Create incredible AI portraits and headshots of yourself, your loved ones, dead relatives (or really anyone) in stunning 8K quality. (Get started for free)
AI Image Generation in High-Stakes Training How VR Simulations Are Revolutionizing Product Safety Photography
AI Image Generation in High-Stakes Training How VR Simulations Are Revolutionizing Product Safety Photography - Amazon Sellers Replace Studio Photography With AI Product Image Generator VRStudio
Amazon is experimenting with a new AI-powered tool called VRStudio, aiming to revolutionize how sellers create product images. Instead of hiring photographers and managing studio setups, sellers can now use this AI to generate images that reflect their brand identity. This shift offers several benefits, including a quicker and potentially cheaper route to producing high-quality visuals. The belief is that more attractive product images will lead to higher customer engagement and better ad performance.
However, this reliance on AI raises concerns about whether the images remain authentic representations of the products. This technology, still in its testing phase, is being rolled out gradually in parts of North America and Europe. The success of VRStudio hinges on Amazon's ability to refine it and ensure that the generated images are not only visually appealing but also genuinely reflect the products' nature. Ultimately, the widespread adoption of this technology could redefine the role of visual content in online commerce, impacting how consumers perceive and interact with products.
Amazon's recent foray into AI-powered product image generation, dubbed VRStudio, signifies a potential shift in how sellers manage their online storefronts. This technology, which leverages AI to generate images from simple inputs like product descriptions or existing images, presents an enticing proposition for sellers, particularly those needing a quick turnaround for listings. The system essentially bridges the gap between a seller's product information and visually appealing, lifestyle-focused imagery—something traditionally achieved with laborious photo shoots.
The speed and agility offered by VRStudio are undeniable. While traditional photography often involves time-consuming setups and post-processing, this tool can generate multiple options within seconds. This efficiency could greatly reduce the time it takes to get products onto the market, particularly advantageous in fast-paced eCommerce. Further, it empowers sellers to readily experiment with product presentations, adjusting aspects like lighting, colors, and background scenes within the AI environment. This ability to test variations instantly removes the logistical constraints of physical studio setups and allows sellers to tailor presentations to a wider range of potential audiences.
However, as with other AI advancements, the rise of VRStudio raises questions about the future of product photography and its broader impact on consumer perception. The generated images, while demonstrably improving in realism, are not yet fully indistinguishable from traditional photography. The potential for ambiguity in authenticity is a growing concern, particularly as consumer trust is crucial for driving purchasing decisions. It's worth investigating how consumers will react to a world where AI-generated imagery becomes prevalent and what ramifications it might have on the perceived value and quality of a brand.
The learning capabilities of the VRStudio AI are also intriguing. By processing and analyzing existing product imagery, the system can potentially emulate successful design and staging patterns, essentially learning what resonates with consumers. This aspect could be instrumental in helping sellers tailor their product presentations to specific demographics and market niches. However, there's an inherent risk that this can lead to a homogenization of product imagery within certain sectors, potentially reducing creative diversity.
Finally, VRStudio opens a Pandora's Box regarding the role of human creativity in product photography. While the tool offers immense potential for efficiency and experimentation, its automation capabilities might eventually necessitate changes within the field. It is important to explore the ethical implications of relying on AI to fully automate product presentations, including the impact on professional photographers and other creative professionals involved in product visual storytelling. With this tool, it's becoming increasingly clear that AI could significantly reshape the eCommerce landscape and the roles that people play in the creation of product experiences.
AI Image Generation in High-Stakes Training How VR Simulations Are Revolutionizing Product Safety Photography - Safety Testing Labs Generate Virtual Product Photos Before Physical Production
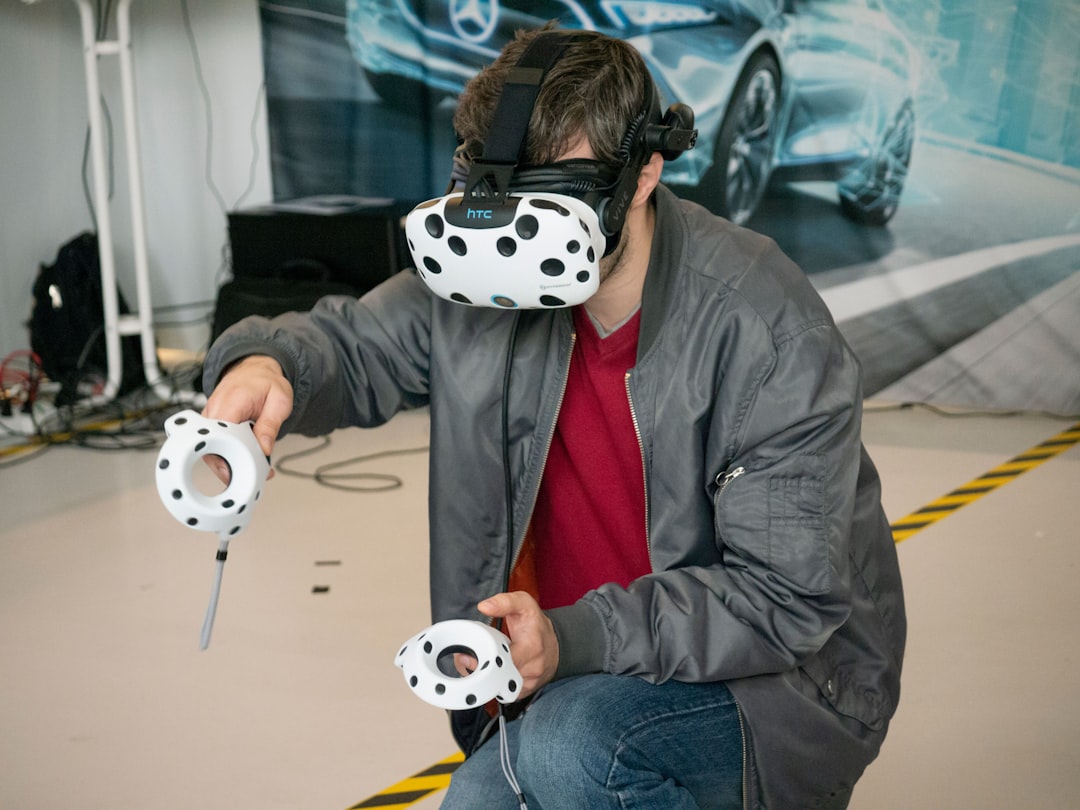
Safety testing labs are now utilizing AI-generated virtual product photos before physical products are even made. This shift in approach accelerates the product development cycle and allows for a more in-depth, visual examination of a product's safety. These AI-generated images can capture complex details related to design, environment, and lighting, which is valuable for recognizing potential hazards and improving the way consumers perceive the product. Integrating these digital simulations with safety assessments gives businesses a strong way to visualize how their products will behave in diverse situations, leading to more informed design and marketing decisions compared to the old way of using traditional photos. Yet, with the growth of this technology, the authenticity of these virtual images and whether consumers will trust them remain important issues for e-commerce.
Safety labs are increasingly using AI-generated product images before physical production starts. This is a new development that seems to be streamlining the process. It's interesting how these labs are using AI to essentially 'preview' how products will look in marketing materials before any physical prototypes are made. It makes sense that this would lead to a faster workflow, but I wonder if it's adding another layer of potential errors. If the AI-generated image doesn't accurately represent the final product, there could be confusion and disappointment down the line.
For this to work well, the AI models need really good training data. The data used to train these models heavily influences how the generated images look, covering things like lighting, composition, and overall style. In essence, the quality of the training data dictates the quality and usability of the generated images. It's all about having the right data to get a 'realistic' look.
Product image generators seem to be gaining traction as a way to quickly create a large volume of images. It is feasible to customize product images rapidly for different marketing campaigns or aesthetics. Imagine a system that could create variations of an image based on the brand's needs and target demographic. However, the question remains, will consumers notice or care if a picture is AI-generated vs. traditionally shot in a studio?
Several AI image generators are out there, like Stable Diffusion, Midjourney, or DALL-E, each with its own strengths and limitations. How these systems learn and are trained is fascinating. Are there potential ethical or intellectual property concerns regarding who owns the rights to a specific AI-generated image? The issue of ownership is definitely going to become a key challenge in the near future.
It's not just about making images; these systems can also create simulations that blend digital and physical environments. Think of showcasing a product in a realistic virtual setting. This could improve how products are demonstrated and marketed. There's a potential for enhancing the storytelling aspect of product marketing. But again, there are concerns about authenticity and whether this blending of real and virtual might lead to misrepresentation.
There are valid concerns to consider. If the training data used by these systems isn't diverse or representative, then the images might reflect existing biases, potentially leading to a less accurate portrayal of products aimed towards different demographics. Furthermore, there's always the potential for AI systems to introduce errors, leading to inconsistencies between the virtual images and the actual product. We need to be mindful of how AI might perpetuate any existing bias within the existing product photography domain.
The question arises: will relying too much on AI-generated product imagery potentially devalue or diminish the role of human creativity in product photography? With automation, could there be unintended consequences for photographers and the creativity involved in the visual aspect of product marketing? These are interesting and open-ended questions to consider moving forward.
AI Image Generation in High-Stakes Training How VR Simulations Are Revolutionizing Product Safety Photography - Automated Background Removal Tools Transform Raw Product Images Into Clean Assets
Automated background removal tools are revolutionizing how product images are prepared for online marketplaces. These tools, powered by artificial intelligence, can swiftly and accurately separate products from their original backgrounds, generating clean, professional-looking assets. This automation significantly accelerates the image editing process, lessening the need for traditional photography studios and specialized photo editing skills. E-commerce businesses can now quickly and efficiently create visually appealing product images, contributing to more efficient workflows and a stronger online presence. While these tools offer clear advantages in speed and efficiency, the increasing reliance on AI raises questions. Will the resulting images be perceived as authentic by consumers, or will the use of AI-driven editing tools lead to a sense of detachment or artificiality in product imagery? It's a complex issue that will continue to evolve as these technologies mature and influence the way consumers interact with products online.
AI-powered background removal tools are fundamentally altering the way product images are prepared for online marketplaces. These tools, utilizing sophisticated algorithms and deep learning, can swiftly extract products from their backgrounds, dramatically shortening the image processing time. Traditionally, this process was painstaking and time-consuming, often requiring manual editing or specialized software. These new automated methods can often achieve similar results in seconds.
Furthermore, the precision of these tools in identifying and isolating product details is noteworthy. They can often detect subtle textures and edges that a human editor might miss, potentially enhancing the visual appeal of the products. Many of these tools also allow for dynamic alterations of elements like lighting and color palettes, offering sellers the capacity to experiment with different styles on the fly. The potential for immediate adjustments is a powerful capability that negates the need for constant re-shoots or hours spent in post-production.
This trend is resonating within the eCommerce sector, where studies show a direct correlation between the quality of product imagery and sales conversion rates. The improvement in image quality and consistency offered by these AI solutions is clearly beneficial for businesses aiming to bolster their online presence. However, there are also growing concerns about the impact these automated tools might have on traditional photography. Product photographers are facing the reality that businesses might increasingly opt for these cost-effective and time-saving solutions, possibly leading to a decrease in demand for their services.
These AI-powered tools don't simply remove backgrounds; they're also becoming increasingly capable of analyzing consumer engagement data from previous images. This capacity allows them to learn and adapt to prevailing trends, potentially shaping their outputs based on what's popular in the market. However, this also raises concerns about potential biases. As the AI learns from data, it might inadvertently reproduce existing biases, leading to an imbalanced representation of products across different demographics. The reliance on these algorithms also points to another area of concern—inconsistency in output quality. While many AI tools deliver impressive results, variations in training data can lead to inconsistencies that can confuse customers when comparing products from various sellers.
The ethical implications of AI-generated images should not be overlooked. As consumers become more accustomed to this technology, the line between AI-generated and traditionally-captured imagery will blur. This has the potential to erode trust, especially if the AI's interpretations of products stray from consumer expectations. In addition to ethical concerns, the automation of image processing could also eventually lead to a significant shift in the workflow for eCommerce. These tools are evolving to integrate seamlessly into broader platforms, simplifying the management of images and streamlining the process for sellers. The continued advancements and widespread adoption of these tools pose a multitude of intriguing challenges and opportunities, both for the businesses that use them and the consumers who interact with them.
AI Image Generation in High-Stakes Training How VR Simulations Are Revolutionizing Product Safety Photography - Machine Learning Matches Product Photos With Virtual Safety Warning Labels
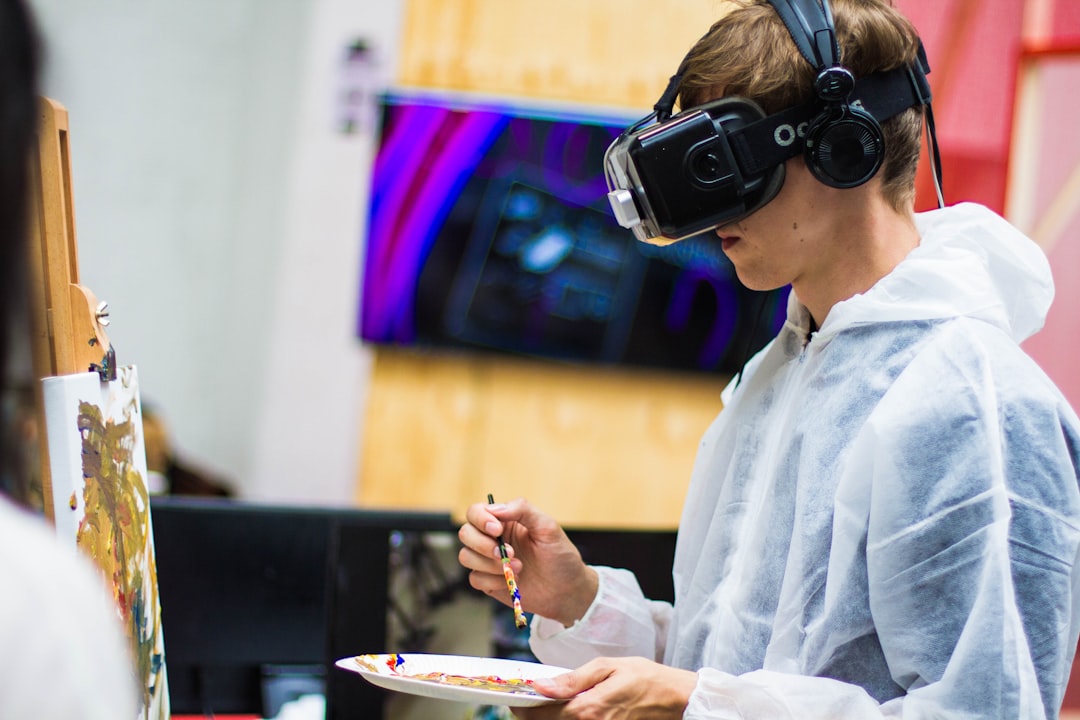
In the evolving landscape of eCommerce, machine learning is being used to automatically connect product photos with virtual safety warning labels. This approach aims to improve how safety information is communicated visually, by placing warning labels directly onto product images. This method of conveying critical safety details more directly in the imagery helps streamline communication and makes it easier to spot potential hazards.
However, this new way of presenting safety information via AI raises questions regarding the authenticity of the product images and how much trust consumers place in AI-generated visuals. These concerns are vital because purchasing decisions rely heavily on the trustworthiness of the product images.
The future of this technology will require a careful balance between enhancing efficiency through automation and preserving the authenticity and integrity of product safety information. Maintaining ethical standards in AI-generated visuals will be essential, especially as AI becomes more integrated in the presentation of safety information to ensure that it fulfills its purpose without eroding consumer confidence.
AI is increasingly being used to generate product images, leading to faster turnaround times and potentially more appealing visuals. These tools can create high-quality images very quickly, a stark contrast to the hours or days typically needed with traditional methods. There's a growing interest in how AI can learn from customer behavior. By analyzing past engagement data, product images can be tailored to specific demographics, which could lead to higher sales.
Furthermore, AI-powered tools for background removal are getting exceptionally good. They can now handle incredibly detailed backgrounds, preserving complex textures and fine edges that were once hard to achieve with manual editing. This detail and level of precision can certainly make a product image stand out.
However, even with advances in realism, questions linger about whether AI-generated images are entirely trustworthy. If these virtual images aren't a perfectly accurate representation of the real products, consumers might be disappointed or suspicious, potentially eroding trust in e-commerce.
It's also worth considering that the growing use of AI might lead to a decline in creative variety within product imagery. If AI-generated images are too similar, it could make it difficult for brands to differentiate themselves visually. The quality of the training data is a key factor in the success of these systems. If the data is poor or reflects existing biases, the resulting images might not accurately portray products, potentially leading to misinterpretations.
This increased reliance on AI brings up a lot of ethical questions, particularly concerning ownership and copyright of AI-generated images. It's a complex area that will likely spark debate in the years to come.
The use of AI in product safety is an interesting example. It allows safety testing labs to use virtual photos to pinpoint potential design issues or safety hazards long before the physical products are built. This can include simulating different environments and lighting conditions to really highlight potential problems.
AI-powered tools that enable changes to an image in real-time could be very valuable for fast-paced markets. Sellers can make adjustments during the production process without the need to create a new set of images, giving them an advantage in quickly responding to market trends.
In the end, the growing presence of AI-generated images makes it important for businesses to be transparent with their customers about how images are created. How sellers choose to handle disclosures regarding AI-generated imagery will significantly impact consumer trust. There's a delicate balance between leveraging the efficiency of AI and preserving consumer trust and authenticity in online product experiences.
AI Image Generation in High-Stakes Training How VR Simulations Are Revolutionizing Product Safety Photography - Digital Twin Technology Creates Interactive 3D Product Views From 2D Photos
Digital twin technology is revolutionizing how we see products online by creating interactive, 3D views from simple 2D photos. This means that instead of just static images, online shoppers might soon interact with virtual replicas of products, almost like having the item in their hands. This can be especially beneficial for eCommerce, allowing companies to easily show off product details or features in ways that were previously difficult. It's a pathway to possibly boosting customer engagement and potentially streamlining product design processes. But, as with any new technology, this raises questions about how genuine these 3D models are and whether they will convince customers that they accurately depict the product. As we move forward, the success of this technology will depend on how effectively it can combine captivating visual displays with honest representations of the goods being sold.
Digital twin technology, initially conceived in the early 1990s, has evolved into a powerful tool for creating virtual replicas of physical objects. In the realm of product development, this technology has the potential to transform how we visualize and interact with products, especially within ecommerce. One fascinating aspect is the ability to generate interactive 3D product views directly from 2D images. This essentially means we can use a set of photos to create a full, 3D model of a product within a virtual environment.
The ability to generate 3D models from photographs relies on advanced computer vision techniques for 3D reconstruction. This process is helping to drive the advancement of digital twins, as it bridges the gap between the physical world and the digital realm. It also allows engineers to test and experiment in a digital space before committing to costly physical prototypes. While this technology shows promise in helping to visualize products, it introduces the challenge of ensuring that the digital representation is an accurate reflection of the product's physical properties.
Digital twins are becoming increasingly interwoven with AI, particularly through the use of generative AI models. These models can learn from the real-time data streams that digital twins provide, enhancing their ability to respond dynamically to user inputs. This capability has huge potential to tailor the experience for customers based on their interactions. For example, a 3D model of a product could adapt its appearance based on different lighting conditions or user preferences.
As this technology matures, there are growing concerns about the potential for misrepresentation. The generated 3D models, while impressive, may not always perfectly match the actual product. There's always a chance that the virtual representation could lead to discrepancies between consumer expectations and the product they ultimately receive. Ensuring that the digital twin accurately captures the physical attributes of the product is critical to maintaining consumer trust.
The digital twin concept has been embraced in various fields, particularly manufacturing. For example, 'product twins' can be used to represent a product throughout its life cycle—from its initial design phase through its operational use. As digital twin technology continues to advance, it is becoming an integral aspect of the Fourth Industrial Revolution, impacting various domains, including manufacturing, product design, and ecommerce. These digital representations provide new avenues for collaborative design and for testing product behavior under various conditions, all while potentially mitigating risk by reducing the need for physical prototypes. However, navigating the complexities of these digital representations, including potential biases within the AI models, remains a challenge as they become more prevalent.
The integration of VR and XR technologies with digital twins opens up even more possibilities. Users can experience an immersive 3D environment, interacting with products in a more realistic manner. This type of experience can enhance training or provide more engaging product demos, particularly in high-stakes scenarios where safety and detailed understanding are paramount. The impact on consumer engagement with products presented in these immersive environments is a topic of continued research.
AI Image Generation in High-Stakes Training How VR Simulations Are Revolutionizing Product Safety Photography - Training Neural Networks To Detect Product Defects Through Computer Vision
Employing neural networks for product defect detection via computer vision is rapidly evolving, relying on advanced deep learning methods like Convolutional Neural Networks (CNNs). These networks are particularly adept at analyzing visual information, leading to more precise identification of intricate surface defects compared to conventional image processing techniques. The latter often struggle with the complexity of textures and fluctuating lighting conditions. The effectiveness of these neural networks is tied to the quality of the training data they receive—the better the data, the better the results. This has prompted a fundamental change in how product quality is managed and safety standards are upheld. However, despite these advances, some doubt the authenticity of products presented in online marketplaces, questioning the reliance on AI alone for crucial visual assessments. As these AI-driven approaches become more commonplace, striking a balance between operational efficiency and reliability becomes paramount within the safety and manufacturing sectors.
The use of deep learning, specifically Convolutional Neural Networks (CNNs), is significantly improving our ability to automatically detect flaws in product images. These networks are designed to mimic how humans visually analyze images, and they're proving quite effective, particularly in areas like infrastructure inspections where traditional methods often fall short.
CNNs are particularly well-suited for spotting surface defects because they can learn intricate details from large datasets, including factors like color, texture, and shape. Traditional image processing methods often struggle in situations with complex textures, varying lighting, and background noise, leading to a shift towards these more adaptable deep learning approaches. Recent innovations in neural network architectures, like DG2GAN, are exploring the use of multiple generators and discriminators to create synthetic images, which can boost defect recognition performance.
These advancements in deep learning wouldn't be possible without the parallel progress in hardware, like GPUs, CPUs, and TPUs, which enable us to train complex models on massive datasets. Computer vision systems historically relied on a lot of manual labor, but machine learning is providing a compelling alternative to the standard image processing methods. Modern defect detection heavily relies on big data and deep learning algorithms to manage the intense computational needs of complex image analysis.
A growing trend is the use of synthetic data for training these AI models. This means that instead of needing a massive collection of real-world images with defects, we can generate these defects in simulated images, which can reduce the cost and complexity of the training process.
Effectively spotting defects is vital for maintaining product quality, ensuring consumer safety, and enhancing the reputation of manufacturers. However, there's a crucial aspect related to the training data itself that needs careful attention. For instance, if the training sets contain biases, it could lead to unfair or inaccurate defect classifications, particularly in cases involving products targeting diverse demographics. This highlights a need for careful consideration of ethical implications within this AI application. While AI-driven defect detection is automating a critical process, it's worth thinking about how this automation might affect the role of humans in quality control moving forward, particularly as manufacturing moves towards fully automated production lines.
Create incredible AI portraits and headshots of yourself, your loved ones, dead relatives (or really anyone) in stunning 8K quality. (Get started for free)
More Posts from kahma.io: