AI Personalization: Examining the Evolution of Digital Portraits
AI Personalization: Examining the Evolution of Digital Portraits - Looking back how digital portraits learned to adapt
Looking back, the trajectory of digital portraits has been dramatically altered by artificial intelligence, moving well beyond earlier approaches to capturing faces. As AI technologies matured, they became more than just tools for enhancing; they evolved into systems capable of generating entirely new visual identities, a trend seen clearly in the prevalence of personalized AI headshots today. This development required digital portraiture to adapt significantly. While it undeniably broadened the creative landscape, offering new avenues for representation, it also immediately surfaced critical discussions. Questions arose about what constitutes 'realness' when algorithms can craft compelling likenesses, and whether the ease of generating AI images might, paradoxically, reduce the visual diversity we see online. The story of how digital portraits navigated these changes is very much about learning to incorporate powerful new computational abilities while wrestling with their implications for identity and expression.
Examining the path digital portraits have taken reveals some intriguing evolutionary steps:
1. Reaching a level of visual fidelity where algorithmic outputs based on immense training datasets, often from vast collections of photographs, have become genuinely difficult to distinguish from traditionally captured images in controlled perceptual studies conducted relatively recently. This indicates a significant maturation in generative model capabilities.
2. Observing the economic impact, specifically a noticeable downturn in the median market rate for simple studio portraiture over the past few years. This appears correlated with the proliferation and ease of access to sophisticated automated tools, alongside a potential increase in the supply of practitioners operating with lower overheads facilitated by this technology.
3. Investigating the emergence of systems capable of subtly adjusting portrait characteristics, such as micro-expressions or apparent lighting, in near real-time. These attempts often leverage analytical feedback loops, potentially incorporating passive sensing of viewer interaction signals, aiming to dynamically shape the perceived impact or engagement of the image. This raises questions about algorithmic influence and privacy boundaries.
4. Tracing the evolution of post-processing AI beyond simple cosmetic corrections to encompass more abstract modifications. We see the development of models purported to alter the viewer's perception of personality attributes – making a face seem more open, assertive, or reliable, for instance – based on user-defined high-level goals. This moves from manipulating pixels to attempting to manipulate psychological interpretation.
5. Exploring methods for embedding hidden, machine-readable identifiers within the synthetic image structure itself. These cryptographic markers serve as a form of digital fingerprint, intended to provide a rudimentary chain of custody or validation mechanism, crucial for verifying the origin of a portrait in an environment increasingly populated by convincing synthetic media.
AI Personalization: Examining the Evolution of Digital Portraits - Understanding the data behind personalized digital likenesses
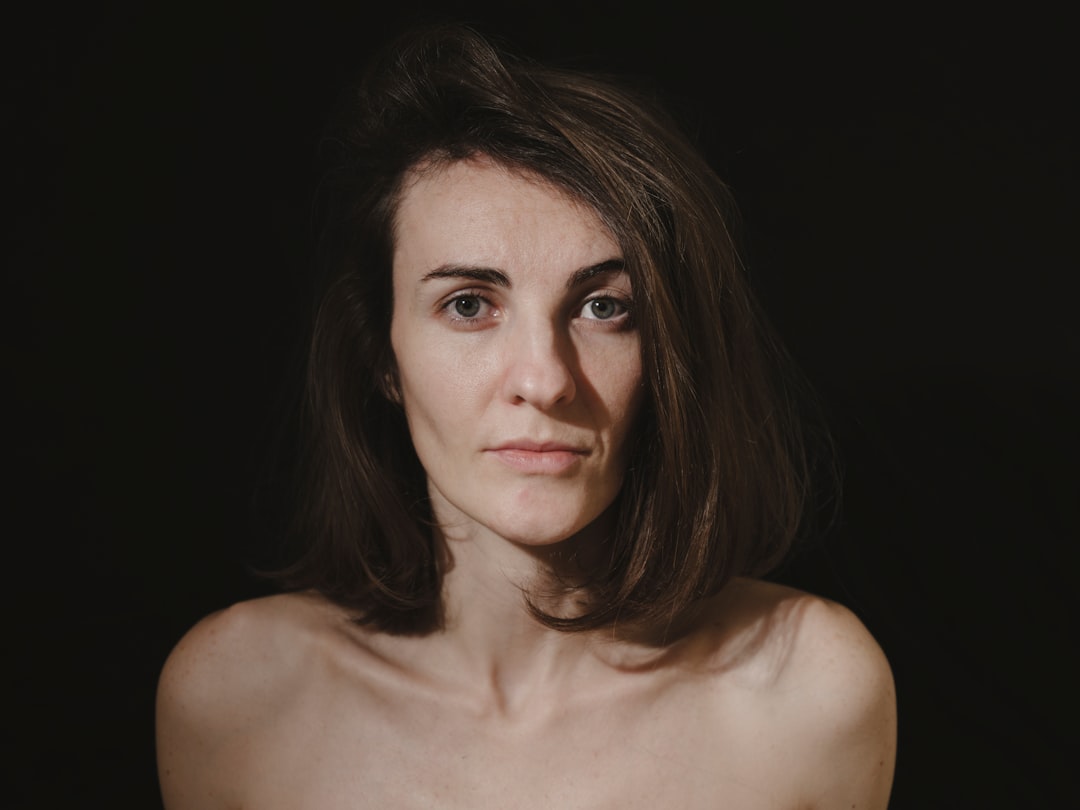
Delving into how data underpins the creation of personalized digital likenesses reveals a complex relationship between input information and the resulting image. These AI systems are fundamentally shaped by the vast volumes of data they are trained upon, often comprising immense collections of existing visual content. This reliance means the output is inherently influenced by the patterns, conventions, and even biases embedded within that training data. Consequently, understanding the data is crucial because it dictates not just the technical capability to generate a likeness, but also the aesthetic norms and visual characteristics that tend to emerge, potentially leading to a degree of algorithmic conformity rather than genuine, broad diversity. Furthermore, the sophisticated manipulation of these portraits, including subtle adjustments aimed at altering perceived attributes, is often informed by data analytics that correlate visual features with human judgments or emotional responses. Grappling with the nature and application of this data is central to addressing the ethical implications surrounding authenticity, representation, and the increasing capacity to algorithmically influence how individuals are perceived in the digital realm.
Understanding the inputs shaping individualized algorithmic likenesses
Research continues to highlight how biases inherent in the initial data used to train AI models remain a stubborn challenge in generating diverse and equitable digital likenesses. Even with dedicated efforts towards debiasing, residual patterns can still subtly privilege or misrepresent certain demographic features or skin tones.
Investigations into how viewers perceive AI-crafted portraits indicate a link between their visual properties and perceived authenticity. Counterintuitively, while clear resolution and consistent lighting are generally seen positively, pushing these attributes too far, resulting in an overly slick or 'perfect' look, can trigger an unconscious feeling of artificiality or distrust in the observer, a phenomenon still being explored through perceptual studies.
Analysis of deployment patterns on platforms like professional networks reveals a notable preference for AI-generated headshots tailored to the individual, showing adoption rates significantly higher than generic stock images or unedited personal photos. This uptake suggests individuals perceive real value in a personalized digital representation for certain social or professional contexts, which in turn informs iterative development cycles in the tools themselves.
Although the perceived economic barrier is relevant, observations indicate that user willingness to invest in AI-generated portraits often correlates positively with perceived assurances regarding data privacy and the secure processing of their original images. This points to a growing public understanding and concern surrounding the use and potential monetisation of personal, particularly biometric, information underpinning these services.
Advancements in data acquisition and processing allow contemporary generative models to analyze dynamic input, such as video footage. By applying sophisticated signal processing, these systems can extract and synthesize nuanced details like subtle shifts in facial musculature or variations in skin micro-textures over time, incorporating these into the final portrait output to achieve a level of dynamic realism and potential emotional depth challenging for systems relying solely on static photographic input.
AI Personalization: Examining the Evolution of Digital Portraits - A comparative look at obtaining a portrait the cost equation shifts
The way we acquire a portrait has undergone a fundamental transformation, particularly with the rise of artificial intelligence in image generation. Historically, obtaining a portrait meant entering into a transaction centered around the time, skill, and reputation of a human artist or photographer. Costs scaled significantly based on these factors, often placing high-quality portraiture beyond the reach of many. However, the advent of sophisticated AI models has introduced entirely new variables into this equation. The primary cost is no longer tied directly to the painstaking manual labor or unique artistic genius of an individual creating a singular piece from scratch. Instead, it’s often about accessing computational power, licensing sophisticated algorithms, or paying for subscription-based services that can generate numerous variations rapidly from basic input. This shift has dramatically lowered the barrier to entry for obtaining a personalized likeness. While it has democratized access and made high-quality digital portraits widely affordable, it also necessitates a re-evaluation of what constitutes 'value' in a portrait. Are we paying for authentic human connection and artistic interpretation, or for the efficiency and versatility of an advanced computational process? The financial landscape has undeniably expanded, presenting a much wider range of options, but prompting contemplation on the nature of the portrait itself in this algorithmically-driven era.
A comparative look at obtaining a portrait: the cost equation shifts
Observations indicate a significant reshaping of the financial landscape for acquiring portraits. Traditionally, cost was heavily tied to the photographer's time, skill, equipment, and studio overhead, often presenting a non-trivial upfront expense. The advent of capable generative AI systems introduces alternative economic models. The direct monetary cost per image can potentially be significantly lower once the underlying computational infrastructure or access to services is in place, although this assumes readily available hardware or affordable subscription access, which isn't universally true and represents a different kind of barrier than a photographer's session fee.
One clear change is the proliferation of subscription-based offerings. Instead of commissioning a one-off session, users can now pay a recurring fee for the ability to generate multiple likenesses over a period. This democratizes access to what appears visually similar to professional-grade outputs for individuals or small needs, shifting the cost burden from a potentially large lump sum to a more manageable, ongoing operational expense for the user.
Exploring distributed or more localized AI generation presents an interesting divergence in the cost discussion. While such approaches might reduce reliance on commercial centralized services – potentially lowering their associated fees or privacy costs – they typically require the user to possess or acquire technical knowledge and computational resources. This effectively translates into a 'cost' measured in terms of expertise and capital investment in hardware, factors largely absent from the traditional photography transaction model.
From an organizational perspective, the economic benefits can extend beyond the per-image cost. The capacity to programmatically generate a consistent visual identity for large or dispersed teams can lead to demonstrable savings by eliminating the logistical complexities and travel expenses associated with coordinating traditional photoshoots across multiple locations. This efficiency gain in visual asset production becomes a factor in the overall cost assessment for businesses.
A less frequently discussed but important aspect of the evolving cost is the energy required for computation. Generating high-fidelity, personalized images through complex models is computationally intensive. Initial analyses suggest that scaling these processes can accumulate a significant energy footprint, presenting a hidden cost with environmental implications and driving research into more energy-efficient algorithms and hardware specifically for generative tasks.
AI Personalization: Examining the Evolution of Digital Portraits - Examining the scope of creative variation generated by algorithms
Exploring the array of personalized digital portraits generated by current algorithms reveals a complex picture regarding creative output. While these systems can rapidly produce many options tailored to individual input, closer examination suggests the scope of true creative variation might be more bounded than initially appears. The outputs often exhibit predictable patterns or stylistic commonalities, effectively generating variations *within* a learned set of conventions rather than pushing artistic boundaries or producing truly novel visual concepts. This raises questions about whether such processes genuinely foster creative diversity or merely replicate and subtly remix existing visual paradigms drawn from immense datasets. Furthermore, the efficiency of generating likenesses computationally presents a challenge to the traditional human-led creative process, potentially shifting the perceived value from unique artistic interpretation to the speed and scale of algorithmic execution. Grappling with the implications of algorithms influencing personal representation, and considering the ethical dimensions alongside the aesthetic results, becomes crucial as these tools become more integrated into how we visually present ourselves.
Observing the scope of creative variation produced by these algorithms reveals some nuanced aspects relevant to how we think about digital portraits, including those intended as professional headshots, and the shifting landscape of photography expenses.
1. There's an observable phenomenon where, despite being trained on vast datasets, the generative models, when creating numerous iterations for a single individual, tend to explore variations within a relatively constrained aesthetic envelope. This can lead to outputs that, while distinct from a photographic original, might exhibit a structural or stylistic homogeneity across a collection, suggesting a boundary or "ceiling" to the truly novel visual expressions the current architectures reliably produce.
2. Investigations into how these synthesized likenesses are perceived indicate that even subtle algorithmic manipulations of facial topology – minuscule changes in proportionality or spatial relationships between features – can influence human judgments about traits like approachability or perceived competence. Analysis sometimes points to these automated adjustments inadvertently aligning with or potentially amplifying learned biases present in the training data, correlating certain feature combinations with culturally embedded (and potentially unfounded) assumptions.
3. When considering the overall cost equation, the economic picture extends beyond the per-image generation fee or subscription price. It includes the tangible computational overheads: the significant energy expenditure required to run complex models, the infrastructure costs for storing and managing the substantial data involved in both training and generating personalized outputs, and the anticipated, though not yet fully realized, financial burdens related to technical standards for provenance tracking or labeling synthetic media.
4. Emerging research demonstrates the capacity for algorithms to move beyond static image generation by analyzing dynamic inputs, such as short video clips. This allows for the synthesis of portraits that subtly incorporate transient facial expressions or micro-movements observed over time, resulting in a digital representation that isn't fixed but exhibits a degree of temporal variation, a notable departure from traditional photographic constraints.
5. Discussions surrounding responsible AI deployment in this domain increasingly involve considering mechanisms for accountability within the generation process itself. This points towards the potential need for systems that offer a degree of transparency regarding the data influencing a specific output, some insight into the algorithmic choices made, and clear user agency over the final result, factors that are seen as potentially impacting public trust and the perception of the generated likeness's integrity.
AI Personalization: Examining the Evolution of Digital Portraits - The role of human input in shaping automated portraiture
The involvement of human intention continues to be central in directing automated portrait generation, even as algorithms become more sophisticated. While these systems excel at rapidly producing diverse visual interpretations based on provided inputs, achieving specific creative outcomes or conveying particular emotional qualities still heavily relies on human direction. It's often the user's curation of source material, their specification of aesthetic preferences, and their critical selection from algorithmically generated variations that determine the final portrait's effectiveness. This ongoing need for human judgment extends to addressing the pervasive issue of algorithmic bias; humans must actively monitor and refine outputs to ensure representation is equitable and avoids perpetuating harmful stereotypes learned from data. The idea isn't that AI replaces the creative eye, but rather serves as a powerful, albeit sometimes unpredictable, tool that requires skilled human guidance to translate a personal or artistic vision into a digital likeness. Ultimately, the quality and integrity of automated portraiture, whether for a simple headshot or a more complex artistic piece, currently depend significantly on this critical partnership between human insight and computational capability.
While automated systems are the engine of personalized image generation, the nuanced hand of human input remains crucial in shaping the final output, often in ways not immediately obvious. Observing current practices and research directions offers insights into this interplay.
Experimental evidence suggests that injecting even sparse, strategically timed human feedback signals during the initial training phases or subsequent refinement cycles significantly improves the perceived naturalism and qualitative acceptance of the generated portraits. This highlights the unique capacity of human judgment to guide algorithmic optimization in ways that objective metrics alone currently struggle to capture.
Intriguingly, analysis of how individuals react to these synthesized likenesses often points to a preference for outputs that incorporate subtle, almost random variations – minor textural inconsistencies or slight deviations from strict photographic symmetry – over those that present a computationally perfected, hyper-smooth appearance. This suggests our visual perception actively favors cues associated with natural, human variability, interpreting their presence as contributing positively to the perceived 'authenticity' of the image.
Empirical observations of how users interact with the generated options highlight a frequent and sometimes extensive phase of post-generation human curation and manual adjustment before a final likeness is accepted. This suggests that, regardless of the system's objective quality assessment, the ultimate determination of a 'good' or suitable portrait remains deeply personal and driven by the individual's subjective interpretation and desired self-presentation.
Increasingly, systems are incorporating mechanisms for users to guide the generative process towards specific, personalized aesthetic outcomes, effectively allowing individuals to 'instruct' the AI to render their likeness in a particular style or with a desired visual feel. This goes beyond passive data input, enabling a more active form of human control over the artistic direction of the final output.
Looking further ahead, preliminary research is exploring novel interfaces where the subject's real-time physiological signals – perhaps subtle changes in heart rhythm or basic neural activity – could provide a dynamic input stream used to subtly influence the AI's iterative refinement of the portrait. This represents a highly speculative frontier, attempting to link internal human states directly to the outputting visual form in a non-traditional feedback mechanism.
More Posts from kahma.io: