Create incredible AI portraits and headshots of yourself, your loved ones, dead relatives (or really anyone) in stunning 8K quality. (Get started for free)
The Real Cost of AI Background Removal Tools in 2024 A Price-Performance Analysis
The Real Cost of AI Background Removal Tools in 2024 A Price-Performance Analysis - Cost Analysis 2024 Market Prices From $03 to $29 Per Image
The landscape of AI background removal tools in 2024 presents a diverse range of pricing, with costs per image varying from a mere $0.03 to as much as $29. This wide spectrum reflects the varying levels of sophistication and capabilities offered by different tools, a testament to the burgeoning field and the competitive drive within it. While budget-conscious options are certainly available, more advanced tools catering to professional applications, like AI-powered headshot creation or high-quality portrait photography, typically come with a higher price tag. It's essential to recognize that the cost of developing and maintaining AI capabilities is increasing, driven by factors like more complex training data requirements and rising infrastructure expenses. This inflationary trend in AI development impacts the tools we see in the market. Therefore, the actual value of these tools rests heavily on how well they fulfill individual needs and contribute to desired outcomes. Businesses and individuals must carefully evaluate their specific requirements and weigh them against the potential cost savings or aesthetic improvements offered by AI-driven solutions.
The pricing landscape for AI-powered image manipulation, specifically in the realm of portrait photography and headshot creation, shows a wide range. Prices for services like AI background removal fluctuate wildly, starting as low as three cents per image and going up to twenty-nine dollars. This variance likely reflects the differing levels of sophistication and features that each tool offers.
It's intriguing that some AI-based solutions can be found at extremely low price points, but it's important to consider what you're giving up. These cheaper options often sacrifice output quality, with lower resolution being a common trade-off. In environments like professional headshots, where detail is paramount, such limitations might not be acceptable. This leads to a question: what is the trade-off for the lower price?
There's clearly a massive potential for AI to transform the world of photography, especially in terms of increasing productivity and reducing the labor involved in edits like background removal. However, it's important to keep in mind that AI's ability to truly understand and replicate the intricacies of human expression and aesthetic sensibilities is still developing. Subtleties like color gradients and emotional nuances can be challenging for algorithms to fully grasp. Some researchers are finding that the color range and color accuracy that the human eye can see is difficult for some of these AI systems to create, at least in a natural looking way.
The development and implementation of these AI tools is also not cheap. Training the underlying algorithms on massive amounts of data requires significant infrastructure and computational resources, and this expense is likely reflected in the higher-end tools. Companies like Netflix, as an example, are pouring enormous sums into developing AI technologies, highlighting both the potential rewards and the significant costs involved in creating robust AI solutions. While the cost of AI development is coming down thanks to more affordable training methods, the training costs continue to escalate. There's also the cost of ongoing development and upgrades that need to be accounted for as the technology progresses. This raises the question of how these rising costs are impacting the pricing of image manipulation services.
The entire field is in flux, though, with a growing market and increasing demands for more advanced AI algorithms and deep learning models driving growth. There's still a critical discussion to be had on the best way to use AI in the context of portrait photography and how to get the most out of these technologies. It's a complex ecosystem of development, engineering, pricing, and user needs. Finding the best option for a specific purpose requires careful consideration of the costs and benefits to ensure that one's investment is truly optimized.
The Real Cost of AI Background Removal Tools in 2024 A Price-Performance Analysis - Processing Speed Face Off 32 Images Per Minute vs Manual Editing
When it comes to image editing, particularly in areas like portrait or headshot photography, the speed at which images can be processed is becoming increasingly important. AI-powered tools have emerged as a game-changer, capable of handling tasks like background removal at incredible speeds, potentially processing up to 32 images within a single minute. This rapid turnaround is a major benefit in situations where quick results are crucial.
However, the swiftness of AI solutions comes with a trade-off. While AI can quickly automate repetitive tasks, it often falls short when it comes to the more subtle and artistic aspects of image editing. The ability to make fine adjustments, to create a unique artistic style, or to handle complex edits requiring a nuanced understanding of a photograph often relies more on the human touch of manual editing. This distinction becomes critical when the goal is to create high-quality images, especially in fields like professional headshots where detail and artistry are paramount.
The decision of whether to lean on the speed of AI or the artistic control of manual editing often boils down to the specific needs and priorities of the project. Factors such as turnaround time, desired level of quality, and budget all influence the optimal choice. As the landscape of photo editing continues to evolve with the advancements of AI, it's essential to be aware of the strengths and weaknesses of each approach to make informed choices and achieve the best results.
AI-powered image editing tools have revolutionized the speed at which images can be processed, achieving rates of up to 32 images per minute. This is a significant leap compared to the pace of manual editing, which might only allow for 3 to 5 images per hour. The efficiency gains are undeniably substantial, especially for tasks like background removal or basic photo enhancement. This rapid pace is made possible by AI algorithms that analyze and modify images swiftly.
However, while AI tools excel at speed, they still have limitations when it comes to artistic nuance. Human editors, with their years of experience and understanding of composition and light, can often create more compelling and expressive portraits. AI, while rapidly improving in facial recognition (with some systems boasting over 98% accuracy), sometimes struggles with subtleties like skin tones and achieving a natural look. This can be particularly noticeable in professional headshots where capturing a realistic and flattering representation is paramount.
Interestingly, the cost of AI image editing can be quite variable. While some tools offer low introductory prices, for instance, a few cents per image, those seeking higher resolution or advanced features can see those costs quickly climb due to subscription models and added charges for improved outputs. This can be a bit of a surprise for users initially lured by low costs.
Another intriguing aspect is how AI systems are trained. Many rely on massive databases of images – some upwards of 10 million – to develop their capabilities. This training process is computationally demanding and resource-intensive. It's also worth noting that the development of these AI tools is not a stagnant endeavor. Constantly improving them demands more and more processing power, and significant investments are being made by companies and research teams to optimize these systems.
Ultimately, while AI is undeniably transforming image editing, especially in productivity, there's a nuanced discussion to be had regarding the appropriate use of these tools. Some photographers opt for a hybrid approach, utilizing AI for quick edits and then leveraging their expertise for the finer details. The decision of whether to primarily use AI or manual methods hinges on individual needs and the desired outcome, be it speed, cost optimization, or aesthetic control. And while the efficiency of AI tools is appealing, many users express a preference for human editors, especially when it comes to the crucial aspects of personal branding and capturing unique expressions in a portrait or headshot. This highlights the ongoing conversation around trust and the specific skills that contribute to meaningful visual communication. The reliance on AI is certainly an evolving landscape in the field of photography.
The Real Cost of AI Background Removal Tools in 2024 A Price-Performance Analysis - Quality Check Edge Detection and Hair Strand Analysis
Within the realm of AI-powered image editing, particularly for headshots and portrait photography, the scrutiny of image quality has become increasingly vital. A key element of this quality assessment is the ability to accurately detect and analyze hair strands, a nuanced aspect of image processing that AI is still learning to master.
The precision of edge detection is paramount in ensuring a clean separation between the subject and the background, especially in contexts where a flawless headshot or professional portrait is desired. Newer edge detection algorithms, built upon deep learning principles, show promise in achieving this, capable of discerning subtle variations in hair textures and patterns. This level of detail becomes increasingly important as the demand for high-quality output from AI tools grows.
However, the ability to accurately capture the complexities of hair – from intricate braids to delicate strands – is still a challenge for AI. While algorithms are improving, there's a recognition that replicating the nuance and artistic quality of a human eye and hand in photography remains a significant hurdle. Therefore, the evaluation of these AI systems includes a growing focus on how well they perform in these specific areas. The ability to analyze the edge detection performance, with specific focus on hair strands, has become a key part of determining a tool's ability to provide a quality result. This growing need for quality metrics is indicative of the field's continuing development, balancing the desire for fast and efficient image editing with the high standards demanded by professional applications in fields like portrait and headshot photography.
Examining the quality of AI background removal tools, particularly in the context of headshots and portrait photography, reveals a fascinating interplay between edge detection and hair strand analysis. Edge detection algorithms, while often employing techniques like Sobel and Canny, can introduce inconsistencies in results, sometimes creating undesirable artifacts that detract from the natural appearance of an image. This can be especially problematic for achieving the level of realism and detail needed in professional headshots.
AI faces significant hurdles when it comes to accurately analyzing individual hair strands. The intricacies of hair, including flyaways and varying textures, often prove too challenging for current algorithms. As a result, tools frequently misinterpret hair boundaries, leading to unrealistic and undesirable edits. This becomes a significant issue when maintaining authenticity and natural-looking hair is paramount in a portrait or headshot.
Further complicating matters is the challenge of color accuracy. While the human eye is capable of distinguishing over a million colors, some AI-powered background removal tools struggle to reproduce the subtle nuances of skin tones and hair colors. This can manifest as unnatural-looking skin or overly processed hair, diminishing the intended emotional expression in a portrait.
The resolution of the input image plays a significant role in the quality of the AI's output. High-resolution images offer greater detail for the algorithms to analyze, leading to clearer definition of hair and more precise edge detection. Conversely, lower-resolution images result in a loss of fine detail, making it harder for the AI to effectively analyze hair strands and edges, leading to more errors.
The complexity of neural networks used for these tasks can be another constraint. More sophisticated neural networks, often trained on datasets as large as 100 million images, can dramatically improve the precision of edge detection. However, these models demand extensive computational power and training time, which can be a significant barrier to entry for smaller operations.
Despite promises of rapid processing, real-time performance can be limited by the intricate algorithms required for hair and edge detection. In demanding professional environments, where fast turnaround times are crucial, this can lead to slower-than-desired outputs, potentially creating a bottleneck in the workflow.
Integrating sophisticated quality checks for edge detection can introduce bottlenecks into the workflow. If AI systems are designed to prioritize high-quality outputs, they may require additional processing time to perform those assessments, potentially negating the speed advantage of automation.
Interestingly, the price-to-performance ratio of these tools is closely linked to the quality of hair analysis. Higher-quality tools often command significantly higher prices due to the need for sophisticated algorithms and expansive training datasets. This can create a barrier to entry for smaller businesses who may prioritize affordability over the most advanced features.
AI platforms exhibit variability in how effectively they perform edge detection and hair analysis. This has resulted in inconsistencies in user experiences, where unexpected discrepancies in outcomes have been reported. Consequently, choosing the right platform becomes more critical, emphasizing the need to carefully evaluate a specific platform's suitability for intended use rather than simply selecting the cheapest option.
Despite the remarkable progress in AI technology, it seems that the human touch continues to be indispensable in the final editing stages of portrait photography. Human artists excel at adapting styles and making creative decisions that enhance the artistic quality of the image. This makes AI tools more of a complement than a replacement for human artists, highlighting the critical role of human skills in achieving truly exceptional results. This interplay between AI and human expertise reflects the ongoing evolution of photography in an increasingly AI-driven world.
The Real Cost of AI Background Removal Tools in 2024 A Price-Performance Analysis - Monthly Subscription Models Free Tier vs Pay As You Go
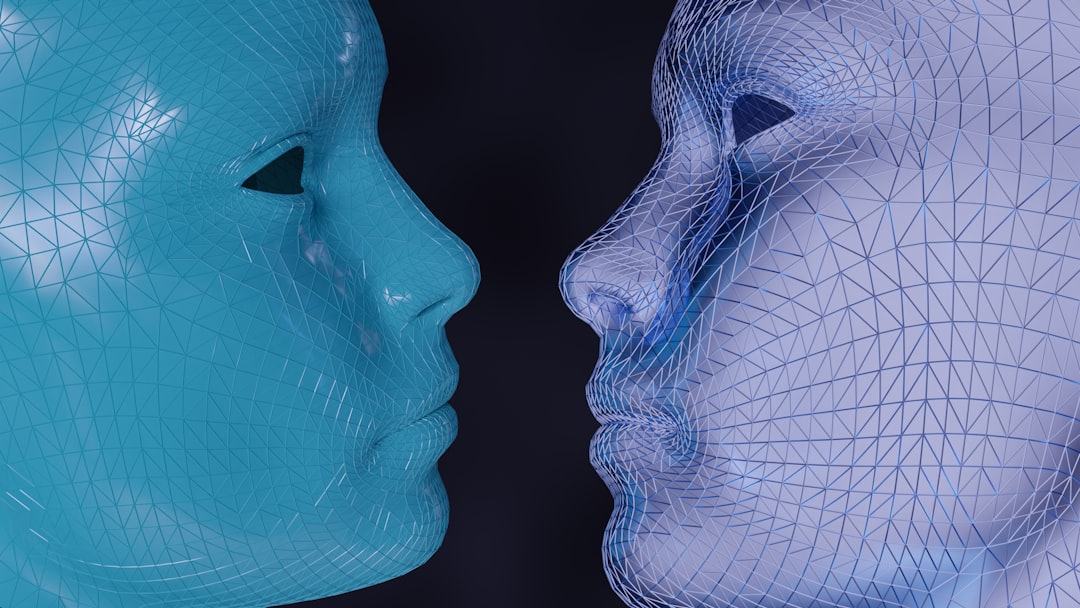
AI-powered background removal tools are increasingly adopting two primary subscription models: free tiers and pay-as-you-go. Free tiers serve as a gateway, offering initial access to the technology without upfront costs. However, this often comes with restrictions that might impact the quality or processing speed of results, making them unsuitable for professionals needing consistently high-quality output in fields like portrait or headshot photography. On the other hand, pay-as-you-go plans provide users with the flexibility to pay only for the services they utilize, appealing to those with unpredictable editing needs. But this approach can lead to budgeting uncertainties, particularly when the goal is to obtain high-quality results, especially in professional applications like headshots where image quality and details are crucial. The selection between these pricing structures ultimately boils down to a user's specific requirements, their approach to cost management, and their need for consistent quality and processing speed in a fast-paced market. This delicate balance of pricing and performance is key to finding the right tool for specific photography purposes.
When exploring the pricing structures of AI-powered portrait and headshot tools, we find a common duality: monthly subscription models often include free tiers, while others use a "pay-as-you-go" system. Understanding these different approaches is key, as they impact both the user and the tool developer.
Initial observations show that users often start with free trials but then shift towards pay-as-you-go options when their needs become more intricate, particularly for tasks like professional headshot creation or detailed portrait editing. This suggests a desire for flexibility and the ability to control spending based on specific project needs.
However, subscriptions, while seemingly attractive, can often lead to higher total costs, especially if your needs are sporadic. For example, businesses occasionally needing high-quality headshots may find pay-as-you-go to be more budget-friendly than a subscription. This highlights a crucial distinction: what seems inexpensive upfront might not be the most cost-effective choice in the long run.
Interestingly, users who choose pay-as-you-go models tend to have higher expectations for image quality. This likely stems from the perception that they're paying for each result and thus demand high value for their money. This increased quality pressure incentivizes AI tool developers to continuously improve their algorithms, ultimately leading to a more competitive market for users.
The allure of free tiers can be deceptive. While a free tier might grant access to basic features, the truly useful functionalities like high-resolution outputs or advanced editing capabilities are usually behind a paywall. This can create a false impression of the tool's actual capabilities.
We also observe a correlation between pricing model and the quality of the underlying AI system. Pay-as-you-go options generally prioritize precision and accuracy due to greater investments in large training datasets, something often lacking in the limited datasets used for free tier models.
Additionally, customer support plays a role in user decisions. Subscription services typically offer more extensive support, particularly helpful for those grappling with intricate edits. Conversely, pay-as-you-go models might offer limited or no support, making them less desirable for those with complex tasks or little prior experience.
User behavior is also shaped by psychology. Studies in behavioral economics reveal that subscription fees are often viewed as "sunk costs," meaning users are less inclined to switch services even if a better, pay-as-you-go option emerges later. This can lock users into a subscription, which may or may not be the most efficient option.
Professionals in photography often prioritize subscription plans that promise regular updates and improvements over the lower upfront cost of a pay-as-you-go approach. This suggests a preference for long-term quality and support, hinting that the perception of consistent value over time is gaining prominence.
Subscription models frequently offer performance guarantees, something often absent in the pay-as-you-go world. This can translate into peace of mind for users, particularly important for those working on critical projects where speed and quality are non-negotiable.
It's essential to acknowledge that the costs associated with AI development and operation are substantial. Subscription fees often contribute to supporting this continuous innovation, including research and ongoing improvement. Users opting for pay-as-you-go models may see less frequent updates or limited support as a result.
Ultimately, understanding these different pricing models requires considering one's individual needs and preferences. There is no single “best” model, as the trade-offs between cost, flexibility, and features vary significantly. Choosing wisely involves carefully evaluating the potential benefits and limitations of each option to ensure the tools chosen truly support individual creative goals.
The Real Cost of AI Background Removal Tools in 2024 A Price-Performance Analysis - Resource Usage Memory Load and CPU Requirements
The increasing sophistication of AI background removal tools in 2024 has brought the topic of resource usage and computational requirements to the forefront, especially for applications like AI-powered headshots and portrait photography. The push to make these tools accessible on a wider range of devices has led to innovations like model quantization, a technique that can reduce memory consumption and improve performance on CPUs. However, this optimization comes with a caveat: a decrease in memory needs can sometimes result in slower processing times or a decline in image quality. In professional contexts, where high-quality images are paramount, these trade-offs can be problematic.
Beyond the technical aspects, the financial implications of deploying these AI tools have also become more prominent. Managing complex projects and maintaining AI infrastructure can necessitate a significant human resource investment, and the costs involved can often exceed initial estimates. Striking a balance between leveraging the power of AI for advanced image editing and managing the associated costs is a challenge many face. The resulting impact on both the costs of running these tools and the ultimate creative output of the photographer is substantial. It’s a balancing act that every user needs to be mindful of as they assess the trade-offs involved when embracing this evolving technology. Ultimately, photographers who want to leverage AI need to carefully consider how these resource needs can affect their workflow and creative vision.
The resource usage of AI background removal tools varies significantly, depending on the complexity of the underlying models. For instance, sophisticated tools might need 16 GB of RAM or more to operate effectively, while simpler options could manage with as little as 2 GB. This difference underscores the connection between a tool's capability and its resource requirements.
Some of the more complex algorithms used for background removal require high CPU performance, particularly those capable of processing data in parallel across multiple cores. This can result in impressively fast processing times, potentially just a few seconds per image for intricate edits. In contrast, older or simpler approaches might require several minutes per high-resolution image, highlighting a substantial gap in speed based on the algorithm's sophistication.
The intricacy of the algorithms themselves has a direct influence on the processing load. For example, convolutional neural networks can dramatically increase the amount of CPU cycles needed, with some models using over 100% more CPU time compared to less complex methods. This reflects the continual tension between the desire for better output quality and the need to maintain efficient performance.
The resolution of the images being processed greatly impacts the performance of these AI tools. Not only do higher-resolution images offer more detail for the algorithms to analyze, but they also require a much larger amount of memory and processing power. This means that even minor increases in resolution can significantly slow down a system that isn't well equipped.
We often find that faster processing comes at the cost of a decrease in output quality, especially when handling intricate tasks like hair strand detection. A tool might boast that it can process 32 images per minute, but the precision of edge detection might suffer, leading to noticeable artifacts that necessitate manual cleanup. This reminds us of the ongoing trade-off between speed and accuracy in these systems.
Many AI tools are now leveraging GPU acceleration to significantly decrease rendering times, achieving processing speeds that previously required a substantial amount of CPU resources. This illustrates a shift towards increased dependence on parallel processing capabilities for improved performance.
In situations where real-time editing is essential – for instance, in live digital photo sessions – the processing load can easily strain system performance. AI algorithms that are designed for batch processing may struggle to provide immediate feedback during portrait photography shoots, making them less suitable for such real-time interactions.
There's notable inconsistency in the performance of AI tools. Benchmarks often showcase excellent results under optimal conditions, but real-world usage frequently exposes limitations depending on the user's hardware and the image's complexity. This creates a challenge for users trying to select the right tool for their needs.
Newer resource management techniques, such as dynamic resource allocation and load balancing, are gaining importance. As these AI tools evolve, there’s a growing emphasis on the ability to intelligently distribute processing power based on current workloads. This could help reduce lag times in situations with high demands.
The growing popularity of cloud-based AI services implies a continual cost for memory and CPU associated with the underlying infrastructure, ultimately influencing pricing models. These infrastructure costs are a critical aspect of how these services are priced for end-users, especially when considering the difference between subscription models and pay-as-you-go models.
The Real Cost of AI Background Removal Tools in 2024 A Price-Performance Analysis - Bulk Processing Options With Different File Formats
The ability to process images in bulk using AI background removal tools has become increasingly important, especially for tasks like large-scale headshot or portrait photography projects. These tools now support a broader range of file formats, including common options like JPG, PNG, and WEBP, making it easier to handle a wide variety of image libraries. The promise of rapid processing for large numbers of images is appealing, but it's worth noting that the actual speed can vary, particularly when dealing with complex images. It seems that achieving the ideal balance between fast processing and high-quality output, particularly when focusing on details like hair or fine edges, is still a challenge. Users must weigh the need for speed against the potential impact on image quality and resource use. These tools, while capable, may introduce bottlenecks or demand substantial computational power, which can significantly affect processing speed and pricing. This brings into focus the trade-offs users have to consider in finding the right balance between efficiency, cost, and desired outcomes. As this field of image editing matures, careful consideration of these factors will be crucial for any photographer hoping to leverage these technologies for their unique projects.
The capabilities of AI background removal tools are increasingly tied to the specific image file formats they handle. Formats like TIFF, due to their ability to preserve finer details, can lead to better results compared to JPEGs when processed by AI algorithms. This difference in detail preservation affects the overall quality of the output.
Interestingly, the choice of file format can also influence processing speed. For instance, PNG files, though lossless, tend to take longer to process than lossy formats like JPEGs due to their larger file sizes. This can significantly impact the speed of bulk image processing operations.
The color depth of a format also plays a role in how accurately AI tools can render details. A standard JPEG has a color depth of 24 bits, while newer formats like JPEG 2000 can support a wider range. This broader color palette can be critical for AI tools to capture the intricate details in elements like hair in portraits.
Furthermore, the way a file format compresses data can significantly impact the accuracy of the background removal process. Lossy formats like JPEGs discard some data during compression, making it challenging for the AI to accurately detect edges. This can lead to less-than-ideal results in professional settings where perfect edges are critical.
AI systems generally perform better with higher-resolution images, and formats like RAW excel at maintaining this higher resolution. Using lower-resolution formats like web-optimized JPEGs can cause the loss of important data needed for background removal. This loss of information is directly reflected in the quality of the resulting headshots.
Some formats, like RAW, include significant metadata, which contains information about the camera and lighting conditions when the image was taken. This additional information can prove very helpful to AI algorithms, allowing them to better understand the image and perform background removal more effectively.
When dealing with large batches of images in different formats, a mixture of formats can slow down the overall processing speed. This is because the AI tools need to switch between different algorithms to handle each unique format. This highlights the importance of having consistent file types in workflows to maintain optimal processing speed.
It’s also worth noting that some AI tools are specifically designed for particular file formats. Using rarer or less common formats like HEIC can lead to compatibility issues and increased processing times, impacting overall production efficiency.
The choice of format can even affect real-time editing experiences. Formats that demand high processing power, due to their greater detail and fidelity, can lead to noticeable delays during live photo sessions, potentially disrupting the flow of portrait shoots.
Finally, there’s the inherent trade-off between quality and cost. Users who need the highest-quality outputs tend to opt for more sophisticated file formats, but this choice can significantly increase the costs of AI processing. This trade-off requires careful consideration when deciding between quality and budget restrictions.
It appears that the field of AI background removal is still very much in development, with a need for users to understand the implications of the file format they choose. It's a fascinating area of research and it's likely the sophistication of algorithms and their ability to handle various formats will continue to improve over time.
Create incredible AI portraits and headshots of yourself, your loved ones, dead relatives (or really anyone) in stunning 8K quality. (Get started for free)
More Posts from kahma.io: