyond watermark removal; they are being explored for other things like image inpainting (filling in missing parts of images) and artistic style transfer. This wide array of applications shows the potential to create different artistic outputs, extending beyond watermark problems.
AI-Enhanced Personalization 7 Cutting-Edge Techniques for Creating Unique Phone Backgrounds in 2024 - Personalized Color Grading with Machine Learning Models
Personalized color grading, powered by machine learning, is introducing a new level of control and creativity to image editing. These advanced models delve into the intricacies of photos, allowing users to craft custom color palettes and visual styles that truly reflect their personal aesthetic. This means adjusting not just brightness and contrast, but also subtly shaping the mood and atmosphere of an image, resulting in truly unique and impactful phone backgrounds. The ability to finely tune colors and achieve a desired visual effect empowers users to express their creativity and individual style.
While offering immense potential for artistic exploration, this technology also raises ethical considerations. The ability to easily alter the core visual elements of a photo opens questions about authorship and the potential misuse of AI in artistic endeavors. Nevertheless, these models showcase how machine learning can profoundly influence the way we personalize our visual experience, particularly when it comes to crafting visually appealing phone backgrounds that resonate with our individual tastes. As these techniques mature and become more readily accessible, they will undoubtedly shape the future of photography and how we perceive the world around us through personalized imagery.
AI-powered personalization is increasingly influencing image editing, including color grading. Machine learning models are now capable of creating unique color styles that cater to individual tastes. They can be trained to understand and apply principles of color psychology, potentially leading to images that evoke specific emotions in viewers. Further, by being trained on different cultural aesthetics, these models can potentially tailor images to suit a wider range of cultural preferences.
It's also becoming possible for users to interact with these algorithms through feedback mechanisms. This interactive process, often powered by reinforcement learning, helps fine-tune the model's output and creates a more satisfying user experience. The scope of these algorithms isn't limited to still images; they're being developed for dynamic color grading in video as well. By understanding the context of different elements within an image through segmentation, models can intelligently adjust colors selectively, giving users more creative control.
Beyond adaptation, some algorithms can even generate entirely new color variations based on user-defined parameters. This generative capability enables a level of artistic exploration and manipulation previously unseen. The use of histogram analysis in these models attempts to replicate the way human vision perceives color, potentially leading to more natural-looking enhancements.
However, as with other forms of AI-powered image editing, there are ethical concerns. The ability to drastically alter the color and tone of photos representing real-world events brings into question issues of authenticity and representation. It’s crucial to consider these implications as the technology becomes more readily available.
Interestingly, algorithms are starting to leverage user engagement data, like click rates and time spent viewing images, to further optimize the results of color grading. This data-driven approach helps to adapt the models based on actual user preferences. Additionally, these models are showing a growing ability to analyze trends in color grading across platforms, enabling them to incorporate currently popular styles into the process. It's fascinating to consider how this constant learning and adaptation will shape the future of personalized imagery and, more broadly, visual content. While still in its early stages, personalized color grading is a compelling example of how AI is beginning to deeply impact how we interact with and interpret images. It will be fascinating to observe how this field develops, and what creative possibilities it unlocks in the coming years.
AI-Enhanced Personalization 7 Cutting-Edge Techniques for Creating Unique Phone Backgrounds in 2024 - AI-Driven Background Generation from Text Descriptions
The ability to generate phone backgrounds directly from text descriptions using AI is transforming how we personalize our devices. AI algorithms are becoming adept at interpreting text prompts and translating them into visually rich backgrounds. Users can now describe the desired style, texture, or lighting conditions, and the AI can generate a custom image that meets those specifications. This level of control offers a path to genuinely unique phone wallpapers that reflect personal tastes. While democratizing access to creative design, it also presents some challenges. The ease of creating backgrounds through AI prompts questions about originality and the potential blurring of lines regarding artistic authorship. Despite these questions, AI-driven background generation represents a significant advance in digital design tools. It brings a fresh wave of creative options while also forcing a re-evaluation of what we consider original design and artistic creation.
AI-driven background generation, powered by text descriptions, is an emerging area with fascinating potential for personalization. It leverages the ability of AI to interpret language and translate that into visuals, effectively creating customized backgrounds based on what a user describes. This opens up possibilities for generating unique phone backdrops that go beyond simple color adjustments or static image choices.
One of the interesting aspects is the algorithm's capacity for context awareness. A well-designed system can interpret the subtleties of a description, understanding not just the literal words, but also the implied mood, setting, or overall vibe. For instance, a prompt like "a serene beach at sunset" might yield a background that subtly evokes feelings of calm and tranquility, rather than simply showing a beach. This ability to understand the semantic meaning behind words is becoming increasingly sophisticated through the use of natural language processing.
The methods employed in this generation process are often rooted in generative models, like GANs or VAEs. These systems are trained on massive datasets of images, essentially learning the statistical patterns that define different styles and visual elements. This training allows the AI to not only recreate existing styles but to also synthesize novel and creative combinations. The result is that users can explore a wide range of backgrounds, including ones that might not be easily captured through conventional photography.
Of course, a major aspect of making this technology useful for individuals is the ability to exert creative control. The user interfaces for these AI-powered background generators are evolving to include adjustable parameters. This empowers individuals to influence the final outcome, specifying aspects like color schemes, levels of detail, or desired stylistic elements. While this process is still relatively new and sometimes requires experimentation to get the desired look, the goal is to create a seamless and enjoyable experience where the user feels a genuine connection to the final output.
Interestingly, even with the same text prompt, the output of these AI models can differ, leading to a range of visually different backgrounds. This is a consequence of the probabilistic nature of the generation process and also highlights how challenging it can be for an AI to precisely capture the nuance of human creativity. This diversity is a double-edged sword: while it can be seen as a creative opportunity, it can also make it harder for a user to consistently get the exact result they envisioned.
A notable aspect of some of the newer models is the ability to integrate multiple artistic styles. This allows users to achieve visually complex and unique combinations. For example, a user could blend elements of realism with abstract art, producing a background that is both aesthetically engaging and deeply personalized. This capability demonstrates how AI can facilitate artistic expression in ways that were previously impractical.
Furthermore, the background images generated by these AI systems are often optimized for various screen resolutions and aspect ratios, ensuring a smooth and high-quality experience across diverse devices. This adaptability is essential in the realm of personalized phone backgrounds where individuals frequently switch between different screen sizes and devices.
However, it is important to be aware of the potential for misuse. The ability to generate believable imagery from simple text instructions also raises the potential for creating misleading or even harmful content. This technology is powerful, and its responsible use is essential.
Looking ahead, we are likely to see even more rapid progress in this area. The ongoing development of faster and more powerful processing capabilities will enable real-time background generation. This would be particularly valuable for applications like video conferencing or live streaming, where users could instantly tailor their virtual backdrop to match a specific context. Additionally, the growing use of data analytics will likely lead to more personalized suggestions and options, allowing these AI tools to better understand and adapt to individual user preferences over time. This feedback loop has the potential to significantly enhance the overall user experience and push the boundaries of personalization even further.
In conclusion, AI-driven background generation presents a unique opportunity to explore creative possibilities and enhance our personal digital experiences. As this technology matures and becomes more readily accessible, it will likely continue to play an increasingly important role in shaping how we create, customize, and interact with our digital environments.
AI-Enhanced Personalization 7 Cutting-Edge Techniques for Creating Unique Phone Backgrounds in 2024 - Smart Object Detection and Removal for Cleaner Backgrounds
Smart object detection and removal is a burgeoning field, fundamentally altering how we refine and personalize our phone backgrounds. AI-powered tools are now capable of automatically recognizing and removing unwanted objects within images, be it a person, a car, or simply clutter. This sophisticated technology streamlines the editing process, simplifying the creation of clean and aesthetically pleasing backgrounds. Platforms like PhotoRoom make quick work of removing objects, allowing users to swiftly "clean up" their chosen images with minimal effort. However, it's not just about object removal; some tools are also starting to create transparent backgrounds or let users modify the background itself. This is enabling a new level of personalization where the user has a stronger hand in designing their desired digital space.
While it's easy to use these tools, as they become more widely used we need to start thinking about the ethical side of things in digital imagery. How much editing is too much? These tools are becoming increasingly sophisticated, and it's an area worth considering as we see these tools adopted further in the mainstream. Overall, it represents an exciting avenue for individuals to express their unique tastes in phone backgrounds while prompting a thoughtful discussion about creative freedom within the realm of digital imagery.
AI-powered image editing is making leaps and bounds, particularly in the area of background cleanup. Tools that can intelligently detect and remove objects are becoming increasingly sophisticated, offering a glimpse into a future where editing can be both intuitive and powerful.
One of the interesting things these algorithms do is consider the whole picture, not just individual pixels. They can recognize objects within a complex scene, like a dog in a park or a car in a cityscape. This 'contextual awareness' allows for a more precise separation between the object and the background, leading to cleaner and more natural-looking edits. Additionally, algorithms are getting better at finding the exact edges of objects, even when the boundary is blurred or gradual. This increased 'edge detection precision' helps reduce weird artifacts often seen in simpler background removal tools, ultimately resulting in higher quality images.
Another key improvement has come through semantic segmentation. The AI isn't just recognizing objects; it's also categorizing each pixel within the image. That means it might be able to tell the difference between a sky, water, or a person, giving it a better understanding of the scene's structure. This allows for a smarter background removal process, preserving fine details (like hair strands) and handling objects with translucent parts effectively. It's fascinating to see how these AI models can now 'fill in' areas after removing an object using generative inpainting. They essentially learn the surrounding visual style and try to 'guess' what was behind it, reducing the awkward empty spaces you might get with older methods.
There's also been exciting development on the speed front. Some applications now offer real-time background removal, right on a smartphone or tablet. This allows for a more spontaneous editing workflow, allowing people to experiment with background removal as they're taking pictures, rather than being limited to a separate post-processing step. The quality of these AI tools is heavily dependent on the variety of images used to train them. If the AI is exposed to a wider range of scenarios and visual elements, it's likely to be more accurate when dealing with different types of objects and backgrounds.
Similarly, analyzing an image at multiple levels of detail (using multi-resolution analysis) allows for a more nuanced understanding of the scene, especially in cluttered or complex environments. This comprehensive approach helps to ensure the background removal is both effective and doesn't compromise the visual quality of the important parts of the image. The power of these AI background removal tools has raised some interesting discussions. It begs questions about what we consider authentic in images and raises some thorny copyright issues – when we make these types of edits, who owns the resulting image and how does it impact the original?
Thankfully, newer applications are designed to put more control into the hands of the user. People can fine-tune the intensity of background removal and how the final image looks, which encourages more responsible use and personal creative input. It's also interesting to see researchers experimenting with combinations of AI techniques and traditional image editing. For example, using automated object detection alongside manual tweaks can produce highly refined results, blending the precision of algorithms with human artistry. The convergence of AI and traditional image processing is accelerating, offering photographers a more flexible and powerful toolkit for enhancing images and refining backgrounds. While there are still limitations and evolving ethical considerations, these techniques suggest a more intuitive and efficient path for shaping our visual experiences.
Create incredible AI portraits and headshots of yourself, your loved ones, dead relatives (or really anyone) in stunning 8K quality. (Get started for free)
More Posts from kahma.io: