Exploring the AI PS2 Filter How Machine Learning Recreates Early 2000s Gaming Aesthetics
Exploring the AI PS2 Filter How Machine Learning Recreates Early 2000s Gaming Aesthetics - Machine Learning Techniques Behind the PS2 Filter
Delving into the machine learning methods powering the PS2 Filter unveils a compelling blend of technological prowess and a yearning for the past. This AI cleverly employs algorithms to analyze and refine image elements, effectively mimicking the distinctive visual characteristics of early 2000s gaming. It achieves this by skillfully reducing pixelation, boosting resolution, and generally enhancing image clarity, all while maintaining that vintage aesthetic. While the results are quite impressive, the emergence of this technology prompts conversations about its influence on our memories and perceptions of those older gaming experiences. This tool isn't just a fun way for fans to experiment with retro styles—it also reflects a wider cultural embrace of older visual styles in a landscape increasingly dominated by hyperrealistic visuals. In essence, the PS2 Filter embodies a fascinating fusion of creative expression and sentimental yearning, which has sparked considerable enthusiasm within the gaming community.
The PS2 filter relies on convolutional neural networks (CNNs) to decipher the intricate relationships between pixels, skillfully replicating the low-resolution charm of early 2000s game visuals. Upscaling within the filter employs established methods like bicubic interpolation, but it goes a step further by incorporating machine learning to intelligently reconstruct and generate textures that echo retro graphics. This process isn't just about enlargement—it involves discerning and subtly removing or blurring elements that clash with the retro style during upscaling. This is accomplished through generative adversarial networks (GANs) which help ensure that the textures and appearance align with the PS2 era.
One of the filter's captivating aspects is its ability to anticipate missing pixel data by drawing upon a massive dataset of textures from PS2-era games. It uses statistical patterns learned from this data to guide the restoration, effectively filling in the blanks in a way that respects the original aesthetic. The filter achieves the nostalgic visual style by training on a dataset consisting entirely of images from classic games. This process enables the filter to internalize the distinctive color palettes and dithering techniques that define that era without simply replicating pixels.
Interestingly, machine learning algorithms can be applied not only for stylistic filtering but also for tasks like watermark removal. The filter intelligently identifies watermarks and reconstructs the underlying pixel data, thereby restoring the vintage visuals. Remarkably, the filter adapts its computational demands based on the input resolution, allowing for real-time image enhancement without significant performance penalties. This is achieved through specialized neural network architectures designed for efficiency.
However, achieving the desired aesthetic often involves adjusting certain parameters, or hyperparameters, that influence the filter's behavior. Different games and styles require fine-tuning for optimal results, leading to slight variations in output and the overall visual fidelity. This filter has proven valuable in remastering older game graphics, addressing a significant need to bridge legacy content with modern hardware. The ongoing refinement of the filter leverages user feedback, continually adapting and evolving based on perceived visual quality and the authenticity of the final output. This iterative process demonstrates the filter’s potential to improve over time based on user preferences and community input.
Exploring the AI PS2 Filter How Machine Learning Recreates Early 2000s Gaming Aesthetics - Analyzing PS2-Era Graphics Characteristics
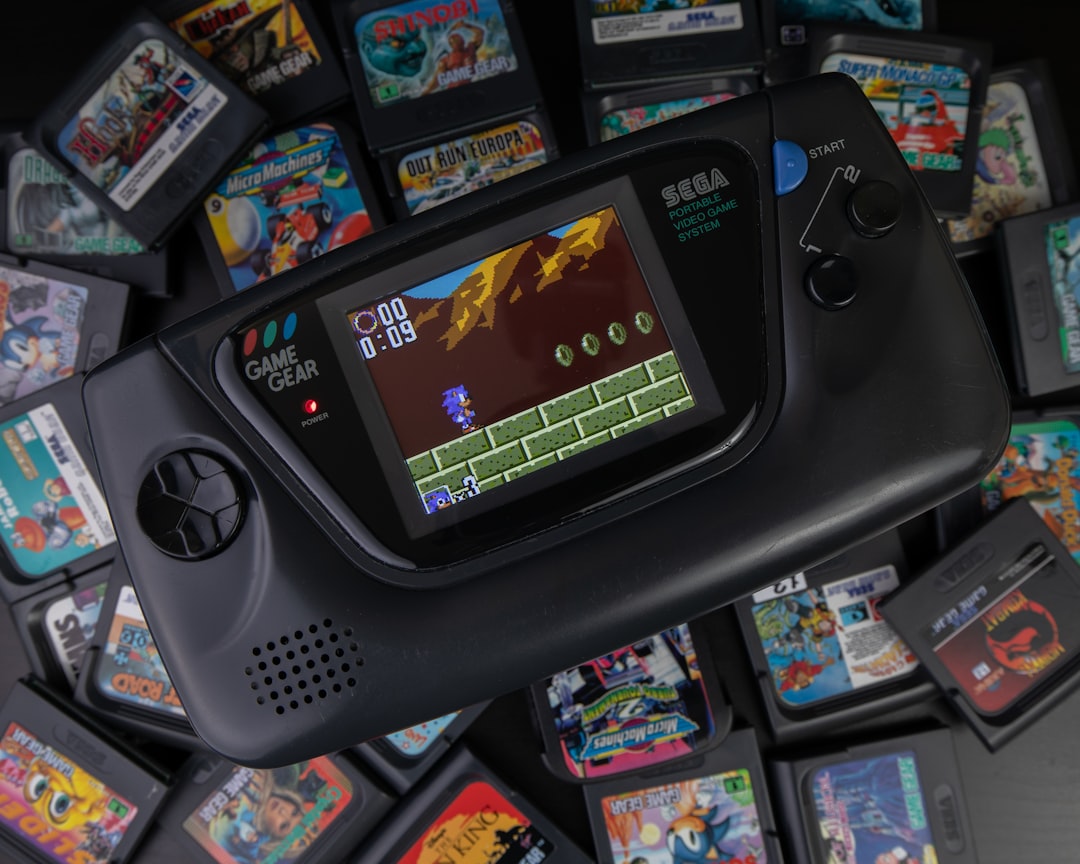
Examining the visual hallmarks of the PS2 era reveals a distinct aesthetic characterized by low-resolution images, unique textures, and specific color palettes. The games of this time often featured simple, low-polygon models and pixelated textures, a stark contrast to today's high-fidelity visuals. However, these seemingly limited graphics fostered a unique charm and sense of nostalgia for many gamers. Understanding these characteristics is crucial in appreciating the development of tools like the PS2 filter. These tools, powered by AI, attempt to recreate the visual quirks of the PS2 era by emulating its rendering styles and lighting techniques. By closely analyzing the features that made PS2 graphics recognizable, we gain a better grasp of how AI-driven filters are able to recapture the essence of this period. The effort to recreate these vintage graphics, often with tools designed for modifying existing images, showcases a desire to bridge the gap between older gaming experiences and the present. This pursuit highlights our enduring fascination with and connection to the visual language of gaming's past, a past where artistic constraints gave birth to a particular creative flair that is now being revisited with modern technologies.
Analyzing the visual characteristics of PS2-era graphics reveals a fascinating blend of technical limitations and creative workarounds. These graphics often exhibited distinct features like dithering and banding, techniques used to simulate texture and depth within the constraints of limited color palettes. Accurately replicating these artefacts is crucial for achieving a true retro aesthetic in modern image processing.
Traditional upscaling methods, like bicubic interpolation, can struggle with the sharp edges and unique pixel density of PS2 graphics, leading to results that feel blurry or lack the original's distinctive sharpness. However, machine learning approaches are able to learn these specific features and reproduce them more accurately. This ability stems from the fact that PS2 hardware was constrained by only 32MB of RAM, heavily impacting texture resolution and game complexity. Developers were forced to be creative, employing color compression methods such as palette swapping to get the most out of the limited resources. These techniques contribute to the recognizable aesthetics of the era and pose a unique challenge for AI models to accurately replicate.
Many PS2 games used temporal anti-aliasing, blurring fast-moving elements to reduce jagged edges. AI filters can emulate this by examining motion patterns in a scene, subtly smoothing out any harsh edges while preserving the overall retro feel. Machine learning excels in upscaling as it's able to examine spatial pixel relationships and learn from a massive collection of PS2 game textures. This allows it to "predict" new pixels and enhance details in a way that doesn't introduce modern-looking artefacts.
The accuracy of a PS2-style AI filter relies significantly on the comprehensiveness of the training dataset. A filter trained on a narrower range of texture styles might not handle all visual elements equally, leading to inconsistencies when compared to the original game visuals. Because PS2 graphics often relied on visual simplifications and abstractions due to low resolution, effective AI models need to factor in those stylistic choices. The aim isn't just to increase the resolution but to intelligently reconstruct missing elements in a manner faithful to the original style.
Beyond upscaling, display refresh rates of PS2 games (commonly 30 frames per second) also impacted motion perception. Some AI filters incorporate this factor, optimizing for clarity and preventing artifacts that might otherwise interfere with the nostalgic impression. The ongoing development of these filters incorporates user feedback, demonstrating that cultural appreciation for specific graphic quirks can directly impact the refinement of these AI tools. Community input refines the filters, bringing them closer to the general understanding of what defines an authentic PS2 aesthetic. This highlights a fascinating interplay between technology and human perception of visual style.
Exploring the AI PS2 Filter How Machine Learning Recreates Early 2000s Gaming Aesthetics - User Interface and Customization Options
The AI PS2 filter provides a user interface that's designed to be easy to use, making it accessible to a wide range of users, even those without a technical background. This ease of use is a positive aspect, allowing individuals to easily experiment with the nostalgic PS2 look on their images. Customization options, like controlling the filter strength, offer a level of personalization that allows users to fine-tune the retro effect to their liking. They can dial in the level of pixelation and other visual artifacts to achieve a specific aesthetic.
However, this freedom can lead to unintended consequences. While the filter is designed to mimic the PS2 era, relying solely on the user interface's adjustments without a thorough understanding of PS2 graphics might lead to images that don't quite capture the true essence of the period's visual style. It's a balance between user-friendliness and the potential for overly simplified applications that can sometimes miss the mark in terms of authenticity. The ideal situation would be to have easily understood controls that also promote awareness of the stylistic choices that made PS2 graphics distinct, preventing a superficial application of the filter. For those truly seeking a genuine PS2-era look, it's a reminder that a little extra attention to the specific details and underlying visual elements can help achieve a more accurate and engaging result.
When exploring image enhancement tools powered by AI, particularly those focused on recreating vintage aesthetics like the early 2000s gaming era, a few aspects of the user interface and customization options stand out.
One intriguing feature is the ability for the interface to adjust to the user's hardware. It can dynamically adapt its complexity and resource usage in real-time, ensuring that even users with less powerful computers can experience smooth and responsive image processing. This adaptable approach makes the technology accessible across a wider range of devices.
Many of these tools offer a remarkable level of control through customizable hyperparameters. These are the fine-tuning settings that influence aspects of the enhancement process, such as smoothing, sharpness, or even the overall color palette. Users gain the power to sculpt the visual output to achieve specific results, matching their own artistic preferences, and this can lead to a diverse range of artistic styles.
Some platforms provide pre-set configurations tailored for specific vintage styles, simplifying the customization process. Users can quickly apply settings that emulate the desired era, making it easier for those who might not be as comfortable with technical settings. This approach is helpful in making the technology more accessible for a broader audience.
Interestingly, user feedback plays a significant role in shaping these tools. Developers often integrate feedback mechanisms to capture user preferences and adjust the algorithms over time. This leads to continuous improvement, but it also points to a community-driven model of software development.
Modern interfaces commonly incorporate interactive preview functions, allowing users to see the original image side by side with the enhanced version. This visual feedback is immensely helpful for decision-making, enabling real-time adjustments and providing a clear understanding of how changes impact the final result.
Beyond simply upscaling, some tools employ AI to automatically apply the essence of classic game styles to user-provided images. Rather than just a simple resolution boost, these tools try to understand the style and apply it to new content. This aspect blends nostalgia with the user's current content, leading to some interesting creative possibilities.
The capacity to process multiple images efficiently is another notable feature. Many tools support batch processing with customizable queues, which is convenient for improving efficiency when working with large numbers of images. Users can define specific styles and settings for each image within the queue, ensuring both efficiency and individual control.
Some of the more sophisticated tools offer layered editing features, allowing users to treat different enhancements as separate layers. This flexible editing approach offers greater control over the final result and makes it easy to switch between effects, refine individual aspects, or undo changes as needed.
It's also worth noting that AI is being increasingly applied to tasks like watermark removal. While traditional techniques sometimes introduce unwanted artifacts, some modern solutions intelligently replace watermarked areas with compatible textures. This method aims for seamless removal that respects the overall visual integrity of the image.
Finally, these tools are starting to integrate with other popular graphic design and photo editing software. This seamless integration simplifies workflow, allowing users to easily incorporate enhanced images into larger creative projects.
The evolution of user interfaces and customization options within these tools highlight a broader trend of enhancing user experience while embracing the fascinating intersection of art, nostalgia, and technological innovation. As these tools mature, they are likely to become even more powerful, versatile, and user-friendly, further blurring the lines between past and present visual styles.
Exploring the AI PS2 Filter How Machine Learning Recreates Early 2000s Gaming Aesthetics - Impact on Digital Art and Retro Gaming Communities
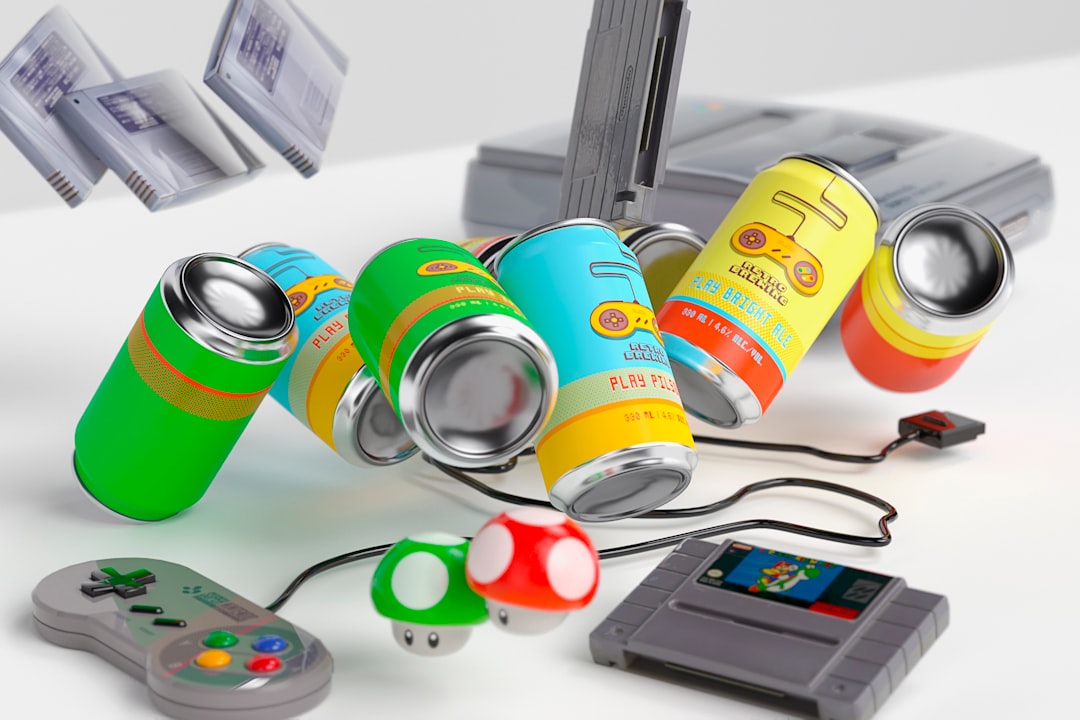
The rise of AI filters capable of mimicking the visual style of PlayStation 2 games, like the PS2 Filter, has had a noticeable impact on both digital art and the retro gaming communities. These filters provide individuals with a simple way to apply the nostalgic aesthetics of early 2000s gaming to their own images, inspiring creative exploration and a renewed appreciation for the charm of simpler graphics. The ability to effortlessly achieve a vintage look empowers a wider audience to participate in digital art, potentially bringing about new forms of creative expression within these communities. Beyond the creative aspect, the filters reignite interest in classic gaming titles by offering users a way to revisit the unique visuals of their favorite games from the past. The tools themselves, with their customization features and intuitive interfaces, allow for extensive experimentation and tweaking, pushing the boundaries of creativity while celebrating the distinctive characteristics of retro game aesthetics. However, this increased accessibility and focus on nostalgia also presents a challenge: striking a balance between simplifying the process for broader use and ensuring the results stay true to the intricacies of the PS2 era's specific visual style. It's a question of whether the ease of use can sometimes lead to a less authentic recreation of the style and visuals that defined those games.
The integration of machine learning into digital art tools has opened up new avenues for image reconstruction and restoration. These AI-powered systems can effectively fill in missing details or recover degraded parts of images by learning from vast datasets. This ability is especially relevant in preserving older digital art and reclaiming potentially lost pieces of gaming history.
Within retro gaming communities, this technology has sparked a renewed interest in classic titles. The capacity to upscale and enhance the visuals of older games has not only revitalized discussions but also fostered new creative endeavors, potentially breathing new life into titles that might have faded from the spotlight. The ability to remaster and bring these experiences to modern platforms invites a new generation of gamers to explore and appreciate game design from a different era.
The filters also offer a fascinating capability to apply retro aesthetics to modern content, allowing artists to blend older styles with new artistic directions. This encourages experimentation and pushes creative boundaries within the gaming community, fostering a hybrid aesthetic that draws from both modern and retro inspirations.
Furthermore, AI-driven image enhancement tools are adept at capturing and reproducing the nuanced visual characteristics of past gaming eras. Tools like the PS2 filter meticulously learn from massive datasets of PS2 game visuals, including unique color palettes and dithering techniques, to achieve remarkably authentic recreations. This focus on stylistic detail not only provides users with impressive visuals but also cultivates a stronger appreciation for the clever workarounds and artistic limitations of early game developers.
The precision of these adaptive upscaling techniques surpasses traditional methods, often producing images with superior detail and sharpness. This is particularly noticeable in the reproduction of retro game graphics, which often feature sharp edges and distinct textures that traditional upscaling techniques struggle to accurately represent. The ability to maintain those unique characteristics makes AI-based filters appealing for anyone seeking a faithful recreation of classic games’ distinct visual styles.
The accessibility of these AI-driven tools has significantly boosted the vibrancy of online communities dedicated to retro gaming and digital art. User-generated content flourishes as people share their remastered creations, and online discussions spark new collaborations to refine styles and techniques. This collaborative environment helps to fuel a shared passion for the aesthetics of gaming's past and helps define those visual characteristics as a community.
The enhanced capabilities of watermark removal techniques raise complex ethical issues for these online spaces. As these AI tools grow increasingly sophisticated, questions around the ownership and rights of original content creators emerge when modified works are distributed. This issue is prominent as fan-made content becomes intertwined with commercially distributed media.
The concept of historical accuracy is also a common debate amongst retro enthusiasts. While the AI filters can produce stunning results, purists argue that certain stylistic choices need to be preserved for the resulting images to accurately represent the genuine experiences of the original games. Striking a balance between modern technical capabilities and historical faithfulness is an ongoing point of discussion.
The development of these image processing tools has also sparked a reassessment of archival practices amongst retro artists. They can now revisit their older work and, with the help of these powerful enhancements, update or improve their creations. This renewed interest in the past can be seen as an exchange between past and present artistic expressions. The ease of refinement or the ability to modify can create a nuanced interpretation of an original creator's vision.
The ongoing development and refinement of AI-powered image filters are directly shaped by continuous feedback from users. This collaborative approach helps refine the technology, ensuring that it resonates with the aesthetic preferences of the community it serves. Features that are deemed successful through user input will often drive future enhancements, blurring the line between technological innovation and user experience.
Exploring the AI PS2 Filter How Machine Learning Recreates Early 2000s Gaming Aesthetics - Comparison with Other Retro Gaming Filters
When comparing the AI PS2 Filter to other retro gaming filters, we see a clear distinction in how effectively they recreate the nostalgic visuals of older gaming. While many filters simply upscale images, the PS2 Filter leverages advanced AI, specifically convolutional neural networks, to recreate the specific textures and visual oddities of PS2 graphics. This approach produces results that better capture the core aesthetic of low-resolution games, avoiding many of the artifacts that plague other approaches. However, this same reliance on AI introduces some questions. For instance, the ease of use might lead some users to simply tweak settings without understanding the nuances of PS2-era styles, ultimately resulting in less authentic-looking images. Despite this, the continuous development of filters like these embodies a wider movement – a fascinating combination of technological innovation with the wish to keep the unique charm of older gaming styles alive.
### Comparison with Other Retro Gaming Filters
The AI PS2 Filter stands out from other retro gaming filters in a few key ways, largely due to its foundation in machine learning. Firstly, it goes beyond simple pixelation effects and instead employs detailed machine learning processes to reconstruct fine image features lost in original graphics. This enables a more accurate representation of textures and the visual style of the PS2 era. Traditional filters tend to have a more generic approach, relying on fixed parameters which can limit the range of achievable styles. The PS2 filter, however, learns from a comprehensive database of PS2 game textures and visual characteristics, which allows it to capture the unique qualities of specific games more precisely.
Another difference is the AI PS2 filter's ability to accurately emulate dithering, a technique used in PS2-era games to simulate a richer color range and depth. Basic filters often struggle to replicate the subtleties of dithering, leading to less convincing visuals. The PS2 Filter, however, uses its trained algorithms to understand and mimic these patterns, which are a signature visual feature of the era.
Beyond static visuals, the PS2 filter incorporates temporal smoothing, something often overlooked in other filters. This means it can account for motion artifacts in videos and animations, making enhanced gameplay appear fluid and authentic rather than jerky. Many upscale filters struggle to handle diverse input resolutions effectively, but this filter can adjust its processes based on the input resolution to optimize its performance without sacrificing the retro aesthetic. It's an elegant solution that sets it apart from filters that offer more static processing.
However, the increasing sophistication of AI image filters raises some complex ethical considerations. Specifically, its capability for watermark removal poses questions about altering and sharing existing content, a challenge less prominent with simpler filters. Another distinct characteristic is the active participation of the community in refining the PS2 Filter. It includes mechanisms for user feedback and preferences, leading to ongoing improvements in the filter. This approach is quite rare in image processing tools, where development is typically more isolated.
While the filter aims to achieve nostalgic looks, it also focuses on maintaining image quality and clarity via sophisticated algorithms, a welcome compromise between a retro feel and preserving detail. Yet, a key limitation stems from the reliance on the training dataset—if the dataset lacks representation of specific game styles or visual approaches, the filter's performance might vary significantly, possibly leading to a disconnect with the intended aesthetic of the source material.
Lastly, unlike many conventional filters that apply modifications uniformly, the PS2 Filter offers layered editing, allowing users to customize specific parts of the enhancement process. This level of customization allows for a more fine-grained artistic approach compared to tools that offer only limited modifications. The combination of nuanced control and retro-style recreation showcases a promising development direction for AI-powered image filters and its role in preserving and extending gaming's visual history.
Exploring the AI PS2 Filter How Machine Learning Recreates Early 2000s Gaming Aesthetics - Future Developments in AI-Powered Nostalgia Filters
The future trajectory of AI-powered nostalgia filters holds a mix of promise and challenge. As these filters incorporate more advanced learning techniques and respond to user feedback, they could become increasingly adept at blending retro styles with modern artistic approaches. This might lead to a fascinating fusion of old and new aesthetics, allowing users to create images that resonate with both past and present artistic expressions.
However, there's a potential drawback to this evolving technology. As it becomes more user-friendly, it may also risk oversimplifying the complex visual characteristics of vintage graphics styles. If users don't grasp the subtle details of past gaming aesthetics, the resulting images might not accurately capture the spirit of those eras, ultimately detracting from the authentic experience.
This journey of technological advancement, intertwined with human creativity and memory, will likely redefine our interaction with digital art and the way we revisit and reimagine cherished gaming memories. Further down the line, the ethical implications of AI-driven image generation, particularly issues of ownership and original content, will likely become more prominent within the digital art and gaming communities. The balance between technological innovation and the preservation of authentic vintage styles will become increasingly crucial in these evolving landscapes.
AI-powered nostalgia filters are becoming increasingly sophisticated in their ability to recreate the visual styles of past gaming eras. These filters, often relying on convolutional neural networks, go beyond simple upscaling by learning from vast datasets of vintage game graphics. This allows them to reconstruct textures and color dynamics with impressive accuracy, capturing the distinctive pixelation and limited color palettes that defined games like those on the PS2. For instance, they've become quite adept at recognizing and replicating the dithering techniques employed back then, which simulated depth and richness within the confines of those earlier hardware limitations. This makes a significant difference in achieving a genuinely nostalgic look, something that less sophisticated filters often struggle to replicate.
Another fascinating aspect is the implementation of temporal smoothing in filters like the PS2 Filter. It helps create smoother animations and gameplay that aligns with the original experience of those games, addressing a common issue in simple retro filters that often lead to jerky or unnatural-looking movement in upscaled video. Furthermore, these filters are becoming more adaptable, intelligently adjusting their computational load based on the image resolution. This allows for real-time enhancements without significant performance bottlenecks, a feature often missing in less refined tools.
However, this level of sophistication also introduces ethical considerations. The ability of some filters to remove watermarks effectively raises concerns about content ownership and the potential for misuse. It's a stark contrast to simpler filtering techniques which don't alter the original image to such a degree. Moreover, the development of these AI filters is increasingly driven by user feedback, creating a unique relationship between developers and users, distinct from the more traditional software development approach. This feedback helps developers refine their algorithms based on community preferences, leading to continuous improvements over time.
The impact of these filters on digital art and the gaming community is noteworthy. They make it significantly easier to experiment with retro aesthetics, allowing for a broader range of creative expression. This can redefine styles and introduce new forms of digital art. But, we also see a trade-off: The greater the ease of use, the greater the potential for overlooking the intricacies of specific styles, leading to results that might not perfectly capture the authenticity of a PS2-era game.
The quality of these filters' output ultimately depends heavily on the training data. Filters trained on a limited dataset may not be able to handle all styles effectively, leading to inconsistent results. Thus, the breadth and depth of the training dataset are crucial in ensuring that the filter successfully recreates a specific era's aesthetics.
Beyond creating visually impressive retro images, these AI filters are helping to preserve the visual heritage of gaming. By meticulously reconstructing the unique characteristics of PS2 graphics, they ensure that this style and the creative limitations that led to its distinctive look don't fade into obscurity, making this visual history available for future generations of gamers and artists. They help solidify a link between past and present, where the ingenuity and artistry of those earlier eras is maintained for future appreciation.
More Posts from kahma.io: