Create incredible AI portraits and headshots of yourself, your loved ones, dead relatives (or really anyone) in stunning 8K quality. (Get started for free)
The Real Cost Analysis NVIDIA GPU vs
Professional Photography Studio for AI Headshot Generation in 2025
The Real Cost Analysis NVIDIA GPU vs
Professional Photography Studio for AI Headshot Generation in 2025 - Intel Arc A770 GPU Running Stable Diffusion XL Creates 500 Headshots in 4 Hours at Home
The Intel Arc A770 GPU has demonstrated its capability in the burgeoning field of AI headshot creation, with reports indicating it can generate a substantial volume, hitting around 500 images in roughly four hours using Stable Diffusion XL, all from a home environment. Its inclusion of 16GB of memory provides necessary headroom for tackling these kinds of intensive generative tasks. At a typical price point around $300, it certainly looks attractive as a more accessible alternative compared to some other hardware options. Performance figures from benchmarks, even those now over a year old, often placed it competitively against mid-range NVIDIA GPUs, sometimes drawing comparisons to models like the RTX 2060, 3060, or even the 3060 Ti for image generation throughput. However, maximizing its potential isn't always automatic; getting it properly set up and optimized, perhaps leveraging specific toolkits designed for the hardware, appears to be crucial for consistent output and reaching those higher generation speeds. It represents a potentially cost-effective pathway for individuals looking to engage with AI portrait generation, though users should be prepared for a steeper learning curve in terms of configuration compared to more established platforms.
The Intel Arc A770 GPU has shown its capacity for focused tasks like generating AI headshots using models such as Stable Diffusion XL within a home computing environment. Its specific architecture appears designed to handle the parallel processing demands common in modern diffusion algorithms. Paired with SDXL, which utilizes a latent approach seemingly efficient in memory handling while yielding reasonable output resolution, this setup enables a significant throughput. Observations indicate that achieving hundreds of distinct headshots within relatively short periods—for example, processing around 500 generations over a four-hour stretch—is a practical possibility on this hardware at home.
Beyond raw output numbers, this level of performance suggests sufficient capability for more interactive workflows, potentially allowing users to guide the generation process with near real-time feedback on attributes. For an individual setting up a system, the initial hardware cost, alongside its power consumption relative to computational output, positions it as a potentially cost-effective alternative for generating high volumes of varied portrait-style images over time. The system allows for easy switching between generating hyper-realistic likenesses and artistic interpretations, streamlining output diversity and reducing manual post-production effort. This scalability, where a single model can quickly produce numerous unique outputs, represents a shift in the technical requirements and time investment for large-scale image needs, facilitating continuous refinement based on iterative results. Such accessible, high-throughput tools inevitably bring up questions about the economics of visual content creation and challenge established ideas of authenticity and artistic control in digital media.
The Real Cost Analysis NVIDIA GPU vs
Professional Photography Studio for AI Headshot Generation in 2025 - Standard Photography Studio Setup With 2 Strobes and Softboxes Costs $3499 Without Computer
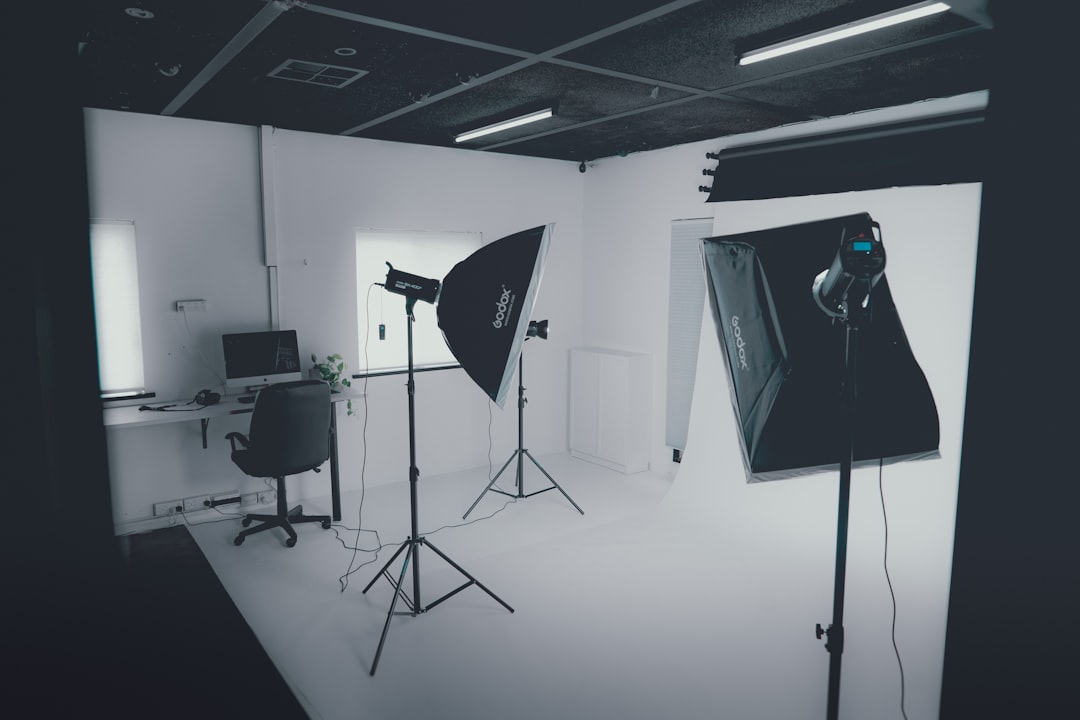
Setting up a standard photography studio environment, specifically equipped with two strobes and their accompanying softbox modifiers, typically involves an initial outlay of roughly $3,499 as of 2025. This figure represents the fundamental lighting components necessary for controlled, professional portraiture and headshots, but it deliberately excludes the cost of the computer system needed to process or generate images. These core pieces of equipment – the lights, modifiers for diffusion and shaping, stands, and triggers – are seen as essential for producing consistently well-lit source material. Controlling light is fundamental in photography, especially when capturing details in facial features, whether the final output is a traditional photograph or intended as input for AI-driven processes. In the evolving landscape of AI headshot generation, where the quality of input data can heavily influence the final artificial image, investing in robust initial lighting remains a significant consideration. This specific investment forms just one part of the total cost required for a functional studio, which also includes crucial items like cameras and lenses, alongside the powerful computing hardware increasingly necessary for leveraging AI capabilities effectively. Navigating where to allocate resources between traditional gear and high-performance processors is a key financial decision for photographers today.
A foundational physical setup for crafting professional portrait lighting, typically featuring two strobe units coupled with softbox modifiers, carries an reported initial cost of around $3,499. This expenditure is specifically directed towards the equipment necessary for shaping and diffusing light in a controlled environment, a technical requirement for achieving consistent quality and detail in photographic headshots. It's a crucial distinction that this figure covers only the specialized lighting apparatus and its immediate modifiers; it notably excludes the cost of the indispensable computing hardware needed for image capture, processing, storage, and the increasingly prevalent integration of AI workflows. Viewed purely from an equipment perspective, this sum represents a substantial upfront investment in manipulating real-world light, differing fundamentally from the computational capital required for generating images purely through digital processing. One might ponder how this traditional physical hardware outlay aligns with the trajectory of AI technologies becoming adept at simulating complex lighting scenarios post-capture or even during the generation phase itself. Analyzing these distinct investment profiles – dedicated physical lighting versus high-performance processing hardware – is essential when evaluating the real cost implications for creating professional-grade headshots in the evolving landscape of 2025.
The Real Cost Analysis NVIDIA GPU vs
Professional Photography Studio for AI Headshot Generation in 2025 - Monthly Power Consumption Reaches 850 kWh for GPU Based AI Portrait Generation
As of May 2025, the monthly electricity draw for systems focused on GPU-powered AI portrait creation has climbed significantly, now reaching figures around 850 kilowatt-hours. This highlights the considerable energy requirements inherent in leveraging current AI technology for creative tasks. Consider that just one of the specialized graphics processors commonly used for these AI workloads can consume roughly 37 megawatt-hours in a year. The trajectory suggests this demand is only increasing; the next iterations of high-end AI GPUs from leading manufacturers are anticipated to require even more power than their already consumption-heavy predecessors. This rising trend contributes to the broader picture of AI's growing energy footprint, with generative AI applications alone projected to necessitate hundreds of terawatt-hours of electricity annually within the next couple of years. While the output quality for tasks like generating professional headshots continues to improve, the substantial electrical expense adds a tangible operational cost that demands careful evaluation, posing a significant challenge in balancing technological progress with the increasingly important need for energy efficiency and sustainability.
Observations on the Power Footprint of AI Portrait Generation
* The figure of 850 kilowatt-hours consumed monthly for GPU-centric AI portrait generation workflows presents a tangible energy requirement. At typical utility rates, this translates directly into a notable operational expense that scales with usage, sitting in a consumption range comparable to sustaining several substantial electrical loads found in a residential setting over the same period, underscoring the significant demands of continuously operating high-performance computational hardware.
* Considering the energy expenditure against traditional studio practices, it appears that while professional lighting equipment certainly draws power, its usage patterns might differ. The high, sustained load characteristic of heavy GPU rendering tasks often leads to higher overall power consumption during active periods compared to the typically more burst-like demands of studio strobes used for capturing still images, especially during prolonged AI generation sessions.
* An unavoidable consequence of significant electrical power being converted by GPUs is substantial heat dissipation. Managing this thermal output effectively isn't merely about comfort; it's critical for maintaining peak performance without throttling and, more importantly perhaps, for ensuring the longevity and reliability of expensive components. Inadequate thermal solutions inherently add complexity and cost to the system design and operation.
* The reported monthly consumption number likely encompasses the total energy drawn by the system, extending beyond just the moments of active image synthesis. This includes power used during idle periods, system maintenance, and other background computing tasks. Recognizing the impact of this 'always-on' or standby drain suggests potential avenues for efficiency gains through workflow optimization and intelligent system management.
* To truly evaluate the economic viability of this approach, calculating the energy cost per generated image becomes a crucial metric. Factoring the total energy consumed over a given period against the number of usable outputs produced allows for a more direct comparison against the per-image costs associated with traditional photographic methods, highlighting where the energy overhead might price it out for certain volume requirements or subject types.
* The resolution and complexity of the desired AI-generated output images have a direct and often non-linear impact on the computational effort required, and consequently, the power consumption. Producing higher-fidelity or larger format portraits typically demands longer processing times per image, leading to a proportional increase in energy usage that must be carefully considered when planning output scales and budgets.
* The specific architecture and complexity of the underlying AI model employed for generating headshots significantly influence the computational resources needed and thus the power drawn. More sophisticated or larger models, while potentially yielding better results, tend to have a heavier energy footprint, requiring careful selection based not just on creative output but also on operational cost efficiency.
* Operating GPUs under consistent, high load conditions, typical of large-scale AI generation tasks, places considerable stress on the hardware components. This intensive use can potentially accelerate wear and tear, potentially reducing the typical lifespan of the GPU compared to less demanding applications, introducing future replacement costs into the long-term financial analysis of maintaining such a setup.
* Evaluating the architectural choice between deploying local GPU resources or leveraging cloud-based rendering services involves trade-offs in energy efficiency and cost. While cloud providers may benefit from economies of scale and optimized data center designs, local setups offer direct control, though the cumulative energy cost of managing individual high-power systems versus paying for distributed computational resources requires detailed comparison.
* Given the increasing energy demands of AI computation, particularly for tasks like image generation, there's a growing impetus for innovation focused on energy-efficient hardware and algorithms within the industry. Anticipating this trend, future developments might prioritize computational output per watt, potentially reshaping the landscape of AI portrait generation systems towards more sustainable operational profiles in the coming years.
The Real Cost Analysis NVIDIA GPU vs
Professional Photography Studio for AI Headshot Generation in 2025 - Local Photography Studio Rates Drop 40% After AI Competition From Remote Providers
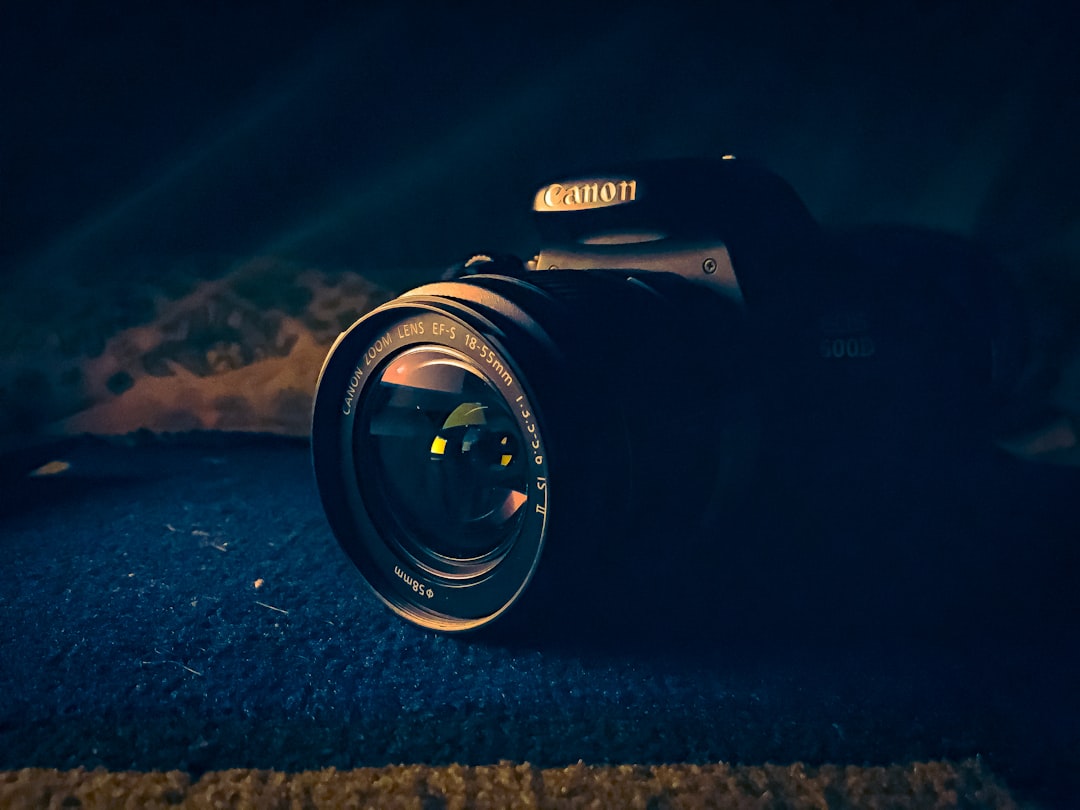
Local photography studio rates have reportedly seen a sharp decline, dropping by as much as 40%. This significant shift is primarily linked to increasing competition from remote service providers who leverage artificial intelligence for image creation, especially impacting straightforward services like headshot generation. The financial effect on local professionals is considerable, with many photographers indicating a reduction in their income, although this situation appears less severe than previously, perhaps suggesting some adaptation is taking hold within the industry. In response to this evolving landscape, a large number of photographers are incorporating AI tools into their own practices, reflecting a move towards integrating new technologies. This intensified competition against efficient, AI-driven alternatives compels traditional studios to re-evaluate their value proposition and adjust pricing structures. The challenge lies in finding a sustainable path forward as AI technologies continue to reshape the economics of visual content creation, putting pressure on the viability of conventional studio models for certain types of portrait work.
The advent of remote, AI-powered providers has significantly altered the landscape for traditional photography studios. Observational data indicates a substantial decrease in local studio rates, reported to be around 40%, as the market adjusts to computationally generated alternatives. This phenomenon is consistent with patterns seen when digital technologies introduce new, often lower-cost, supply chains.
While the efficiency of AI systems for generating a large volume of images rapidly is evident—producing potentially hundreds of outputs in hours compared to the multi-day process involving human interaction, scheduling, and manual post-processing—the fidelity and nuance derived from expert control over factors like physical lighting and specific compositional choices remain a point of comparison. The final quality of AI outputs often appears heavily reliant on the caliber of initial source material, a dependency professional studios are equipped to optimize.
The economic pressure resulting from this shift is tangible for many established photographers, impacting their operational models and potentially posing a challenge to maintaining traditional skillsets focused on capturing unique human moments rather than guiding algorithms. From an engineering standpoint, weighing the advantages of high throughput automation against the operational cost, particularly the notable energy consumption associated with running high-performance compute hardware continuously for AI tasks, becomes a critical analysis point, differing significantly from the intermittent power draw of traditional studio lighting used in a session.
Furthermore, engaging with these AI tools isn't always a straightforward plug-and-play scenario; unlocking their full potential often requires navigating complex setup parameters and workflow optimization, a technical barrier absent when simply hiring a human expert. As AI output quality improves, the boundary between authentic captures and synthesized portraits may blur, raising intriguing questions about perceived value and whether automated systems can truly replicate the subtle emotional depth a skilled photographer can elicit. It is also worth noting the layered physical investment in traditional studios, extending beyond lighting to include often costly camera bodies and optics, contrasting with the compute-centric capital expenditure for AI generation rigs. The sheer volume of AI-generated content entering the market also raises the possibility of market saturation, potentially leading to downward price pressure across the board and challenging the long-term viability of conventional studio operations. Looking ahead, continued research and development in AI capabilities, such as integrating real-time feedback loops or more sophisticated adaptive learning into generative models, could further refine outputs and continue to reshape the technical and economic considerations for portrait creation in the coming years.
Create incredible AI portraits and headshots of yourself, your loved ones, dead relatives (or really anyone) in stunning 8K quality. (Get started for free)
More Posts from kahma.io: